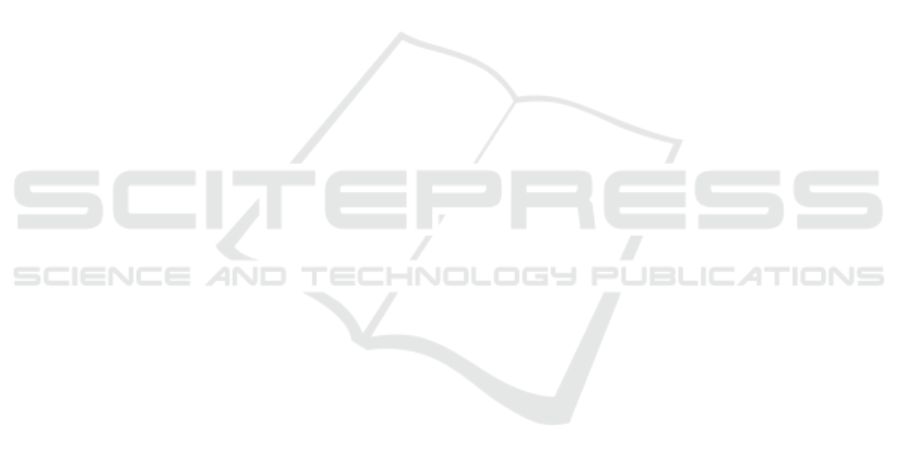
neural network (3d-cnn). Lung Cancer, 8(8):409.
Almakady, Y., Mahmoodi, S., and Bennett, M. (2020a).
Copd detection using three-dimensional gaussian
markov random fields based on binary features. In
2020 IEEE International Conference on Image Pro-
cessing (ICIP), pages 340–344. IEEE.
Almakady, Y., Mahmoodi, S., Conway, J., and Bennett,
M. (2018). Volumetric texture analysis based on
three-dimensional gaussian markov random fields for
copd detection. In Annual Conference on Medical
Image Understanding and Analysis, pages 153–164.
Springer.
Almakady, Y., Mahmoodi, S., Conway, J., and Bennett, M.
(2020b). Rotation invariant features based on three
dimensional gaussian markov random fields for vol-
umetric texture classification. Computer Vision and
Image Understanding, 194:102931.
Dey, R., Lu, Z., and Hong, Y. (2018). Diagnostic classifi-
cation of lung nodules using 3d neural networks. In
2018 IEEE 15th International Symposium on Biomed-
ical Imaging (ISBI 2018), pages 774–778. IEEE.
Dharmagunawardhana, C., Mahmoodi, S., Bennett, M., and
Niranjan, M. (2014). Quantitative analysis of pul-
monary emphysema using isotropic gaussian markov
random fields. In 2014 International Conference on
Computer Vision Theory and Applications (VISAPP),
volume 3, pages 44–53. IEEE.
Dharmagunawardhana, C., Mahmoodi, S., Bennett, M., and
Niranjan, M. (2016). Rotation invariant texture de-
scriptors based on gaussian markov random fields for
classification. Pattern Recognition Letters, 69:15–21.
Dirksen, A., Holstein-Rathlou, N.-H., Madsen, F., Skov-
gaard, L. T., Ulrik, C. S., Heckscher, T., and Kok-
Jensen, A. (1998). Long-range correlations of se-
rial fev1 measurements in emphysematous patients
and normal subjects. Journal of applied physiology,
85(1):259–265.
Du, R., Qi, S., Feng, J., Xia, S., Kang, Y., Qian, W., and
Yao, Y.-D. (2020). Identification of copd from multi-
view snapshots of 3d lung airway tree via deep cnn.
IEEE Access, 8:38907–38919.
Gomez-Donoso, F., Garcia-Garcia, A., Garcia-Rodriguez,
J., Orts-Escolano, S., and Cazorla, M. (2017). Lon-
chanet: A sliced-based cnn architecture for real-time
3d object recognition. In 2017 International Joint
Conference on Neural Networks (IJCNN), pages 412–
418. IEEE.
Han, F., Wang, H., Zhang, G., Han, H., Song, B., Li, L.,
Moore, W., Lu, H., Zhao, H., and Liang, Z. (2015).
Texture feature analysis for computer-aided diagnosis
on pulmonary nodules. Journal of digital imaging,
28(1):99–115.
Han, M. K., Agusti, A., Calverley, P. M., Celli, B. R.,
Criner, G., Curtis, J. L., Fabbri, L. M., Goldin, J. G.,
Jones, P. W., MacNee, W., et al. (2010). Chronic ob-
structive pulmonary disease phenotypes: the future of
copd. American journal of respiratory and critical
care medicine, 182(5):598–604.
Hatt, C., Galban, C., Labaki, W., Kazerooni, E., Lynch, D.,
and Han, M. (2018). Convolutional neural network
based copd and emphysema classifications are predic-
tive of lung cancer diagnosis. In Image Analysis for
Moving Organ, Breast, and Thoracic Images, pages
302–309. Springer.
He, K., Zhang, X., Ren, S., and Sun, J. (2016). Deep resid-
ual learning for image recognition. In Proceedings of
the IEEE conference on computer vision and pattern
recognition, pages 770–778.
Krizhevsky, A., Sutskever, I., and Hinton, G. E. (2012). Im-
agenet classification with deep convolutional neural
networks. In Advances in neural information process-
ing systems, pages 1097–1105.
Lapperre, T. S., Sont, J. K., van Schadewijk, A., Gos-
man, M. M., Postma, D. S., Bajema, I. M., Timens,
W., Mauad, T., Hiemstra, P. S., Group, G. S., et al.
(2007). Smoking cessation and bronchial epithelial
remodelling in copd: a cross-sectional study. Respira-
tory research, 8(1):85.
Liu, K. and Kang, G. (2017). Multiview convolutional neu-
ral networks for lung nodule classification. Interna-
tional Journal of Imaging Systems and Technology,
27(1):12–22.
Manjunath, B. S. and Ma, W.-Y. (1996). Texture features
for browsing and retrieval of image data. IEEE Trans-
actions on pattern analysis and machine intelligence,
18(8):837–842.
Mannino, D. M. and Buist, A. S. (2007). Global burden of
copd: risk factors, prevalence, and future trends. The
Lancet, 370(9589):765–773.
Paulhac, L., Makris, P., Ramel, J.-Y., et al. (2009). A solid
texture database for segmentation and classification
experiments. In VISAPP (2), pages 135–141.
Ronneberger, O., Fischer, P., and Brox, T. (2015). U-net:
Convolutional networks for biomedical image seg-
mentation. In International Conference on Medical
image computing and computer-assisted intervention,
pages 234–241. Springer.
Shen, W., Zhou, M., Yang, F., Yu, D., Dong, D., Yang,
C., Zang, Y., and Tian, J. (2017). Multi-crop convo-
lutional neural networks for lung nodule malignancy
suspiciousness classification. Pattern Recognition,
61:663–673.
Xu, Y., Huang, S., Ji, H., and Ferm
¨
uLler, C. (2012). Scale-
space texture description on sift-like textons. Com-
puter Vision and Image Understanding, 116(9):999–
1013.
Zhang, R. (2019). Making convolutional networks shift-
invariant again. In ICML.
Zhu, W., Liu, C., Fan, W., and Xie, X. (2018). Deeplung:
Deep 3d dual path nets for automated pulmonary nod-
ule detection and classification. In 2018 IEEE Win-
ter Conference on Applications of Computer Vision
(WACV), pages 673–681. IEEE.
ICPRAM 2021 - 10th International Conference on Pattern Recognition Applications and Methods
578