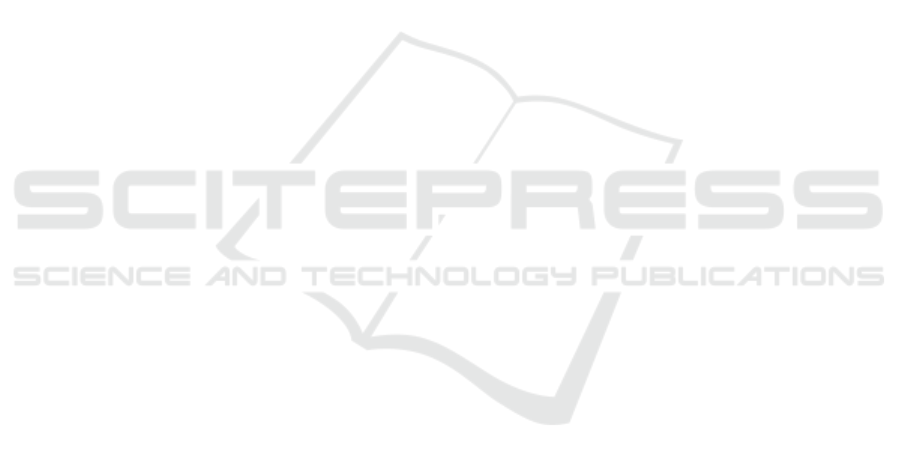
non-linear function in addition to several linear func-
tions is currently under study. A further study will
be conducted with three objectives: 1) improving
the adaptation of morph agents to include non-linear
transformations; 2) reducing the computation time of
the data agent displacement between groups by re-
ducing the necessity for a group to know every other
group; 3) reducing initial computation time by giving
a cooperative strategy to morph agents to select only
the one that needs adaptation the most. Furthermore,
group values adaptation can be improved by estimat-
ing the noise of each data agent.
ACKNOWLEDGEMENTS
This work is part of the neOCampus opera-
tion of the University Toulouse III Paul Sabatier.
www.neocampus.org
REFERENCES
Duda, R. O., Hart, P. E., et al. (2006). Pattern classification.
John Wiley & Sons.
Efron, B. (1994). Missing data, imputation, and the boot-
strap. Journal of the American Statistical Association,
89(426):463–475.
Georg
´
e, J.-P., Gleizes, M.-P., and Camps, V. (2011). Co-
operation. In Di Marzo Serugendo, G., Gleizes, M.-
P., and Karageorgos, A., editors, Self-organising Soft-
ware: From Natural to Artificial Adaptation, pages
193–226. Springer, Berlin Heidelberg.
Gibbons, J. D., Olkin, I., and Sobel, M. (1979). An intro-
duction to ranking and selection. The American Statis-
tician, 33(4):185–195.
Hall, D. L. and Llinas, J. (1997). An introduction to multi-
sensor data fusion. Proceedings of the IEEE, 85(1):6–
23.
Heaton, J. (2008). Introduction to Neural Networks for
Java, 2nd Edition. Heaton Research, Inc., 2nd edition.
Hoc, J.-M. and Carlier, X. (2002). Role of a common frame
of reference in cognitive cooperation: sharing tasks
between agents in air traffic control. Cognition, Tech-
nology & Work, 4(1):37–47.
Kumar, K., Parida, M., and Katiyar, V. (2013). Short term
traffic flow prediction for a non urban highway using
artificial neural network. Procedia - Social and Be-
havioral Sciences, 104.
Li, J., Cheng, K., Wang, S., Morstatter, F., Trevino, R. P.,
Tang, J., and Liu, H. (2017). Feature selection: A data
perspective. ACM Computing Surveys, 50(6):1–45.
Liu, T., Wei, H., and Zhang, K. (2018). Wind power predic-
tion with missing data using gaussian process regres-
sion and multiple imputation. Applied Soft Comput-
ing, 71:905 – 916.
Loke, S. W. (2019). Cooperative automated vehicles: A
review of opportunities and challenges in socially in-
telligent vehicles beyond networking. IEEE Transac-
tions on Intelligent Vehicles, 4(4):509–518.
Marwala, T. (2009). Computational Intelligence for Miss-
ing Data Imputation, Estimation, and Management:
Knowledge Optimization Techniques. IGI Global, 1st
edition.
Montgomery, D. C., Peck, E. A., and Vining, G. G. (2012).
Introduction to linear regression analysis. John Wiley
& Sons.
Niel, C. and Sinoquet, C. (2018). Optimisation par colonie
de fourmis pour la s
´
election de variables par con-
struction stochastique de couverture de Markov - Ap-
plication pour la m
´
edecine de pr
´
ecision. In 19th
edition of the annual congress of the French Soci-
ety for Operation Research and Decision Assistance,
ROADEF2018, Lorient, France.
Ryzhov, I. O., Defourny, B., and Powell, W. B. (2012).
Ranking and selection meets robust optimization. In
Proceedings of the 2012 Winter Simulation Confer-
ence (WSC), pages 1–11.
Serugendo, G. D. M., Foukia, N., Hassas, S., Karageorgos,
A., Most
´
efaoui, S. K., Rana, O. F., Ulieru, M., Valck-
enaers, P., and Van Aart, C. (2003). Self-organisation:
Paradigms and applications. In International Work-
shop on Engineering Self-Organising Applications,
pages 1–19. Springer.
Van Buuren, S. (2018). Flexible imputation of missing data.
CRC press.
Wang, H., Nie, F., and Huang, H. (2013). Multi-view clus-
tering and feature learning via structured sparsity. In
Dasgupta, S. and McAllester, D., editors, Proceed-
ings of the 30th International Conference on Machine
Learning, volume 28, pages 352–360, Atlanta, Geor-
gia, USA.
Wooldridge, M. (2009). An introduction to multiagent sys-
tems. John Wiley & Sons.
Zhang, L. and Suganthan, P. (2016). A survey of random-
ized algorithms for training neural networks. Informa-
tion Sciences, 364-365:146 – 155.
Zhao, Z. and Liu, H. (2008). Multi-source feature se-
lection via geometry-dependent covariance analysis.
In Saeys, Y., Liu, H., Inza, I., Wehenkel, L., and
de Pee, Y. V., editors, Proceedings of the Workshop on
New Challenges for Feature Selection in Data Min-
ing and Knowledge Discovery at ECML/PKDD 2008,
volume 4, pages 36–47, Antwerp, Belgium. PMLR.
ICAART 2021 - 13th International Conference on Agents and Artificial Intelligence
360