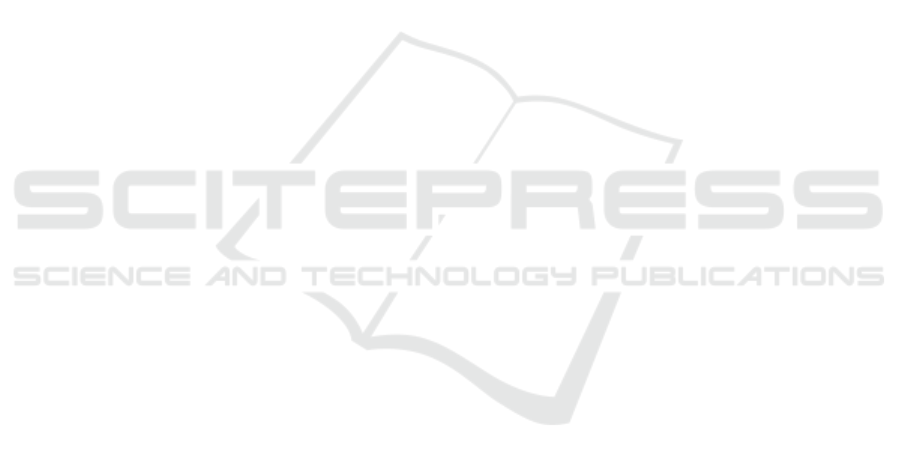
Li, L., Qin, L., Xu, Z., Yin, Y., Wang, X., Kong, B., Bai,
J., Lu, Y., Fang, Z., Song, Q., et al. (2020). Artifi-
cial intelligence distinguishes covid-19 from commu-
nity acquired pneumonia on chest ct. Radiology, page
200905.
Lin, T.-Y., Doll
´
ar, P., Girshick, R., He, K., Hariharan, B.,
and Belongie, S. (2017). Feature pyramid networks
for object detection. In Proceedings of the IEEE con-
ference on computer vision and pattern recognition,
pages 2117–2125.
Parveen, N. and Sathik, M. M. (2011). Detection of pneu-
monia in chest x-ray images. Journal of X-ray Science
and Technology, 19(4):423–428.
Qin, C., Yao, D., Shi, Y., and Song, Z. (2018). Computer-
aided detection in chest radiography based on artificial
intelligence: a survey. Biomedical engineering online,
17(1):113.
Rajpurkar, P., Irvin, J., Zhu, K., Yang, B., Mehta, H., Duan,
T., Ding, D., Bagul, A., Langlotz, C., Shpanskaya, K.,
et al. (2017). Chexnet: Radiologist-level pneumonia
detection on chest x-rays with deep learning. arXiv
preprint arXiv:1711.05225.
Randhawa, G. S., Soltysiak, M. P., El Roz, H., de Souza,
C. P., Hill, K. A., and Kari, L. (2020). Machine learn-
ing using intrinsic genomic signatures for rapid classi-
fication of novel pathogens: Covid-19 case study. Plos
one, 15(4):e0232391.
Ronneberger, O., Fischer, P., and Brox, T. (2015). U-net:
Convolutional networks for biomedical image seg-
mentation. In International Conference on Medical
image computing and computer-assisted intervention,
pages 234–241. Springer.
Shan, F., Gao, Y., Wang, J., Shi, W., Shi, N., Han, M., Xue,
Z., and Shi, Y. (2020). Lung infection quantification
of covid-19 in ct images with deep learning. arXiv
preprint arXiv:2003.04655.
Shin, H.-C., Roth, H. R., Gao, M., Lu, L., Xu, Z., Nogues,
I., Yao, J., Mollura, D., and Summers, R. M. (2016).
Deep convolutional neural networks for computer-
aided detection: Cnn architectures, dataset charac-
teristics and transfer learning. IEEE transactions on
medical imaging, 35(5):1285–1298.
Song, Y., Zheng, S., Li, L., Zhang, X., Zhang, X., Huang,
Z., Chen, J., Zhao, H., Jie, Y., Wang, R., et al. (2020).
Deep learning enables accurate diagnosis of novel
coronavirus (covid-19) with ct images. medRxiv.
Wang, M., Zhou, Y., Zong, Z., Liang, Z., Cao, Y., Tang,
H., Song, B., Huang, Z., Kang, Y., Feng, P., et al.
(2020a). A precision medicine approach to managing
2019 novel coronavirus pneumonia. Precision Clini-
cal Medicine, 3(1):14–21.
Wang, S., Kang, B., Ma, J., Zeng, X., Xiao, M., Guo, J.,
Cai, M., Yang, J., Li, Y., Meng, X., et al. (2020b). A
deep learning algorithm using ct images to screen for
corona virus disease (covid-19). MedRxiv.
Xie, X., Zhong, Z., Zhao, W., Zheng, C., Wang, F., and Liu,
J. (2020). Chest ct for typical 2019-ncov pneumonia:
relationship to negative rt-pcr testing. Radiology, page
200343.
Xu, X., Jiang, X., Ma, C., Du, P., Li, X., Lv, S., Yu, L.,
Chen, Y., Su, J., Lang, G., et al. Deep learning system
to screen coronavirus disease 2019 pneumonia. arxiv
2020. arXiv preprint arXiv:2002.09334.
Yan, Q., Wang, B., Gong, D., Luo, C., Zhao, W., Shen,
J., Shi, Q., Jin, S., Zhang, L., and You, Z. (2020).
Covid-19 chest ct image segmentation–a deep con-
volutional neural network solution. arXiv preprint
arXiv:2004.10987.
Yosinski, J., Clune, J., Bengio, Y., and Lipson, H. (2014).
How transferable are features in deep neural net-
works? In Advances in neural information processing
systems, pages 3320–3328.
Zeiler, M. D. and Fergus, R. (2014). Visualizing and under-
standing convolutional networks. In European confer-
ence on computer vision, pages 818–833. Springer.
Zhang, J., Xie, Y., Li, Y., Shen, C., and Xia, Y. (2020).
Covid-19 screening on chest x-ray images using deep
learning based anomaly detection. arXiv preprint
arXiv:2003.12338.
Zhao, J., Zhang, Y., He, X., and Xie, P. (2020a). Covid-
ct-dataset: a ct scan dataset about covid-19. arXiv
preprint arXiv:2003.13865.
Zhao, J., Zhang, Y., He, X., and Xie, P. (2020b). Covid-
ct-dataset: a ct scan dataset about covid-19. arXiv
preprint arXiv:2003.13865.
Zhou, T., Canu, S., and Ruan, S. (2020). An automatic
covid-19 ct segmentation based on u-net with atten-
tion mechanism. arXiv preprint arXiv:2004.06673.
Zhou, Z., Siddiquee, M. M. R., Tajbakhsh, N., and Liang, J.
(2018). Unet++: A nested u-net architecture for medi-
cal image segmentation. In Deep Learning in Medical
Image Analysis and Multimodal Learning for Clinical
Decision Support, pages 3–11. Springer.
ICPRAM 2021 - 10th International Conference on Pattern Recognition Applications and Methods
570