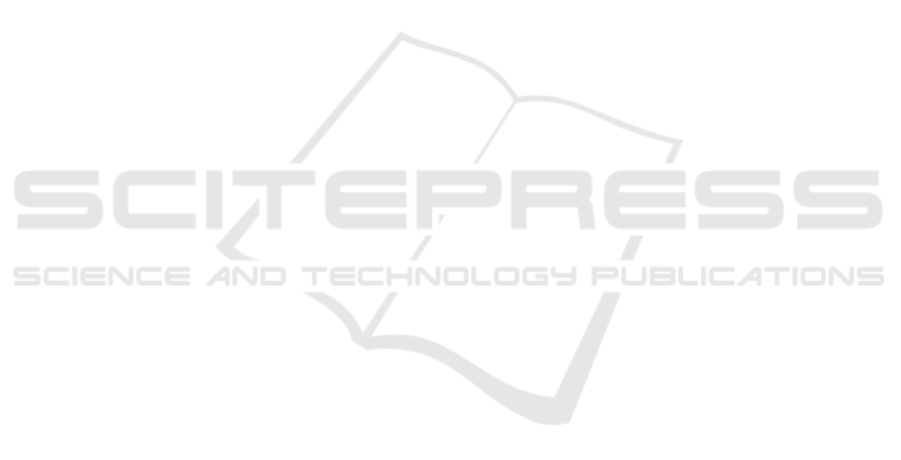
Signal Processing to Audio and Acoustics (WASPAA),
pages 313–317. IEEE.
Koizumi, Y., Yasuda, M., Murata, S., Saito, S., Uematsu,
H., and Harada, N. (2020). Spidernet: Attention
network for one-shot anomaly detection in sounds.
In ICASSP 2020-2020 IEEE International Confer-
ence on Acoustics, Speech and Signal Processing
(ICASSP), pages 281–285. IEEE.
Li, Y., Li, X., Zhang, Y., Liu, M., and Wang, W. (2018).
Anomalous sound detection using deep audio repre-
sentation and a blstm network for audio surveillance
of roads. IEEE Access, 6:58043–58055.
Liu, F. T., Ting, K. M., and Zhou, Z.-H. (2008). Isolation
forest. In 2008 Eighth IEEE International Conference
on Data Mining, pages 413–422. IEEE.
Makhzani, A., Shlens, J., Jaitly, N., Goodfellow, I., and
Frey, B. (2015). Adversarial autoencoders.
Marchi, E., Vesperini, F., Eyben, F., Squartini, S., and
Schuller, B. (2015). A novel approach for automatic
acoustic novelty detection using a denoising autoen-
coder with bidirectional lstm neural networks. In 2015
IEEE International Conference on Acoustics, Speech
and Signal Processing (ICASSP), pages 1996–2000.
IEEE.
Meire, M. and Karsmakers, P. (2019). Comparison of deep
autoencoder architectures for real-time acoustic based
anomaly detection in assets. In 2019 10th IEEE In-
ternational Conference on Intelligent Data Acquisi-
tion and Advanced Computing Systems: Technology
and Applications (IDAACS), volume 2, pages 786–
790. IEEE.
Mescheder, L., Nowozin, S., and Geiger, A. (2017). Adver-
sarial variational bayes: Unifying variational autoen-
coders and generative adversarial networks. In Pro-
ceedings of the 34th International Conference on Ma-
chine Learning-Volume 70, pages 2391–2400. JMLR.
org.
Muggleton, J. and Brennan, M. (2004). Leak noise propaga-
tion and attenuation in submerged plastic water pipes.
Journal of Sound and Vibration, 278(3):527–537.
M
¨
uller, R., Ritz, F., Illium, S., and Linnhoff-Popien, C.
(2020). Acoustic anomaly detection for machine
sounds based on image transfer learning. arXiv
preprint arXiv:2006.03429.
Nakajima, Y., Naito, T., Sunago, N., Ohshima, T., and
Ono, N. (2016). Dnn-based environmental sound
recognition with real-recorded and artificially-mixed
training data. In INTER-NOISE and NOISE-CON
Congress and Conference Proceedings, volume 253,
pages 1832–1841. Institute of Noise Control Engi-
neering.
Nguyen, D., Kirsebom, O. S., Fraz
˜
ao, F., Fablet, R.,
and Matwin, S. (2019). Recurrent neural networks
with stochastic layers for acoustic novelty detection.
In ICASSP 2019-2019 IEEE International Confer-
ence on Acoustics, Speech and Signal Processing
(ICASSP), pages 765–769. IEEE.
Ni, Q.-Q. and Iwamoto, M. (2002). Wavelet transform of
acoustic emission signals in failure of model com-
posites. Engineering Fracture Mechanics, 69(6):717–
728.
Oord, A. v. d., Dieleman, S., Zen, H., Simonyan, K.,
Vinyals, O., Graves, A., Kalchbrenner, N., Senior,
A., and Kavukcuoglu, K. (2016). Wavenet: A
generative model for raw audio. arXiv preprint
arXiv:1609.03499.
Purohit, H., Tanabe, R., Ichige, K., Endo, T., Nikaido,
Y., Suefusa, K., and Kawaguchi, Y. (2019). Mimii
dataset: Sound dataset for malfunctioning indsutrial
machine investigation and inspection. In Acoustic
Scenes and Events 2019 Workshop (DCASE2019),
page 209.
Ruff, L., Vandermeulen, R., Goernitz, N., Deecke, L., Sid-
diqui, S. A., Binder, A., M
¨
uller, E., and Kloft, M.
(2018). Deep one-class classification. In International
Conference on Machine Learning, pages 4393–4402.
Rushe, E. and Namee, B. M. (2019). Anomaly detec-
tion in raw audio using deep autoregressive networks.
In ICASSP 2019 - 2019 IEEE International Con-
ference on Acoustics, Speech and Signal Processing
(ICASSP), pages 3597–3601.
Socor
´
o, J. C., Ribera, G., Sevillano, X., and Al
´
ıas, F. (2015).
Development of an anomalous noise event detection
algorithm for dynamic road traffic noise mapping. In
Proceedings of the 22nd International Congress on
Sound and Vibration (ICSV22), Florence, Italy, pages
12–16.
Stoianov, I., Nachman, L., Madden, S., Tokmouline, T., and
Csail, M. (2007). Pipenet: A wireless sensor network
for pipeline monitoring. In 2007 6th International
Symposium on Information Processing in Sensor Net-
works, pages 264–273.
Stowell, D., Giannoulis, D., Benetos, E., Lagrange, M., and
Plumbley, M. D. (2015). Detection and classification
of acoustic scenes and events. IEEE Transactions on
Multimedia, 17(10):1733–1746.
Suefusa, K., Nishida, T., Purohit, H., Tanabe, R., Endo,
T., and Kawaguchi, Y. (2020). Anomalous sound de-
tection based on interpolation deep neural network.
In ICASSP 2020-2020 IEEE International Confer-
ence on Acoustics, Speech and Signal Processing
(ICASSP), pages 271–275. IEEE.
SWM, P. (2018). Die swm vernetzen m
¨
unchen:
Lora-netz am start f
¨
ur das internet der dinge.
https://www.swm.de/dam/swm/pressemitteilungen/
2018/06/20180604-swm-bauen-lora-netz-auf.pdf.
Ting, L., Tey, J., Tan, A., King, Y., and Faidz, A. (2019).
Improvement of acoustic water leak detection based
on dual tree complex wavelet transform-correlation
method. In IOP Conference Series: Earth and En-
vironmental Science, volume 268. IOP Publishing.
WaterUK. Leaking pipes. http://https://www.discoverwater.
co.uk/leaking-pipes. Accessed: 2020-01-20.
Acoustic Leak Detection in Water Networks
313