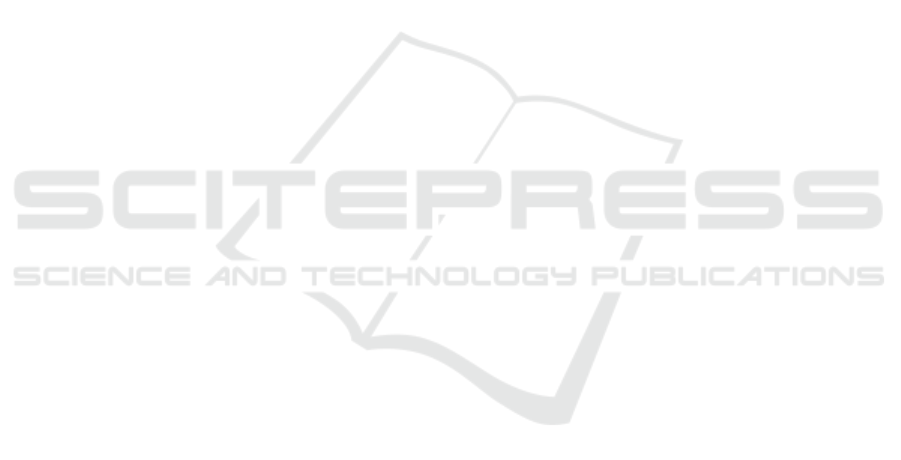
Human Language Technologies, Volume 1 (Long and
Short Papers), pages 4171–4186.
Dinan, E., Humeau, S., Chintagunta, B., and Weston, J.
(2019). Build it break it fix it for dialogue safety:
Robustness from adversarial human attack. In Inui,
K., Jiang, J., Ng, V., and Wan, X., editors, Proceed-
ings of the 2019 Conference on Empirical Methods
in Natural Language Processing and the 9th Inter-
national Joint Conference on Natural Language Pro-
cessing, EMNLP-IJCNLP 2019, Hong Kong, China,
November 3-7, 2019, pages 4536–4545. Association
for Computational Linguistics.
Dixon, L., Li, J., Sorensen, J., Thain, N., and Vasserman,
L. (2018). Measuring and mitigating unintended bias
in text classification. In Proceedings of the 2018
AAAI/ACM Conference on AI, Ethics, and Society,
AIES ’18, page 67–73, New York, NY, USA. Asso-
ciation for Computing Machinery.
Friedler, S. A., Scheidegger, C., Venkatasubramanian, S.,
Choudhary, S., Hamilton, E. P., and Roth, D. (2019).
A comparative study of fairness-enhancing interven-
tions in machine learning. In Proceedings of the con-
ference on fairness, accountability, and transparency,
pages 329–338.
Google (2019). Unintended bias in toxicity classifi-
cation, https://www.kaggle.com/c/jigsaw-unintended-
bias-in-toxicity-classification.
Kleinberg, J. M., Mullainathan, S., and Raghavan, M.
(2016). Inherent trade-offs in the fair determination
of risk scores. CoRR, abs/1609.05807.
Kumar, R., Ojha, A. K., Malmasi, S., and Zampieri, M.
(2018). Benchmarking aggression identification in
social media. In Proceedings of the First Workshop
on Trolling, Aggression and Cyberbullying (TRAC-
2018), pages 1–11, Santa Fe, New Mexico, USA. As-
sociation for Computational Linguistics.
Liu, X., He, P., Chen, W., and Gao, J. (2019). Multi-task
deep neural networks for natural language understand-
ing. In Proceedings of the 57th Annual Meeting of
the Association for Computational Linguistics, pages
4487–4496.
Loshchilov, I. and Hutter, F. (2018). Decoupled weight
decay regularization. In International Conference on
Learning Representations.
McCann, B., Keskar, N. S., Xiong, C., and Socher, R.
(2018). The natural language decathlon: Multi-
task learning as question answering. arXiv preprint
arXiv:1806.08730.
Menon, A. K. and Williamson, R. C. (2018). The cost of
fairness in binary classification. volume 81 of Pro-
ceedings of Machine Learning Research, pages 107–
118, New York, NY, USA. PMLR.
Mishra, P., Tredici, M. D., Yannakoudakis, H., and Shutova,
E. (2019). Author profiling for hate speech detection.
CoRR, abs/1902.06734.
Park, J. H., Shin, J., and Fung, P. (2018). Reducing gender
bias in abusive language detection. In Proceedings of
the 2018 Conference on Empirical Methods in Natu-
ral Language Processing, pages 2799–2804, Brussels,
Belgium. Association for Computational Linguistics.
Raffel, C., Shazeer, N., Roberts, A., Lee, K., Narang, S.,
Matena, M., Zhou, Y., Li, W., and Liu, P. J. (2019).
Exploring the limits of transfer learning with a unified
text-to-text transformer. arXiv e-prints.
Sun, C., Qiu, X., Xu, Y., and Huang, X. (2019). How
to fine-tune bert for text classification? In Sun,
M., Huang, X., Ji, H., Liu, Z., and Liu, Y., editors,
Chinese Computational Linguistics, pages 194–206,
Cham. Springer International Publishing.
Suresh, H., Gong, J. J., and Guttag, J. V. (2018). Learn-
ing tasks for multitask learning: Heterogenous patient
populations in the icu. In Proceedings of the 24th
ACM SIGKDD International Conference on Knowl-
edge Discovery & Data Mining, pages 802–810.
ACM.
Thomas, D., Dana, W., Michael, M., and Ingmar, W. (2017).
Automated hate speech detection and the problem of
offensive language. In Proceedings of the 11th Inter-
national AAAI Conference on Web and Social Media.
ICWSM.
Waseem, Z. (2016). Are you a racist or am I seeing things?
annotator influence on hate speech detection on Twit-
ter. In Proceedings of the First Workshop on NLP
and Computational Social Science, pages 138–142,
Austin, Texas. Association for Computational Lin-
guistics.
Wiegand, M., Ruppenhofer, J., and Kleinbauer, T. (2019).
Detection of Abusive Language: the Problem of Bi-
ased Datasets. In Proceedings of the 2019 Conference
of the North American Chapter of the Association for
Computational Linguistics: Human Language Tech-
nologies, Volume 1 (Long and Short Papers), pages
602–608, Minneapolis, Minnesota. Association for
Computational Linguistics.
Wulczyn, E., Thain, N., and Dixon, L. (2017). Ex machina:
Personal attacks seen at scale. In Proceedings of the
26th International Conference on World Wide Web,
WWW ’17, page 1391–1399, Republic and Canton of
Geneva, CHE. International World Wide Web Confer-
ences Steering Committee.
Zhu, Y., Kiros, R., Zemel, R., Salakhutdinov, R., Urtasun,
R., Torralba, A., and Fidler, S. (2015). Aligning books
and movies: Towards story-like visual explanations by
watching movies and reading books. In The IEEE In-
ternational Conference on Computer Vision (ICCV).
ICAART 2021 - 13th International Conference on Agents and Artificial Intelligence
940