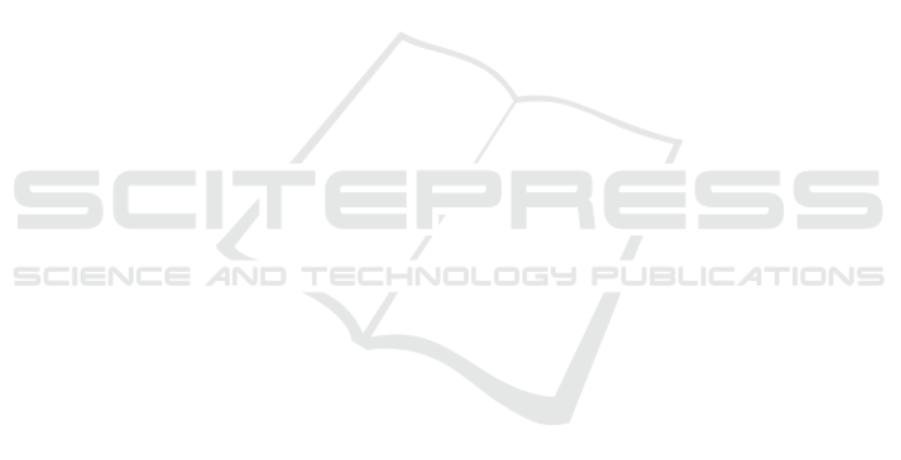
Kannan, A., Kurach, K., Ravi, S., Kaufmann, T., Tomkins,
A., Miklos, B., Corrado, G., Lukacs, L., Ganea, M.,
Young, P., et al. (2016). Smart reply: Automated
response suggestion for email. In Proceedings of
the 22nd ACM SIGKDD International Conference on
Knowledge Discovery and Data Mining, pages 955–
964. ACM.
McClosky, D., Charniak, E., and Johnson, M. (2006). Ef-
fective self-training for parsing. In Proceedings of the
main conference on human language technology con-
ference of the North American Chapter of the Asso-
ciation of Computational Linguistics, pages 152–159.
Association for Computational Linguistics.
Miyato, T., Dai, A. M., and Goodfellow, I. (2016). Adver-
sarial training methods for semi-supervised text clas-
sification. arXiv preprint arXiv:1605.07725.
Pavlinek, M. and Podgorelec, V. (2017). Text classification
method based on self-training and lda topic models.
Expert Systems with Applications, 80:83–93.
Pedregosa, F., Varoquaux, G., Gramfort, A., Michel, V.,
Thirion, B., Grisel, O., Blondel, M., Prettenhofer,
P., Weiss, R., Dubourg, V., Vanderplas, J., Passos,
A., Cournapeau, D., Brucher, M., Perrot, M., and
Duchesnay, E. (2011). Scikit-learn: Machine learning
in Python. Journal of Machine Learning Research,
12:2825–2830.
Perez, L. and Wang, J. (2017). The effectiveness of data
augmentation in image classification using deep learn-
ing. arXiv preprint arXiv:1712.04621.
Savelka, J., Trivedi, G., and Ashley, K. D. (2015). Apply-
ing an interactive machine learning approach to statu-
tory analysis. In Proceedings of the 28th Annual Con-
ference on Legal Knowledge and Information Systems
(JURIX’15). IOS Press.
Sawant, S. S. and Prabukumar, M. (2018). A review
on graph-based semi-supervised learning methods for
hyperspectral image classification. The Egyptian
Journal of Remote Sensing and Space Science.
Sun, X. and He, J. (2018). A novel approach to generate a
large scale of supervised data for short text sentiment
analysis. Multimedia Tools and Applications, pages
1–21.
Waltl, B., Bonczek, G., Scepankova, E., and Matthes, F.
(2019). Semantic types of legal norms in german laws:
classification and analysis using local linear explana-
tions. Artificial Intelligence and Law, 27(1):43–71.
Waltl, B., Muhr, J., Glaser, I., Bonczek, G., Scepankova,
E., and Matthes, F. (2017). Classifying legal norms
with active machine learning. In Proceedings of the
28th Annual Conference on Legal Knowledge and In-
formation Systems (JURIX’15), pages 11–20.
Wang, W. Y. and Yang, D. (2015). That’s so annoying!!!:
A lexical and frame-semantic embedding based data
augmentation approach to automatic categorization of
annoying behaviors using# petpeeve tweets. In Pro-
ceedings of the 2015 Conference on Empirical Meth-
ods in Natural Language Processing, pages 2557–
2563.
Wei, J. and Zou, K. (2019). Eda: Easy data augmentation
techniques for boosting performance on text classifi-
cation tasks. arXiv preprint arXiv:1901.11196.
Wiegand, M., Siegel, M., and Ruppenhofer, J. (2018).
Overview of the germeval 2018 shared task on the
identification of offensive language. In 14th Con-
ference on Natural Language Processing KONVENS
2018.
Yang, Y. and Shafiq, M. O. (2018). Large scale and par-
allel sentiment analysis based on label propagation in
twitter data. In 2018 17th IEEE International Con-
ference On Trust, Security And Privacy In Computing
And Communications/12th IEEE International Con-
ference On Big Data Science And Engineering (Trust-
Com/BigDataSE), pages 1791–1798. IEEE.
Zhang, X. and LeCun, Y. (2015). Text understanding from
scratch. arXiv preprint arXiv:1502.01710.
Zhou, D., Bousquet, O., Lal, T. N., Weston, J., and
Sch
¨
olkopf, B. (2004). Learning with local and global
consistency. In Advances in neural information pro-
cessing systems, pages 321–328.
Zhu, X., Ghahramani, Z., and Lafferty, J. D. (2003). Semi-
supervised learning using gaussian fields and har-
monic functions. In Proceedings of the 20th Inter-
national conference on Machine learning (ICML-03),
pages 912–919.
Zhu, X. and Goldberg, A. B. (2009). Introduction to semi-
supervised learning. Synthesis lectures on artificial
intelligence and machine learning, 3(1):1–130.
Zhu, X. J. (2005). Semi-supervised learning literature
survey. Technical report, University of Wisconsin-
Madison Department of Computer Sciences.
ICPRAM 2021 - 10th International Conference on Pattern Recognition Applications and Methods
564