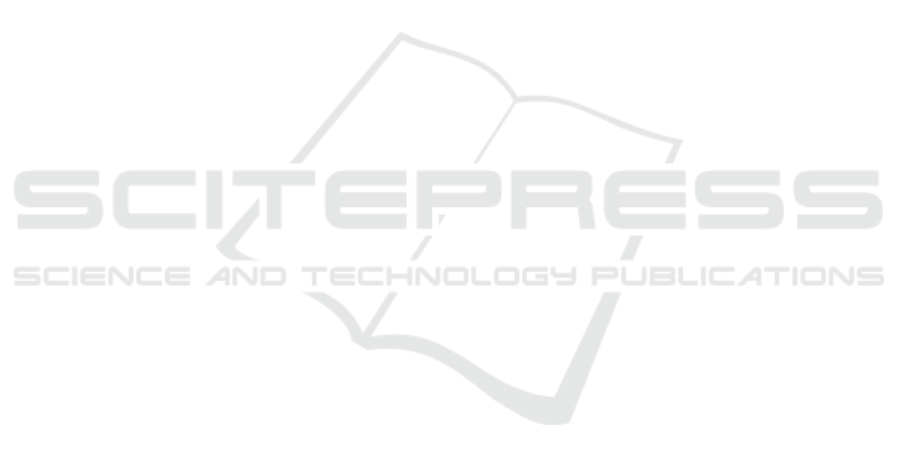
Feichtenhofer, C., Fan, H., Malik, J., and He, K. (2019).
Slowfast networks for video recognition. In ICCV,
pages 6202–6211.
Goetz, C. G., Tilley, B. C., Shaftman, S. R., Stebbins,
G. T., Fahn, S., Martinez-Martin, P., Poewe, W.,
Sampaio, C., Stern, M. B., Dodel, R., et al. (2008).
Movement Disorder Society-sponsored revision of
the Unified Parkinson’s Disease Rating Scale (MDS-
UPDRS): scale presentation and clinimetric testing re-
sults. Movement Disorders: official Journal of the
Movement Disorder Society, 23(15):2129–2170.
Hall, J., Hannuna, S., Camplani, M., Mirmehdi, M., Damen,
D., Burghardt, T., Tao, L., Paiement, A., and Crad-
dock, I. (2016). Designing a video monitoring system
for AAL applications: The SPHERE case study.
Hobert, M. A., Nussbaum, S., Heger, T., Berg, D., Maet-
zler, W., and Heinzel, S. (2019). Progressive gait
deficits in Parkinsons disease: A wearable-based bian-
nual 5-year prospective study. Frontiers in Aging Neu-
roscience, 11:22.
Hssayeni, M. D., Jimenez-Shahed, J., Burack, M. A., and
Ghoraani, B. (2019). Wearable sensors for estima-
tion of Parkinsonian tremor severity during free body
movements. Sensors, 19(19):4215.
Jeon, H., Lee, W., Park, H., Lee, H. J., Kim, S. K., Kim,
H. B., Jeon, B., and Park, K. S. (2017). Automatic
classification of tremor severity in Parkinsons disease
using a wearable device. Sensors, 17(9):2067.
Kay, W., Carreira, J., Simonyan, K., Zhang, B., Hillier, C.,
Vijayanarasimhan, S., Viola, F., Green, T., Back, T.,
Natsev, P., et al. (2017). The kinetics human action
video dataset. arXiv preprint arXiv:1705.06950.
Khokhlova, M., Migniot, C., Morozov, A., Sushkova, O.,
and Dipanda, A. (2019). Normal and pathological gait
classification LSTM model. Artificial Intelligence in
Medicine, 94:54–66.
Li, M. H., Mestre, T. A., Fox, S. H., and Taati, B.
(2018a). Vision-based assessment of Parkinsonism
and levodopa-induced dyskinesia with pose estima-
tion. Journal of Neuroengineering and Rehabilitation,
15(1):97.
Li, Q., Wang, Y., Sharf, A., Cao, Y., Tu, C., Chen, B., and
Yu, S. (2018b). Classification of gait anomalies from
Kinect. The Visual Computer, 34(2):229–241.
Lin, T.-Y., Goyal, P., Girshick, R., He, K., and Doll
´
ar, P.
(2017). Focal loss for dense object detection. In ICCV,
pages 2980–2988.
Liu, K., Liu, W., Gan, C., Tan, M., and Ma, H. (2018).
T-C3D: Temporal convolutional 3D network for real-
time action recognition. In Thirty-second AAAI Con-
ference on Artificial Intelligence.
Long, X., Gan, C., De Melo, G., Wu, J., Liu, X., and Wen,
S. (2018). Attention clusters: Purely attention based
local feature integration for video classification. In
CVPR, pages 7834–7843.
Nguyen, P., Liu, T., Prasad, G., and Han, B. (2018).
Weakly supervised action localization by sparse tem-
poral pooling network. In CVPR, pages 6752–6761.
Pei, W., Baltrusaitis, T., Tax, D. M., and Morency, L.-P.
(2017). Temporal attention-gated model for robust se-
quence classification. In CVPR, pages 6730–6739.
Pereira, C. R., Pereira, D. R., Weber, S. A., Hook, C., de Al-
buquerque, V. H. C., and Papa, J. P. (2019). A sur-
vey on computer-assisted Parkinson’s disease diagno-
sis. Artificial Intelligence in Medicine, 95:48–63.
Pintea, S. L., Zheng, J., Li, X., Bank, P. J., van Hilten, J. J.,
and van Gemert, J. C. (2018). Hand-tremor frequency
estimation in videos. In ECCV.
Sam
`
a, A., P
´
erez-L
´
opez, C., Romagosa, J., Rodriguez-
Martin, D., Catal
`
a, A., Cabestany, J., Perez-Martinez,
D., and Rodr
´
ıguez-Molinero, A. (2012). Dyskinesia
and motor state detection in parkinson’s disease pa-
tients with a single movement sensor. In 2012 An-
nual International Conference of the IEEE Engineer-
ing in Medicine and Biology Society, pages 1194–
1197. IEEE.
Sigcha, L., Costa, N., Pav
´
on, I., Costa, S., Arezes, P., L
´
opez,
J. M., and De Arcas, G. (2020). Deep Learning Ap-
proaches for Detecting Freezing of Gait in Parkinsons
Disease Patients through On-Body Acceleration Sen-
sors. Sensors, 20(7):1895.
Simonyan, K. and Zisserman, A. (2014). Two-stream con-
volutional networks for action recognition in videos.
In Advances in Neural Information Processing Sys-
tems, pages 568–576.
Szegedy, C., Liu, W., Jia, Y., Sermanet, P., Reed, S.,
Anguelov, D., Erhan, D., Vanhoucke, V., and Rabi-
novich, A. (2015). Going deeper with convolutions.
In CVPR, pages 1–9.
Wang, H. and Schmid, C. (2013). Action recognition with
improved trajectories. In Proceedings of the IEEE
international conference on computer vision, pages
3551–3558.
Wang, L., Xiong, Y., Wang, Z., Qiao, Y., Lin, D., Tang, X.,
and Van Gool, L. (2016). Temporal segment networks:
Towards good practices for deep action recognition. In
ECCV, pages 20–36. Springer.
Wei, S.-E., Ramakrishna, V., Kanade, T., and Sheikh, Y.
(2016). Convolutional pose machines. In CVPR,
pages 4724–4732.
Xia, Y., Yao, Z., Ye, Q., and Cheng, N. (2019). A dual-
modal attention-enhanced deep learning network for
quantification of Parkinsons disease characteristics.
IEEE Transactions on Neural Systems and Rehabili-
tation Engineering, 28(1):42–51.
Zach, C., Pock, T., and Bischof, H. (2007). A dual-
ity based approach for realtime TV-l 1 optical flow.
In Joint Pattern Recognition Symposium, pages 214–
223. Springer.
Zhao, A., Qi, L., Li, J., Dong, J., and Yu, H. (2018). A hy-
brid spatio-temporal model for detection and severity
rating of Parkinsons disease from gait data. Neuro-
computing, 315:1–8.
Zhao, Y., Tan, L., Lau, P., Au, W., Li, S., and Luo, N.
(2008). Factors affecting health-related quality of life
amongst Asian patients with Parkinsons disease. Eu-
ropean Journal of Neurology, 15(7):737–742.
Exploring Motion Boundaries in an End-to-End Network for Vision-based Parkinson’s Severity Assessment
97