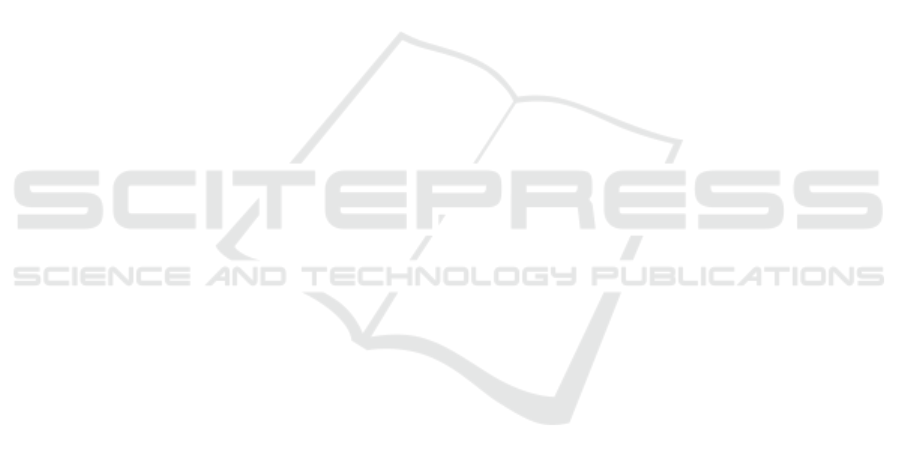
90.74% in-sample test accuracy using the proposed
system. The experiments also show that using both
visual and audio features improves performance. Our
model is very good at predicting negative and neutral
sentiment, but it is less effective at predicting pos-
itive sentiment. We hypothesize that the lower ac-
curacy for positive sentiment is due to a low num-
ber of happy video samples in RAVDESS. Moreover,
in the positive samples, positive sentiment is not ex-
pressed in every frame. In frames of the positive-
labeled videos, the subjects actually evince a neu-
tral sentiment. Thus, at test time, the model may
over-estimate the probability of neutral sentiment in
the happy sample. As a final observation, test accu-
racy with the out-of-sample dataset is lower than with
the in-sample dataset. We suppose this is because
the RAVDESS actors are Caucasian Americans, while
our out-of-sample actors are Asian.
It is worth mentioning again that we target real-
time sentiment monitoring for retail businesses; hence
the size of the neural networks used in the system
is very important, and our work compares favorably
with larger state-of-the-art models such as Inception-
ResNet-v2 and VGG16 in size. In the future, we
will employ our solution at commercial scale, e.g, to
predict customer satisfaction in multiple SME retail
shops, which usually have limited willingness to in-
vest in software and hardware capability. Moreover,
we will improve the out-of-sample performance of
our system and explore alternative methods to accu-
mulate information over a period of time.
REFERENCES
Avots, E., Sapi
´
nski, T., Bachmann, M., and Kami
´
nska, D.
(2019). Audiovisual emotion recognition in wild. Ma-
chine Vision and Applications, 30(5):975–985.
Cambria, E., Schuller, B., Xia, Y., and Havasi, C. (2013).
New avenues in opinion mining and sentiment analy-
sis. IEEE Intelligent systems, 28(2):15–21.
Chen, M., Yang, J., Zhu, X., Wang, X., Liu, M., and Song, J.
(2017). Smart home 2.0: Innovative smart home sys-
tem powered by botanical iot and emotion detection.
Mobile Networks and Applications, 22(6):1159–1169.
Chen, M., Zhang, Y., Qiu, M., Guizani, N., and Hao, Y.
(2018). Spha: Smart personal health advisor based
on deep analytics. IEEE Communications Magazine,
56(3):164–169.
Dalal, N. and Triggs, B. (2005). Histograms of oriented
gradients for human detection. In 2005 IEEE com-
puter society conference on computer vision and pat-
tern recognition (CVPR’05), volume 1, pages 886–
893. IEEE.
Glorot, X. and Bengio, Y. (2010). Understanding the diffi-
culty of training deep feedforward neural networks.
In Proceedings of the thirteenth international con-
ference on artificial intelligence and statistics, pages
249–256.
Harley, J. M., Lajoie, S. P., Frasson, C., and Hall, N. C.
(2015). An integrated emotion-aware framework for
intelligent tutoring systems. In Conati, C., Heffernan,
N., Mitrovic, A., and Verdejo, M. F., editors, Artifi-
cial Intelligence in Education, pages 616–619, Cham.
Springer International Publishing.
He, Z., Jin, T., Basu, A., Soraghan, J., Di Caterina, G., and
Petropoulakis, L. (2019). Human emotion recognition
in video using subtraction pre-processing. In Proceed-
ings of the 2019 11th International Conference on Ma-
chine Learning and Computing, pages 374–379.
Hossain, M. S. and Muhammad, G. (2019). Emotion
recognition using deep learning approach from audio–
visual emotional big data. Information Fusion, 49:69–
78.
Huang, X., Kortelainen, J., Zhao, G., Li, X., Moilanen,
A., Sepp
¨
anen, T., and Pietik
¨
ainen, M. (2016). Multi-
modal emotion analysis from facial expressions and
electroencephalogram. Computer Vision and Image
Understanding, 147:114 – 124. Spontaneous Facial
Behaviour Analysis.
Jannat, R., Tynes, I., Lime, L. L., Adorno, J., and Cana-
van, S. (2018). Ubiquitous emotion recognition us-
ing audio and video data. In Proceedings of the 2018
ACM International Joint Conference and 2018 In-
ternational Symposium on Pervasive and Ubiquitous
Computing and Wearable Computers, pages 956–959.
Kazemi, V. and Sullivan, J. (2014). One millisecond face
alignment with an ensemble of regression trees. In
Proceedings of the IEEE conference on computer vi-
sion and pattern recognition, pages 1867–1874.
Kingma, D. P. and Ba, J. (2014). Adam: A
method for stochastic optimization. arXiv preprint
arXiv:1412.6980.
Lippi, M. and Torroni, P. (2015). Argument mining: A ma-
chine learning perspective. In International Workshop
on Theory and Applications of Formal Argumentation,
pages 163–176. Springer.
Livingstone, S. R. and Russo, F. A. (2018). The ryerson
audio-visual database of emotional speech and song
(ravdess): A dynamic, multimodal set of facial and
vocal expressions in north american english. PloS one,
13(5):e0196391.
Lyon, D. A. (2009). The discrete fourier transform, part 4:
spectral leakage. Journal of object technology, 8(7).
Rajak, R. and Mall, R. (2019). Emotion recognition from
audio, dimensional and discrete categorization using
cnns. In TENCON 2019-2019 IEEE Region 10 Con-
ference (TENCON), pages 301–305. IEEE.
Rzayeva, Z. and Alasgarov, E. (2019). Facial emotion
recognition using convolutional neural networks. In
2019 IEEE 13th International Conference on Applica-
tion of Information and Communication Technologies
(AICT), pages 1–5.
Valstar, M., Gratch, J., Schuller, B., Ringeval, F., Lalanne,
D., Torres Torres, M., Scherer, S., Stratou, G., Cowie,
R., and Pantic, M. (2016). Avec 2016: Depression,
mood, and emotion recognition workshop and chal-
ICPRAM 2021 - 10th International Conference on Pattern Recognition Applications and Methods
450