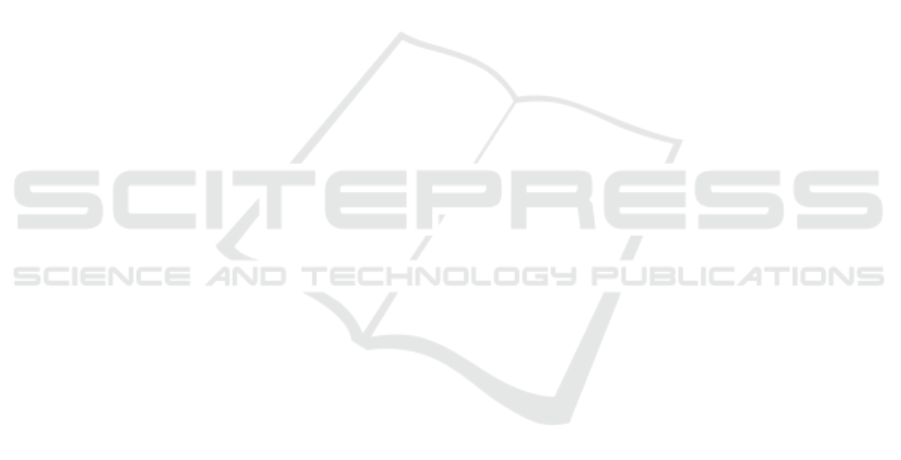
mation can thus easily compensate the performance
which was degraded by audio noise in ASR. In con-
trast, AV-DCCA simultaneously used audio and vi-
sual features projected into the same DCCA space.
Since visual features have less information than clean
audio features, it is considered that the contribution
of visual DCCA features is limited in the AV-DCCA
model. Finally, we also evaluated V-DCCA in which
a model was trained using only visual DCCA features.
We then found that its recognition performance is al-
most the same as ASR.
5.3.2 Experiment B
Fig 5 depicts recognition accuracy using the small
training data set. Note that the recognition accuracy
of lipreading is 65.00%. Our proposed models A-
DCCA and AV-DCCA achieved better performance
than ASR and E2E-MM, similar to Experiment A.
On the contrary, this time AV-DCCA is superior to
A-DCCA in all the SNRs. We also checked the re-
sults when using V-DCCA, and found AV-DCCA is
better. Because the training set included only a few
auditory clean data, the A-DCCA recognition model
might suffer from the lack of reliable training data,
so might V-DCCA. By jointly utilizing visual DCCA
features, it is found that AV-DCCA was able to learn
the model significantly, resulting the performance im-
provement.
6 CONCLUSION
In this paper, we proposed to utilize DCCA tech-
niques for speech recognition, to improve its per-
formance in noisy environments. Because DCCA
tries to enhance the correlation between audio and
visual modalities, we investigate how we can utilize
this architecture for ASR. Compared to conventional
audio-only ASR and early-fusion-based audio-visual
ASR, our DCCA approaches basically achieved better
recognition performance. In the first experiment, in
the case we have enough training data, DCCA can be
used to enhance noisy audio features resulting higher
recognition accuracy. We carried out the second ex-
periment and found that, if only a few training data
are available, using not only audio DCCA but also
visual DCCA features is a better strategy as data aug-
mentation. Consequently, we found the effectiveness
of applying DCCA with visual data to audio-only or
audio-visual ASR.
As future works, we will test Connectionist Tem-
poral Classification (CTC) for utterance-level speech
recognition. Expansion of employing the DCCA ar-
chitecture to the other tasks, not limited to audio-only
or audio-visual speech recognition, is also attractive.
REFERENCES
A.Graves, Mohamed, A.-R., and G.Hinton (2013). Speech
recognition with deep recurrent neural networks. In
Proc. ICASSP2013.
A.Renduchintala, S.Ding, M.Wiesner, and S.Watanabe
(2018). Multi-modal data augmentation for end-to-
end asr. In arXiv preprint arXiv:1803.10299v3.
G.Andrew, R.Arora, J.Bilmes, and K.Livescu (2013). Deep
canonical correlation analysis. In Proc. ICML2013,
pages 1247–1255.
G.Paraskevopoulos, S.Parthasarathy, A.Khare, and
S.Sundaram (2020). Multiresolution and multimodal
speech recognition with transformers. In arXiv
preprint arXiv:2004.14840v1.
H.I.Fawaz, G.Forestier, J.Weber, L.Idoumghar, and
P.A.Muller (2018). Data augmentation using synthetic
data for time series classification with deep residual
networks. In arXiv preprint arXiv:1810.02455.
Joze, H. R. V., Shaban, A., Iuzzolino, M. L., and Koishida,
K. (2020). Mmtm:multimodal transfer module for cnn
fusion. In Proc. of CVPR.
J.Thiemann and N.Ito, E. (2013). Demand: a collection of
multichannel recordings of acoustic noise in diverse
environments. In Proc. ICA, page 035081–035081.
K.Noda, Y.Yamaguchi, K.Nakadai, H.G.Okuno, and
T.Ogata (2015). ”audiovisual speech recognition us-
ing deep learning. In Applied Intelligence, 42(4),
pages 722–737.
M.Shimonishi, S.Tamura, and S.Hayamizu (2019). Mul-
timodal feature conversion for visual speech recog-
nition using deep canonical correlation analysis. In
Proc. NCSP2019.
P.Zhou, W.Yang, W.Chen, Y.Wang, and J.Jia (2019).
Modality attention for end-to-end audio-visual speech
recognition. In arXiv preprint arXiv:1811.05250v2.
R.Arora and K.Livescu (2012). Kernel cca for multi-view
learning of acoustic features using articulatory mea-
surements. In Proc. MLSLP2012.
S.Tamura, C.Miyajima, N.Kitaoka, T.Yamada, S.Tsuge,
T.Takiguchi, K.Yamamoto, T.Nishiura, M.Nakayama,
Y.Denda, M.Fujimoto, S.Matsuda, T.Ogawa,
S.Kuroiwa, K.Takeda, and S.Nakamura (2010).
Censrec-1-av: An audio-visual corpus for noisy
bimodal speech recog- nition. In Proc. AVSP2010,
pages 85–88.
T.DeVries and G.W.Taylor (2017). Improved regularization
of convolutional neural networks with cutout. In arXiv
preprint arXiv:1708.04552.
T.Makino, H.Liao, Y.Assael, B.Shillingford, B.Garcia,
O.Braga, and O.Siohan (2019). Recurrent neural net-
work transducer for audio-visual speech recognition.
In arXiv preprint arXiv:1911.04890v1.
Speech Recognition using Deep Canonical Correlation Analysis in Noisy Environments
69