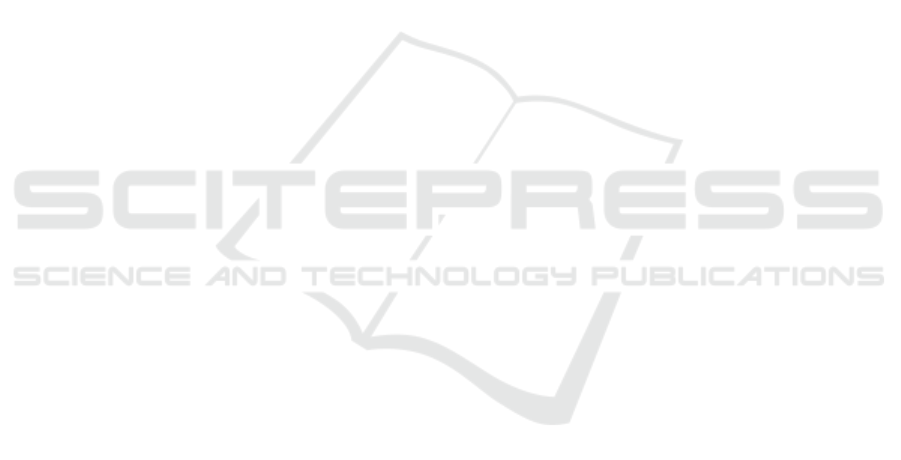
Cao, Z., Hidalgo, G., Simon, T., Wei, S.-E., and Sheikh,
Y. (2018). Openpose: realtime multi-person 2d pose
estimation using part affinity fields. arXiv preprint
arXiv:1812.08008.
Chen, C., Jafari, R., and Kehtarnavaz, N. (2015). Utd-mhad:
A multimodal dataset for human action recognition
utilizing a depth camera and a wearable inertial sen-
sor. In 2015 IEEE International conference on image
processing (ICIP), pages 168–172. IEEE.
Cho, K., Van Merri
¨
enboer, B., Gulcehre, C., Bahdanau, D.,
Bougares, F., Schwenk, H., and Bengio, Y. (2014).
Learning phrase representations using rnn encoder-
decoder for statistical machine translation. arXiv
preprint arXiv:1406.1078.
Du, Y., Wang, W., and Wang, L. (2015). Hierarchical recur-
rent neural network for skeleton based action recog-
nition. In Proceedings of the IEEE conference on
computer vision and pattern recognition, pages 1110–
1118.
Han, Y., Zhang, P., Zhuo, T., Huang, W., and Zhang,
Y. (2018). Going deeper with two-stream convnets
for action recognition in video surveillance. Pattern
Recognition Letters, 107:83–90.
Hou, Y., Li, Z., Wang, P., and Li, W. (2016). Skeleton op-
tical spectra-based action recognition using convolu-
tional neural networks. IEEE Transactions on Circuits
and Systems for Video Technology, 28(3):807–811.
Hussain, Z., Sheng, M., and Zhang, W. E. (2019). Different
approaches for human activity recognition: A survey.
arXiv preprint arXiv:1906.05074.
Hussein, M. E., Torki, M., Gowayyed, M. A., and El-Saban,
M. (2013). Human action recognition using a tem-
poral hierarchy of covariance descriptors on 3d joint
locations. In Twenty-Third International Joint Con-
ference on Artificial Intelligence.
Ji, S., Xu, W., Yang, M., and Yu, K. (2012). 3d convolu-
tional neural networks for human action recognition.
IEEE transactions on pattern analysis and machine
intelligence, 35(1):221–231.
Johansson, G. (1973). Visual perception of biological mo-
tion and a model for its analysis. Perception & psy-
chophysics, 14(2):201–211.
Ke, Q., Bennamoun, M., An, S., Sohel, F., and Boussaid, F.
(2017). A new representation of skeleton sequences
for 3d action recognition. In Proceedings of the IEEE
conference on computer vision and pattern recogni-
tion, pages 3288–3297.
Laraba, S., Brahimi, M., Tilmanne, J., and Dutoit, T. (2017).
3d skeleton-based action recognition by representing
motion capture sequences as 2d-rgb images. Com-
puter Animation and Virtual Worlds, 28(3-4):e1782.
Li, C., Hou, Y., Wang, P., and Li, W. (2017a). Joint dis-
tance maps based action recognition with convolu-
tional neural networks. IEEE Signal Processing Let-
ters, 24(5):624–628.
Li, C., Wang, P., Wang, S., Hou, Y., and Li, W. (2017b).
Skeleton-based action recognition using lstm and cnn.
In 2017 IEEE International Conference on Multime-
dia & Expo Workshops (ICMEW), pages 585–590.
IEEE.
Liu, J., Akhtar, N., and Mian, A. (2019). Skepxels:
Spatio-temporal image representation of human skele-
ton joints for action recognition. In CVPR Workshops.
Liu, J., Wang, G., Duan, L.-Y., Abdiyeva, K., and Kot,
A. C. (2017). Skeleton-based human action recog-
nition with global context-aware attention lstm net-
works. IEEE Transactions on Image Processing,
27(4):1586–1599.
Mandic, D. and Chambers, J. (2001). Recurrent neural net-
works for prediction: learning algorithms, architec-
tures and stability. Wiley.
Martin, P.-E., Benois-Pineau, J., P
´
eteri, R., and Morlier, J.
(2018). Sport action recognition with siamese spatio-
temporal cnns: Application to table tennis. In 2018
International Conference on Content-Based Multime-
dia Indexing (CBMI), pages 1–6. IEEE.
McNally, W., Wong, A., and McPhee, J. (2018). Ac-
tion recognition using deep convolutional neural net-
works and compressed spatio-temporal pose encod-
ings. Journal of Computational Vision and Imaging
Systems, 4(1):3–3.
Ouyang, X., Xu, S., Zhang, C., Zhou, P., Yang, Y., Liu,
G., and Li, X. (2019). A 3d-cnn and lstm based multi-
task learning architecture for action recognition. IEEE
Access, 7:40757–40770.
Savitzky, A. and Golay, M. J. (1964). Smoothing and dif-
ferentiation of data by simplified least squares proce-
dures. Analytical chemistry, 36(8):1627–1639.
Sewell, M. (2008). Ensemble learning. RN, 11(02).
Shahroudy, A., Liu, J., Ng, T.-T., and Wang, G. (2016). Ntu
rgb+ d: A large scale dataset for 3d human activity
analysis. In Proceedings of the IEEE conference on
computer vision and pattern recognition, pages 1010–
1019.
Simonyan, K. and Zisserman, A. (2014). Two-stream con-
volutional networks for action recognition in videos.
In Advances in neural information processing sys-
tems, pages 568–576.
Ullah, A., Ahmad, J., Muhammad, K., Sajjad, M., and Baik,
S. W. (2017). Action recognition in video sequences
using deep bi-directional lstm with cnn features. IEEE
Access, 6:1155–1166.
Vemulapalli, R., Arrate, F., and Chellappa, R. (2014). Hu-
man action recognition by representing 3d skeletons
as points in a lie group. In Proceedings of the IEEE
conference on computer vision and pattern recogni-
tion, pages 588–595.
Wang, P., Li, Z., Hou, Y., and Li, W. (2016). Action recog-
nition based on joint trajectory maps using convolu-
tional neural networks. In Proceedings of the 24th
ACM international conference on Multimedia, pages
102–106.
Weiyao, X., Muqing, W., Min, Z., Yifeng, L., Bo, L., and
Ting, X. (2019). Human action recognition using mul-
tilevel depth motion maps. IEEE Access, 7:41811–
41822.
Ye, M., Zhang, Q., Wang, L., Zhu, J., Yang, R., and Gall,
J. (2013). A survey on human motion analysis from
depth data. In Time-of-flight and depth imaging. sen-
sors, algorithms, and applications, pages 149–187.
Springer.
ICAART 2021 - 13th International Conference on Agents and Artificial Intelligence
358