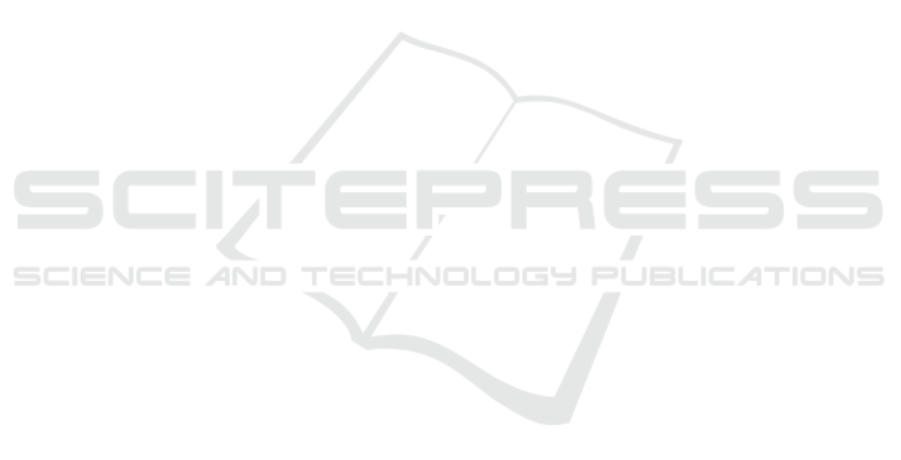
classification of hyperspectral remote sensing images.
Artech House.
Boumeddane, S., Hamdad, L., Dabo-Niang, S., and Had-
dadou, H. (2019). Spatial kernel discriminant anal-
ysis: Applied for hyperspectral image classification.
In Proceedings of the 11th International Conference
on Agents and Artificial Intelligence, ICAART 2019,
Volume 2, Prague, Czech Republic, February 19-21,
2019., pages 184–191.
Boumeddane, S., Hamdad, L., Haddadou, H., and Dabo-
Niang, S. (2020). A kernel discriminant analysis for
spatially dependent data. Distributed and Parallel
Databases, pages 1–24.
Chen, Y.-C. (2017). A tutorial on kernel density estimation
and recent advances. Biostatistics & Epidemiology,
1(1):161–187.
Dabo-Niang, S., Hamdad, L., Ternynck, C., and Yao, A.-
F. (2014). A kernel spatial density estimation allow-
ing for the analysis of spatial clustering. application
to monsoon asia drought atlas data. Stochastic envi-
ronmental research and risk assessment, 28(8):2075–
2099.
Ghamisi, P., Benediktsson, J. A., Cavallaro, G., and Plaza,
A. (2014). Automatic framework for spectral-spatial
classification based on supervised feature extraction
and morphological attribute profiles. IEEE J. Sel. Top.
Appl. Earth Obs. Remote. Sens., 7(6):2147–2160.
Ghosh, A. K. and Chaudhuri, P. (2004). Optimal smooth-
ing in kernel discriminant analysis. Statistica Sinica,
pages 457–483.
Gramacki, A. (2018). Nonparametric kernel density esti-
mation and its computational aspects. Springer.
Hang, R., Liu, Q., Hong, D., and Ghamisi, P. (2019). Cas-
caded recurrent neural networks for hyperspectral im-
age classification. IEEE Trans. Geoscience and Re-
mote Sensing, 57(8):5384–5394.
Li, J., Cheng, K., Wang, S., Morstatter, F., Trevino, R. P.,
Tang, J., and Liu, H. (2018). Feature selection: A data
perspective. ACM Comput. Surv., 50(6):94:1–94:45.
Miller, H. J. (2004). Tobler’s first law and spatial analysis.
Annals of the Association of American Geographers,
94(2):284–289.
Mou, L., Ghamisi, P., and Zhu, X. X. (2017). Deep recur-
rent neural networks for hyperspectral image classifi-
cation. IEEE Trans. Geoscience and Remote Sensing,
55(7):3639–3655.
Mou, L., Ghamisi, P., and Zhu, X. X. (2018). Un-
supervised spectral-spatial feature learning via deep
residual conv-deconv network for hyperspectral image
classification. IEEE Trans. Geoscience and Remote
Sensing, 56(1):391–406.
Pu, H., Chen, Z., Wang, B., and Jiang, G.-M. (2014). A
novel spatial–spectral similarity measure for dimen-
sionality reduction and classification of hyperspectral
imagery. IEEE Transactions on Geoscience and Re-
mote Sensing, 52(11):7008–7022.
Sheikhpour, R., Sarram, M. A., Chahooki, M. A. Z., and
Sheikhpour, R. (2017). A kernelized non-parametric
classifier based on feature ranking in anisotropic gaus-
sian kernel. Neurocomputing, 267:545–555.
Sheikhpour, R., Sarram, M. A., and Sheikhpour, R. (2016).
Particle swarm optimization for bandwidth determina-
tion and feature selection of kernel density estimation
based classifiers in diagnosis of breast cancer. Appl.
Soft Comput., 40:113–131.
Silverman, B. W. (1986). Density estimation for statistics
and data analysis, volume 26. CRC press.
van der Walt, C. M. and Barnard, E. (2017). Variable
kernel density estimation in high-dimensional feature
spaces. In Proceedings of the Thirty-First AAAI Con-
ference on Artificial Intelligence, February 4-9, 2017,
San Francisco, California, USA., pages 2674–2680.
Wang, D., Tan, D., and Liu, L. (2018). Particle swarm op-
timization algorithm: an overview. Soft Computing,
22(2):387–408.
Zaman, F., Wong, Y., and Ng, B. (2016). Density-based
denoising of point cloud. CoRR, abs/1602.05312.
Zhou, Z., Si, G., Zhang, Y., and Zheng, K. (2018). Robust
clustering by identifying the veins of clusters based on
kernel density estimation. Knowledge-Based Systems,
159:309–320.
Dimensionality Reduction and Bandwidth Selection for Spatial Kernel Discriminant Analysis
285