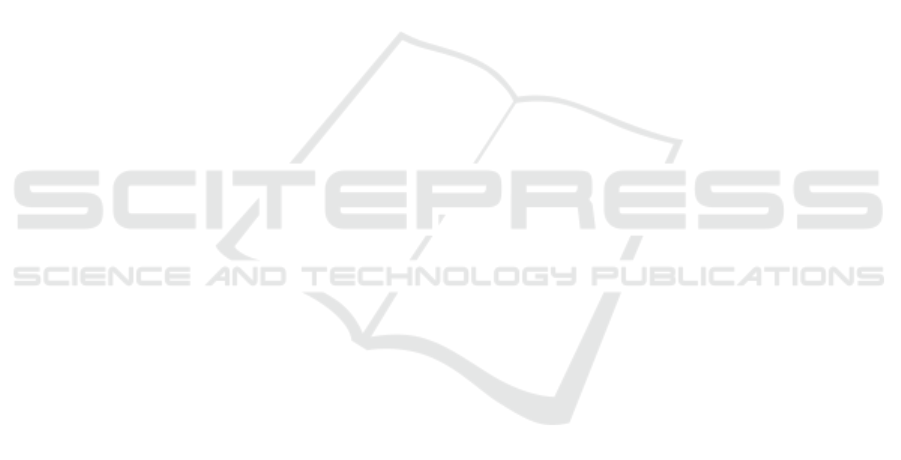
5 CONCLUSIONS
In this study, we introduce a GCN based model that is
capable of grading the heterogeneous prostate cancer
slides with GS 7 automatically. We construct prostate
cancer slides as graphs to model correlations among
patches and capture topological information of the
whole slides. By combining DIFFPOOL layer with
GCN layers, our method achieves an classification
accuracy of 79.5%, which is superior to state-of-the-
art result on the dataset of TCGA. The reported re-
sults demonstrate efficiency of the proposed method,
which are consistent with our expectation.
ACKNOWLEDGEMENTS
This work was supported in part by the National Key
Research and Development Program of China un-
der Grant 2018YFC0910500, in part by the National
Natural Science Foundation of China under Grant
61906032, in part by the Liaoning Key R&D Program
under Grant 2019JH2/10100030, in part by the Liaon-
ing United Foundation under Grant U1908214, and in
part by the Fundamental Research Funds for the Cen-
tral Universities under Grant DUT20RC(4)005 and
DUT18RC(3)069.
REFERENCES
Abhishek, Vahadane, Tingying, Peng, Amit, Sethi, Shadi,
Albarqouni, Lichao, and and, W. (2016). Structure-
preserving color normalization and sparse stain sepa-
ration for histological images. IEEE transactions on
medical imaging.
Arvaniti, E., Fricker, K. S., Moret, M., Rupp, N. J., Her-
manns, T., Fankhauser, C. D., Wey, N., Wild, P. J.,
Ruschoff, J. H., and Claassen, M. (2018). Automated
gleason grading of prostate cancer tissue microarrays
via deep learning. Scientific Reports, 8(1):12054–
12054.
Bulten, W., Pinckaers, H., Van Boven, H., Vink, R., De Bel,
T., Van Ginneken, B., Jeroen, V. D. L., De Kaa, H. V.,
and Litjens, G. (2019). Automated gleason grading of
prostate biopsies using deep learning.
Chang, H., Loss, L. A., and Parvin, B. (2011). Nuclear seg-
mentation in h & e sections via multi-reference graph
cut ( mrgc ).
del Toro, O. J., Atzori, M., Ot
´
alora, S., Andersson, M.,
Eur
´
en, K., Hedlund, M., R
¨
onnquist, P., and M
¨
uller,
H. (2017). Convolutional neural networks for an au-
tomatic classification of prostate tissue slides with
high-grade Gleason score. In Gurcan, M. N. and
Tomaszewski, J. E., editors, Medical Imaging 2017:
Digital Pathology, volume 10140, pages 165 – 173.
International Society for Optics and Photonics, SPIE.
Epstein and Jonathan, I. (2018). Prostate cancer grading:
a decade after the 2005 modified system. Modern
Pathology An Official Journal of the United States &
Canadian Academy of Pathology Inc, 31:S47.
Epstein, J. I., Egevad, L., Amin, M. B., Delahunt, B.,
Srigley, J. R., and Humphrey, P. A. (2015). The 2014
international society of urological pathology (isup)
consensus conference on gleason grading of prostatic
carcinoma definition of grading patterns and proposal
for a new grading system. The American Journal of
Surgical Pathology, 40(2):244–252.
Epstein, J. I., Zelefsky, M. J., Sjoberg, D. D., Nelson, J. B.,
Egevad, L., Magigalluzzi, C., Vickers, A. J., Parwani,
A. V., Reuter, V. E., Fine, S. W., et al. (2016). A
contemporary prostate cancer grading system: A vali-
dated alternative to the gleason score. European Urol-
ogy, 69(3):428–435.
Gilmer, J., Schoenholz, S. S., Riley, P. F., Vinyals, O., and
Dahl, G. E. (2017). Neural message passing for quan-
tum chemistry.
He, K., Zhang, X., Ren, S., and Sun, J. (2016). Deep resid-
ual learning for image recognition.
Hochreiter, S. and Schmidhuber, J. (1997). Long short-term
memory. Neural Computation, 9(8):1735–1780.
Huang, G., Liu, Z., Laurens, V. D. M., and Weinberger,
K. Q. (2016). Densely connected convolutional net-
works.
Jian, Ren, Kubra, Karagoz, Michael, Gatza, David, J,
Foran, and and, X. (2018). Differentiation among
prostate cancer patients with gleason score of 7 us-
ing histopathology whole-slide image and genomic
data. Proceedings of SPIE–the International Society
for Optical Engineering.
Kallen, H., Molin, J., Heyden, A., Lundstrom, C., and As-
trom, K. (2016). Towards grading gleason score us-
ing generically trained deep convolutional neural net-
works. pages 1163–1167.
Khurd, P., Bahlmann, C., Maday, P., Kamen, A., Gibb-
sstrauss, S. L., Genega, E. M., and Frangioni, J. V.
(2010). Computer-aided gleason grading of prostate
cancer histopathological images using texton forests.
pages 636–639.
Li, J., Li, W., Gertych, A., Knudsen, B. S., Speier, W.,
and Arnold, C. W. (2019). An attention-based multi-
resolution model for prostate whole slide imageclassi-
fication and localization. arXiv: Computer Vision and
Pattern Recognition.
Moch, H., Cubilla, A. L., Humphrey, P. A., Reuter, V. E.,
and Ulbright, T. M. (2016). The 2016 who classifica-
tion of tumours of the urinary system and male genital
organs—part a: Renal, penile, and testicular tumours.
European Urology, 70(1):93–105.
Nagpal, K., Foote, D., Liu, Y., Pohsuan, Chen, Wulczyn, E.,
Tan, F., Olson, N., Smith, J. L., Mohtashamian, A.,
et al. (2018). Development and validation of a deep
learning algorithm for improving gleason scoring of
prostate cancer. arXiv: Computer Vision and Pattern
Recognition.
Otsu, N. (2007). A threshold selection method from gray-
level histograms. IEEE Transactions on Systems Man
& Cybernetics, 9(1):62–66.
Weakly Supervised Gleason Grading of Prostate Cancer Slides using Graph Neural Network
433