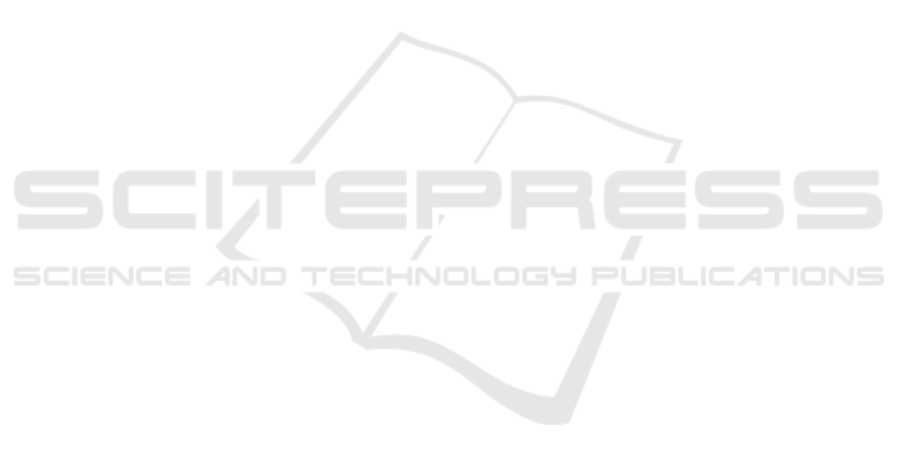
REFERENCES
Abadi, M., Barham, P., Chen, J., Chen, Z., Davis, A., Dean,
J., Devin, M., Ghemawat, S., Irving, G., Isard, M.,
et al. (2016). Tensorflow: A system for large-scale
machine learning. In 12th USENIX symposium on
operating systems design and implementation (OSDI
16), pages 265–283.
Armstrong, J. S. (2001). Principles of forecasting: a hand-
book for researchers and practitioners, volume 30.
Springer Science & Business Media.
Brandenburg, C. and Arnberger, A. (2001). The influence
of the weather upon recreation activities. In Pro-
ceedings of the First International Workshop on Cli-
mate, Tourism and Recreation, International Society
of Biometeorology, Porto Carras, Halkidiki, Greece,
pages 123–132.
Chen, T., He, T., Benesty, M., Khotilovich, V., and Tang,
Y. (2015). Xgboost: extreme gradient boosting. R
package version 0.4-2, pages 1–4.
Dwyer, J. F. (1988). Predicting daily use of urban for-
est recreation sites. Landscape and Urban Planning,
15(1-2):127–138.
Hu, C., Chen, M., and McCain, S.-L. C. (2004). Forecasting
in short-term planning and management for a casino
buffet restaurant. Journal of Travel & Tourism Mar-
keting, 16(2-3):79–98.
Hyndman, R. J. and Koehler, A. B. (2006). Another look at
measures of forecast accuracy. International journal
of forecasting, 22(4):679–688.
Ke, G., Meng, Q., Finley, T., Wang, T., Chen, W., Ma, W.,
Ye, Q., and Liu, T.-Y. (2017). Lightgbm: A highly ef-
ficient gradient boosting decision tree. In Advances in
neural information processing systems, pages 3146–
3154.
Lim, B., Arik, S. O., Loeff, N., and Pfister, T. (2019).
Temporal fusion transformers for interpretable multi-
horizon time series forecasting. arXiv preprint
arXiv:1912.09363.
Makridakis, S., Spiliotis, E., and Assimakopoulos, V.
(2020a). The m4 competition: 100,000 time series
and 61 forecasting methods. International Journal of
Forecasting, 36(1):54–74.
Makridakis, S., Spiliotis, E., and Assimakopoulos, V.
(2020b). The m5 accuracy competition: Results, find-
ings and conclusions.
Pedregosa, F., Varoquaux, G., Gramfort, A., Michel, V.,
Thirion, B., Grisel, O., Blondel, M., Prettenhofer, P.,
Weiss, R., Dubourg, V., et al. (2011). Scikit-learn:
Machine learning in python. the Journal of machine
Learning research, 12:2825–2830.
Pf
¨
affli, D., Lionetti, S., Wegelin, P., Pouly, M., and vor der
Br
¨
uck, T. (2020). Tourism forecast with weather,
event, and cross-industry data. https://dx.doi.org/10.
5281/zenodo.4133644.
Salinas, D., Flunkert, V., Gasthaus, J., and Januschowski, T.
(2020). Deepar: Probabilistic forecasting with autore-
gressive recurrent networks. International Journal of
Forecasting, 36(3):1181–1191.
Shih, C. and Nicholls, S. (2011). Modeling the influence of
weather variability on leisure traffic. Tourism Analy-
sis, 16(3):315–328.
Shih, C., Nicholls, S., and Holecek, D. F. (2009). Impact
of weather on downhill ski lift ticket sales. Journal of
Travel Research, 47(3):359–372.
Smyl, S. (2020). A hybrid method of exponential smoothing
and recurrent neural networks for time series forecast-
ing. International Journal of Forecasting, 36(1):75–
85.
Song, H., Witt, S. F., and Jensen, T. C. (2003).
Tourism forecasting: Accuracy of alternative econo-
metric models. International Journal of Forecasting,
19(1):123–141.
Taylor, S. J. and Letham, B. (2018). Forecasting at scale.
The American Statistician, 72(1):37–45.
Zhou, Y., Li, J., Chen, H., Wu, Y., Wu, J., and Chen, L.
(2020). A spatiotemporal attention mechanism-based
model for multi-step citywide passenger demand pre-
diction. Information Sciences, 513:372–385.
ICAART 2021 - 13th International Conference on Agents and Artificial Intelligence
1104