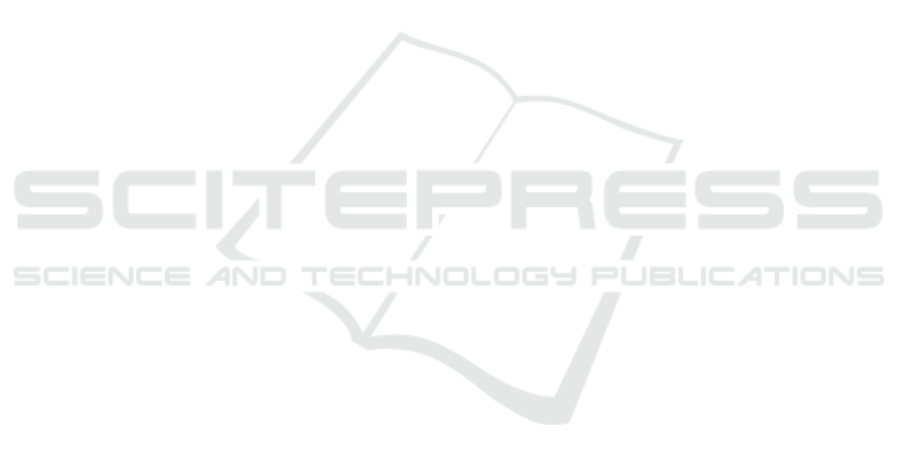
Madhu, P., Kosti, R., M
¨
uhrenberg, L., Bell, P., Maier, A.,
and Christlein, V. (2019). Recognizing characters in
art history using deep learning. In Proceedings of
the 1st Workshop on Structuring and Understanding
of Multimedia heritAge Contents, pages 15–22.
Mager, T., Khademi, S., Siebes, R., Hein, C., de Boer,
V., and van Gemert, J. (2020). Visual Content Anal-
ysis and Linked Data for Automatic Enrichment of
Architecture-Related Images. In Kremers, H., editor,
Digital Cultural Heritage, pages 279–293. Springer
International Publishing, Cham.
Maiwald, F. (2019). Generation of a benchmark dataset us-
ing historical photographs for an automated evaluation
of different feature matching methods. International
Archives of the Photogrammetry, Remote Sensing &
Spatial Information Sciences.
Maiwald, F., Vietze, T., Schneider, D., Henze, F., M
¨
unster,
S., and Niebling, F. (2017). Photogrammetric analysis
of historical image repositories for virtual reconstruc-
tion in the field of digital humanities. The Interna-
tional Archives of Photogrammetry, Remote Sensing
and Spatial Information Sciences, 42:447.
Niebling, F., Bruschke, J., Messemer, H., Wacker, M., and
von Mammen, S. (2020). Analyzing Spatial Distri-
bution of Photographs in Cultural Heritage Applica-
tions. In Liarokapis, F., Voulodimos, A., Doulamis,
N., and Doulamis, A., editors, Visual Computing for
Cultural Heritage, Springer Series on Cultural Com-
puting, pages 391–408. Springer International Pub-
lishing, Cham.
Offert, F. (2018). Images of image machines. visual in-
terpretability in computer vision for art. In Proceed-
ings of the European Conference on Computer Vision
(ECCV).
Oliva, A. and Torralba, A. (2001). Modeling the shape
of the scene: A holistic representation of the spatial
envelope. International journal of computer vision,
42(3):145–175.
Palermo, F., Hays, J., and Efros, A. A. (2012). Dating his-
torical color images. In Fitzgibbon, A., Lazebnik, S.,
Perona, P., Sato, Y., and Schmid, C., editors, Com-
puter Vision – ECCV 2012, pages 499–512, Berlin,
Heidelberg. Springer Berlin Heidelberg.
Petras, V., Hill, T., Stiller, J., and G
¨
ade, M. (2017).
Europeana–a search engine for digitised cultural her-
itage material. Datenbank-Spektrum, 17(1):41–46.
Rawat, W. and Wang, Z. (2017). Deep Convolutional Neu-
ral Networks for Image Classification: A Comprehen-
sive Review. Neural Computation, 29(9):2352–2449.
Smith, L. N. (2018). A disciplined approach to neu-
ral network hyper-parameters: Part 1 – learning
rate, batch size, momentum, and weight decay.
arXiv:1803.09820 [cs, stat].
Szegedy, C., Liu, W., Jia, Y., Sermanet, P., Reed, S.,
Anguelov, D., Erhan, D., Vanhoucke, V., and Rabi-
novich, A. (2014). Going Deeper with Convolutions.
arXiv:1409.4842 [cs].
Xiao, J., Hays, J., Ehinger, K. A., Oliva, A., and Torralba,
A. (2010). Sun database: Large-scale scene recog-
nition from abbey to zoo. In 2010 IEEE Computer
Society Conference on Computer Vision and Pattern
Recognition, pages 3485–3492. IEEE.
Xie, L., Lee, F., Liu, L., Kotani, K., and Chen, Q. (2020).
Scene recognition: A comprehensive survey. Pattern
Recognition, 102:107205.
Yun, S., Han, D., Oh, S. J., Chun, S., Choe, J., and
Yoo, Y. (2019). CutMix: Regularization Strategy
to Train Strong Classifiers with Localizable Features.
arXiv:1905.04899 [cs].
Zhang, H., Cisse, M., Dauphin, Y. N., and Lopez-Paz, D.
(2018). Mixup: Beyond Empirical Risk Minimiza-
tion. arXiv:1710.09412 [cs, stat].
Zhou, B., Lapedriza, A., Khosla, A., Oliva, A., and Tor-
ralba, A. (2018). Places: A 10 Million Image
Database for Scene Recognition. IEEE Transac-
tions on Pattern Analysis and Machine Intelligence,
40(6):1452–1464.
APPENDIX
Here we list our annotation guides lines per cate-
gories. Some of these categories have been not been
included in the training, because they included fewer
than twenty images.
Accident Car. Traffic accident involving an automo-
bile
Accident Stretcher. Accident involving an person on
a stretcher
Accident Train. Traffic accident involving an train
Aerial. Picture with an aerial perspective
Amphitheater. The collection contains many pic-
tures taken at an outside amphitheater in Bloemen-
daal.
Animals cow. Pictures of live and dead cows.
Animals dog. Pictures of dogs
Animals horse. Pictures of horses
Animals misc. Animals that do not fit in the previous
categories. For final dataset, this might be subdivided
in more categories.
Artwork. Artwork without people, the focus is on the
art work. There is also a separate category for statues.
Auditorium. Public building (used for speeches, per-
formances) where audience sits. Pictures with and
without audiences. Overlap with categories ‘confer-
ence room‘ and ‘speech’
Bakery. Photos inside bakery, baking bread, present-
ing bread indoor/outdoors Overlap with Kitchen
Bar. Area where drinks are served/consumed. Over-
lap with Dining Room/Game Room
Baseball. People playing baseball
Basketball. People playing basketball.
Beach. If the beach is the picture’s main focus, we se-
lect beach. Dunes is a separate category. Overlap with
ARTIDIGH 2021 - Special Session on Artificial Intelligence and Digital Heritage: Challenges and Opportunities
608