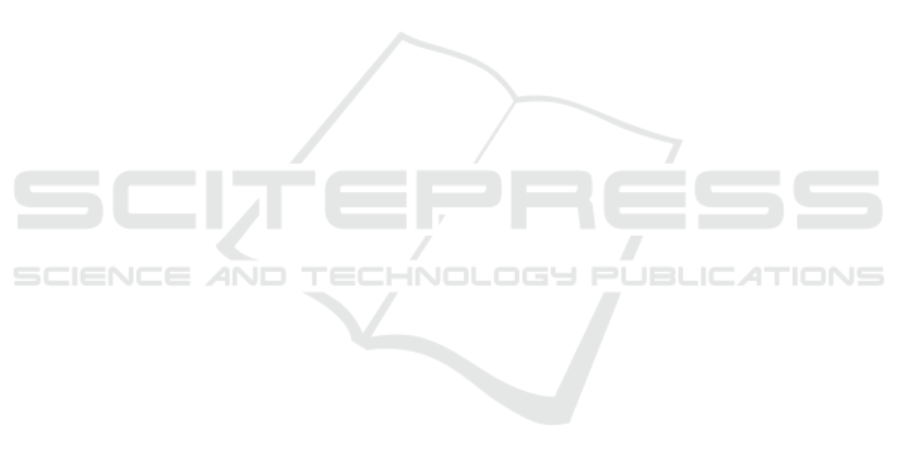
for large-vocabulary speech recognition. IEEE Trans-
actions on audio, speech, and language processing,
20(1):30–42.
Fazil, M. and Abulaish, M. (2018). A hybrid approach
for detecting automated spammers in twitter. IEEE
Transactions on Information Forensics and Security,
13(11):2707–2719.
Ghosh, S. and Shah, C. (2018). Towards automatic fake
news classification. Proceedings of the Association
for Information Science and Technology, 55(1):805–
807.
Hochreiter, S. and Schmidhuber, J. (1997). Long short-term
memory. Neural computation, 9(8):1735–1780.
Kalchbrenner, N., Grefenstette, E., and Blunsom, P. (2014).
A convolutional neural network for modelling sen-
tences. In Proceedings of the 52nd Annual Meeting
of the Association for Computational Linguistics (Vol-
ume 1: Long Papers), pages 655–665.
Kim, Y. (2014). Convolutional neural networks for sentence
classification proceedings of the 2014 conference on
empirical methods in natural language processing,
emnlp 2014, october 25-29, 2014, doha, qatar, a meet-
ing of sigdat, a special interest group of the acl. Asso-
ciation for Computational Linguistics, Doha, Qatar.
Kumar, S. and Shah, N. (2018). False information on web
and social media: A survey. arXiv, pages arXiv–1804.
Ma, J., Gao, W., Mitra, P., Kwon, S., Jansen, B. J., Wong,
K.-F., and Cha, M. (2016). Detecting rumors from
microblogs with recurrent neural networks. In Pro-
ceedings of the Twenty-Fifth International Joint Con-
ference on Artificial Intelligence, pages 3818–3824.
Ma, J., Gao, W., and Wong, K.-F. (2018). Rumor detec-
tion on twitter with tree-structured recursive neural
networks. In Proceedings of the 56th Annual Meet-
ing of the Association for Computational Linguistics
(Volume 1: Long Papers), pages 1980–1989.
Miller, T. P. and Leon, A. (2017). Introduction to special
issue on literacy, democracy, and fake news: Making
it right in the era of fast and slow literacies. Literacy
in Composition Studies, 5(2):10–23.
Roy, A., Basak, K., Ekbal, A., and Bhattacharyya, P. (2018).
A deep ensemble framework for fake news detection
and classification. arXiv, pages arXiv–1811.
Ruchansky, N., Seo, S., and Liu, Y. (2017). Csi: A hybrid
deep model for fake news detection. In Proceedings
of the 2017 ACM on Conference on Information and
Knowledge Management, pages 797–806.
Sainath, T. N., Vinyals, O., Senior, A., and Sak, H. (2015).
Convolutional, long short-term memory, fully con-
nected deep neural networks. In 2015 IEEE Inter-
national Conference on Acoustics, Speech and Signal
Processing (ICASSP), pages 4580–4584. IEEE.
Shu, K., Sliva, A., Wang, S., Tang, J., and Liu, H. (2017).
Fake news detection on social media: A data mining
perspective. ACM SIGKDD explorations newsletter,
19(1):22–36.
Vasudevan, V., Zoph, B., Shlens, J., and Le, Q. V. (2019).
Neural architecture search for convolutional neural
networks. US Patent 10,521,729.
Wang, Y., Ma, F., Jin, Z., Yuan, Y., Xun, G., Jha, K., Su,
L., and Gao, J. (2018). Eann: Event adversarial neu-
ral networks for multi-modal fake news detection. In
Proceedings of the 24th ACM SIGKDD International
Conference on Knowledge Discovery & Data Mining,
pages 849–857. ACM.
Yang, Y., Zheng, L., Zhang, J., Cui, Q., Li, Z., and Yu,
P. S. (2018). Ti-cnn: Convolutional neural networks
for fake news detection. arXiv, pages arXiv–1806.
Yu, F., Liu, Q., Wu, S., Wang, L., and Tan, T. (2017). A con-
volutional approach for misinformation identification.
In Proceedings of the 26th International Joint Confer-
ence on Artificial Intelligence, pages 3901–3907.
Zhang, J., Dong, B., and Philip, S. Y. (2020). Fakedetector:
Effective fake news detection with deep diffusive neu-
ral network. In 2020 IEEE 36th International Confer-
ence on Data Engineering (ICDE), pages 1826–1829.
IEEE.
Zhong, B., Xing, X., Love, P., Wang, X., and Luo, H.
(2019). Convolutional neural network: Deep learning-
based classification of building quality problems. Ad-
vanced Engineering Informatics, 40:46–57.
Zubiaga, A., Liakata, M., and Procter, R. (2016). Learning
reporting dynamics during breaking news for rumour
detection in social media. arXiv, pages arXiv–1610.
ICAART 2021 - 13th International Conference on Agents and Artificial Intelligence
1072