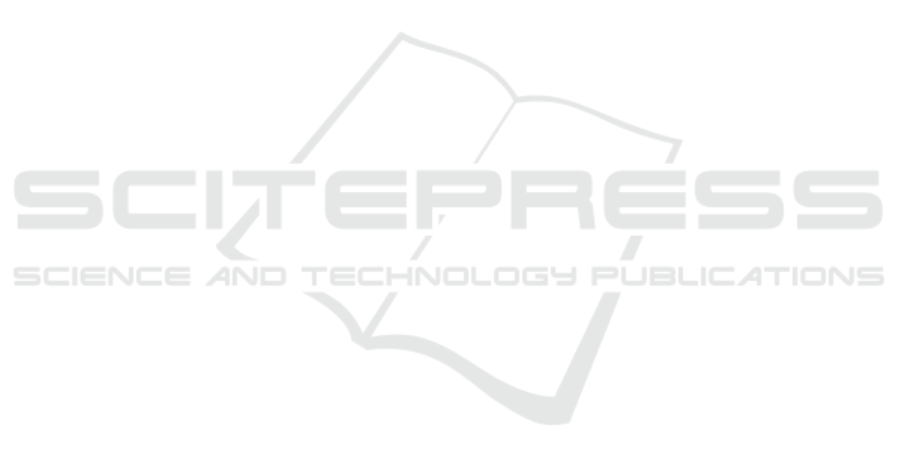
REFERENCES
Akkaya, I., Andrychowicz, M., Chociej, M., Litwin, M.,
McGrew, B., Petron, A., Paino, A., Plappert, M., Pow-
ell, G., Ribas, R., et al. (2019). Solving rubik’s cube
with a robot hand. arXiv preprint arXiv:1910.07113.
Alonso-Mora, J., Samaranayake, S., Wallar, A., Fraz-
zoli, E., and Rus, D. (2017). On-demand high-
capacity ride-sharing via dynamic trip-vehicle assign-
ment. Proc. of the National Academy of Sciences,
114(3):462–467.
Berbeglia, G., Cordeau, J.-F., and Laporte, G. (2012). A
hybrid tabu search and constraint programming algo-
rithm for the dynamic dial-a-ride problem. INFORMS
Journal on Computing, 24:343–355.
Cordeau, J.-F. and Laporte, G. (2003). A tabu search
heuristic for the static multi-vehicle dial-a-ride prob-
lem. Transportation Research Part B: Methodologi-
cal, 37(6):579 – 594.
Erhardt, G. D., Roy, S., Cooper, D., Sana, B., Chen, M.,
and Castiglione, J. (2019). Do transportation network
companies decrease or increase congestion? Science
Advances, 5(5).
Iglesias, R., Rossi, F., Wang, K., Hallac, D., Leskovec, J.,
and Pavone, M. (2017). Data-driven model predictive
control of autonomous mobility-on-demand systems.
2018 IEEE Int. Conf. on Robotics and Automation,
pages 1–7.
Ke, J., Zheng, H., Yang, H., and Chen, X. M. (2017).
Short-term forecasting of passenger demand under
on-demand ride services: A spatio-temporal deep
learning approach. Transportation Research Part C:
Emerging Technologies, 85:591 – 608.
Lin, K., Zhao, R., Xu, Z., and Zhou, J. (2018). Ef-
ficient large-scale fleet management via multi-agent
deep reinforcement learning. In Proc. of the 24th ACM
SIGKDD Int. Conf. on Knowledge Discovery & Data
Mining, KDD ’18, pages 1774–1783, New York, NY,
USA. ACM.
Ma, W., Pi, X., and Qian, S. (2019). Estimat-
ing multi-class dynamic origin-destination demand
through a forward-backward algorithm on computa-
tional graphs.
Miao, F., Lin, S., Munir, S., A Stankovic, J., Huang, H.,
Zhang, D., He, T., and Pappas, G. (2015). Taxi dis-
patch with real-time sensing data in metropolitan ar-
eas — a receding horizon control approach *. IEEE
Trans. on Automation Science and Engineering, 13.
Mnih, V., Kavukcuoglu, K., Silver, D., Rusu, A. A., Ve-
ness, J., Bellemare, M. G., Graves, A., Riedmiller,
M., Fidjeland, A. K., Ostrovski, G., Petersen, S.,
Beattie, C., Sadik, A., Antonoglou, I., King, H., Ku-
maran, D., Wierstra, D., Legg, S., and Hassabis, D.
(2015). Human-level control through deep reinforce-
ment learning. Nature, 518(7540):529–533.
Nakashima, H., Matsubara, H., Hirata, K., Shiraishi, Y.,
Sano, S., Kanamori, R., Noda, I., Yamashita, T., and
Koshiba, H. (2013). Design of the smart access vehi-
cle system with large scale ma simulation. In Proc. of
1st Int. Workshop on Multiagent-Based Societal Sys-
tems.
NYC (2020). Nyc taxi limousine commission-trip record
data-nyc.gov. http://www.nyc.gov.
Oda, T. and Joe-Wong, C. (2018). MOVI: A Model-Free
Approach to Dynamic Fleet Management. In IEEE
INFOCOM 2018 - IEEE Conf. on Computer Commu-
nications, pages 2708–2716.
Silver, D., Hubert, T., Schrittwieser, J., Antonoglou, I., Lai,
M., Guez, A., Lanctot, M., Sifre, L., Kumaran, D.,
Graepel, T., Lillicrap, T., Simonyan, K., and Hass-
abis, D. (2018). A general reinforcement learning
algorithm that masters chess, shogi, and go through
self-play. Science, 362(6419):1140–1144.
Wang, Z., Schaul, T., Hessel, M., Hasselt, H., Lanctot, M.,
and Freitas, N. (2016). Dueling network architectures
for deep reinforcement learning. In Int. Conf. on Ma-
chine Learning, pages 1995–2003.
Wen, J., Zhao, J., and Jaillet, P. (2017). Rebalancing shared
mobility-on-demand systems: A reinforcement learn-
ing approach. In 2017 IEEE 20th Int. Conf. on Intelli-
gent Transportation Systems (ITSC), pages 220–225.
Yaraghi, N. and Ravi, S. (2017). The Current and Future
State of the Sharing Economy. SSRN Electronic Jour-
nal.
Yoshida, N., Noda, I., and Sugawara, T. (2020). Multi-agent
service area adaptation for ride-sharing using deep re-
inforcement learning. In Advances in Practical Ap-
plications of Agents, Multi-Agent Systems, and Trust-
worthiness. The PAAMS Collection, pages 363–375,
Cham. Springer.
ICAART 2021 - 13th International Conference on Agents and Artificial Intelligence
112