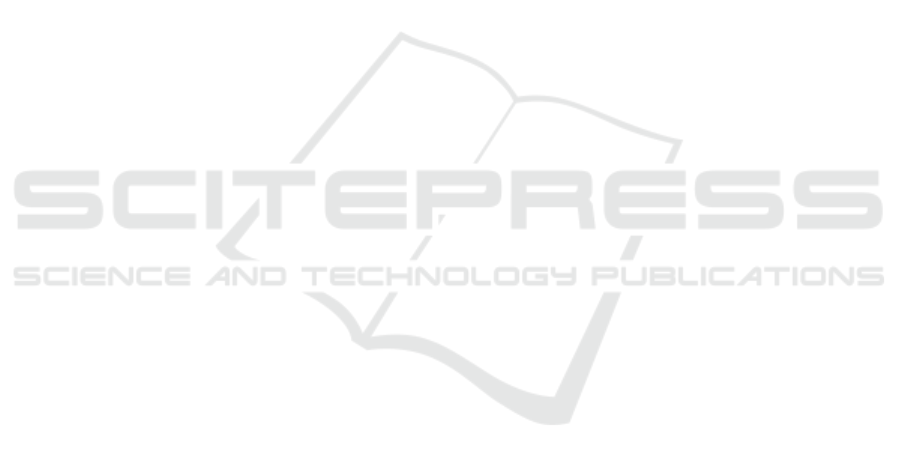
and intensity of physical activity using an accelerom-
eter. Medicine & Science in Sports & Exercise,
41(9):1770–1777.
Bulling, A., Blanke, U., and Schiele, B. (2014). A tuto-
rial on human activity recognition using body-worn
inertial sensors. ACM Computing Surveys (CSUR),
46(3):1–33.
Butterworth, S. (1930). On the theory of filter amplifiers. In
Wireless Engineer (also called Experimental Wireless
and the Wireless Engineer).
Chen, K., Zhang, D., Yao, L., Guo, B., Yu, Z., and Liu, Y.
(2020). Deep learning for sensor-based human activ-
ity recognition: Overview, challenges and opportuni-
ties. arXiv preprint arXiv:2001.07416.
Chen, Y., Wang, J., Huang, M., and Yu, H. (2019). Cross-
position activity recognition with stratified transfer
learning. Pervasive and Mobile Computing, 57:1–13.
Dehghani, A., Sarbishei, O., Glatard, T., and Shihab, E.
(2019). A quantitative comparison of overlapping
and non-overlapping sliding windows for human ac-
tivity recognition using inertial sensors. Sensors,
19(22):5026.
Figueira, C., Matias, R., and Gamboa, H. (2016). Body lo-
cation independent activity monitoring. In Bahr, A.,
Abu Saleh, L., Schr
¨
oder, D., and Krautschneider, W.,
editors, Integrated 16-Channel Neural Recording Cir-
cuit with SPI Interface and Error Correction Code in
130 nm CMOS Technology, pages 190–197, Hamburg
and Set
´
ubal. Technische Universit
¨
at Hamburg Uni-
versit
¨
atsbibliothek and SCITEPRESS - Science and
Technology Publications Lda.
Foerster, F., Smeja, M., and Fahrenberg, J. (1999). Detec-
tion of posture and motion by accelerometry: A vali-
dation study in ambulatory monitoring. Computers in
Human Behavior, 15(5):571–583.
Gao, X., Luo, H., Wang, Q., Zhao, F., Ye, L., and Zhang, Y.
(2019). A human activity recognition algorithm based
on stacking denoising autoencoder and lightgbm. Sen-
sors (Basel, Switzerland), 19(4).
Ghosh, A. and Riccardi, G. (2014). Recognizing human
activities from smartphone sensor signals. In Hua,
K. A., editor, Proceedings of the 2014 ACM Confer-
ence on Multimedia, November 3 - 7, 2014, Orlando,
FL, USA, pages 865–868, New York, NY. ACM.
Huynh, D. T. G. (2008). Human Activity Recognition with
Wearable Sensors. PhD thesis, Technische Universit
¨
at
Darmstadt.
Ignatov, A. (2018). Real-time human activity recognition
from accelerometer data using convolutional neural
networks. Applied Soft Computing, 62:915–922.
Janidarmian, M., Roshan Fekr, A., Radecka, K., and Zilic,
Z. (2017). A comprehensive analysis on wearable ac-
celeration sensors in human activity recognition. Sen-
sors (Basel, Switzerland), 17(3).
Khan, A. M., Lee, Y.-K., Lee, S. Y., and Kim, T.-
S. (2010). A triaxial accelerometer-based physical-
activity recognition via augmented-signal features and
a hierarchical recognizer. IEEE transactions on infor-
mation technology in biomedicine : a publication of
the IEEE Engineering in Medicine and Biology Soci-
ety, 14(5):1166–1172.
Klieme, E., Tietz, C., and Meinel, C. (2018). Beware of
smombies: Verification of users based on activities
while walking. In 2018 17th IEEE International Con-
ference On Trust, Security And Privacy In Computing
And Communications/12th IEEE International Con-
ference On Big Data Science And Engineering (Trust-
Com/BigDataSE), pages 651–660. IEEE.
Krishnan, N. C. and Panchanathan, S. (2008). Analysis of
low resolution accelerometer data for continuous hu-
man activity recognition. In 2008 IEEE International
Conference on Acoustics, Speech and Signal Process-
ing, pages 3337–3340. IEEE.
Mannini, A. and Sabatini, A. M. (2010). Machine learning
methods for classifying human physical activity from
on-body accelerometers. Sensors, 10(2):1154–1175.
Mannini, A., Sabatini, A. M., and Intille, S. S. (2015).
Accelerometry-based recognition of the placement
sites of a wearable sensor. Pervasive and Mobile Com-
puting, 21:62–74.
Mantyjarvi, J., Himberg, J., and Seppanen, T. (2001). Rec-
ognizing human motion with multiple acceleration
sensors. In 2001 IEEE International Conference on
Systems, Man and Cybernetics. e-Systems and e-Man
for Cybernetics in Cyberspace (Cat. No. 01CH37236),
volume 2, pages 747–752. IEEE.
Mohamed, R., Zainudin, M. N. S., Sulaiman, M. N., Peru-
mal, T., and Mustapha, N. (2018). Multi-label classi-
fication for physical activity recognition from various
accelerometer sensor positions. Journal of Informa-
tion and Communication Technology, 17(2):209–231.
Orha, I. and Oniga, S. (2014). Study regarding the opti-
mal sensors placement on the body for human activity
recognition. In 2014 IEEE 20th International Sympo-
sium for Design and Technology in Electronic Pack-
aging (SIITME), pages 203–206. IEEE.
Rickham, P. P. (1964). Human experimentation. code of
ethics of the world medical association. declaration of
helsinki. British medical journal, 2(5402):177.
Rivera, P., Valerezo, E., Choi, M.-T., and Kim, T.-S. (2017).
Recognition of human hand activities based on a sin-
gle wrist imu using recurrent neural networks. Inter-
national Journal of Pharma Medicine and Biological
Sciences, 6(4).
Shoaib, M., Scholten, H., and Havinga, P. (2013). To-
wards physical activity recognition using smartphone
sensors. In Ubiquitous Intelligence and Computing,
2013 IEEE 10th International Conference on and 10th
International Conference on Autonomic and Trusted
Computing (UIC/ATC), pages 80–87. IEEE.
Su, X., Tong, H., and Ji, P. (2014). Activity recognition
with smartphone sensors. Tsinghua Science and Tech-
nology, 19(3):235–249.
Tapia, E. M., Intille, S. S., Haskell, W., Larson, K., Wright,
J., King, A., and Friedman, R. (2007). Real-time
recognition of physical activities and their intensities
using wireless accelerometers and a heart rate moni-
tor. In 2007 11th IEEE international symposium on
wearable computers, pages 37–40. IEEE.
Optimal Sensor Placement for Human Activity Recognition with a Minimal Smartphone–IMU Setup
47