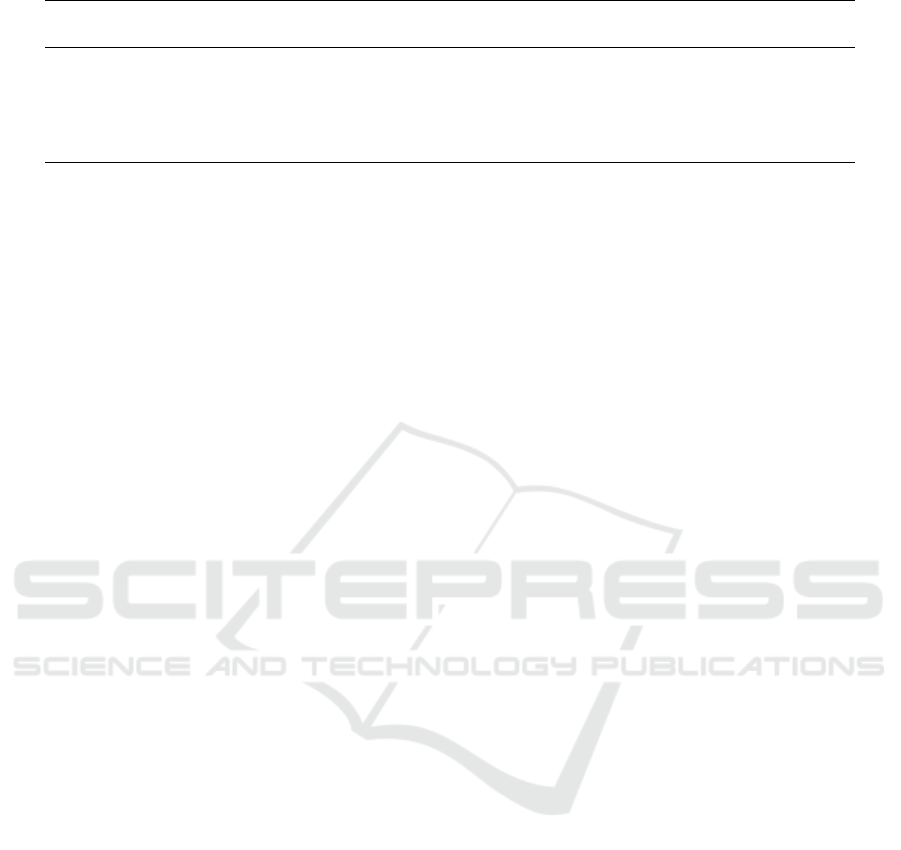
Table 2: Average IoU and the number of detected text lines.
Case Model Data sets for Data set for test Avg. IoU # of detected text lines
training and validation
Case 1 M1 I1-1 and I1-2 I1-3 0.897 514
Case 2 M2 I2-1 and I2-2 I2-3 0.892 1,676
Case 3 M2 I2-1 and I2-2 I1-3 0.806 574
Case 4 M3 I3-1 and I3-2 I3-3 0.903 1,320
Case 5 M3 I3-1 and I3-2 I1-3 0.877 682
• If we use the document image synthesis method
and the object detection algorithm for text line
segmentation, we need post processes such as re-
moval of redundant detected text lines and expan-
sion of detected text lines.
5 CONCLUSION
We proposed an annotated Japanese historical doc-
ument image synthesis method, and experimentally
applied it to text line segmentation using a machine
learning-based object detection algorithm YOLOv3
where synthetic document images were used as train-
ing data for YOLOv3. The experimental results show
that a model trained by the synthetic annotated docu-
ment images can be competitive with a model trained
by the manually annotated real document images
from the view point of intersection-over-union. Pa-
rameters in our method are, however, needed to man-
ually adjust to generate such competitive document
images.
Future work would be as follows. To confirm
applicability of our method, we need to apply our
method to same type of other historical documents
because we applied it to only a historical document.
Automatic parameter adjustment methods for docu-
ment image synthesis and post-processing for seg-
mented text lines will improve text line segmenta-
tion results. The post-processing, for example, would
be to remove segmented text lines included in other
segmented ones and to extend segmented text lines
to include missing parts of the text lines. Our docu-
ment image synthesis method will be also applicable
to character segmentation.
REFERENCES
Aoike, T., Kinoshita, T., Satomi, W., and Kawashima, T.
(2019). Construction and publication of book layout
datasets for machine learning. In Proceedings of the
Computers and the Humanities Symposium, volume
2019, pages 115–120.
Capobianco, S. and Marinai, S. (2017). Docemul: A
toolkit to generate structured historical documents. In
2017 14th IAPR International Conference on Docu-
ment Analysis and Recognition (ICDAR), volume 01,
pages 1186–1191.
Clanuwat, T., Bober-Irizar, M., Kitamoto, A., Lamb, A.,
Yamamoto, K., and Ha, D. (2018). Deep learning for
classical japanese literature. CoRR, abs/1812.01718.
Inuzuka, N. and Suzuki, T. (2020). Text line segmentation
for japanese historical document images using deep
learning and data synthesis. SIG Technical Reports
(CH), 2020-CH-122(4):1–6.
Ogiso, T., Ogura, H., Tanaka, M., Kondo, A., and Den,
Y. (2010). Development of an electrical dictionary
for morphological analysis of classical japanese. SIG
Technical Reports (CH), 2010-CH-85(4):1–8.
Otsu, N. (1979). A threshold selection method from gray-
level histograms. IEEE Transactions on Systems,
Man, and Cybernetics, 9(1):62–66.
Pondenkandath, V., Alberti, M., Diatta, M., Ingold, R., and
Liwicki, M. (2019). Historical document synthesis
with generative adversarial networks. In 2019 Interna-
tional Conference on Document Analysis and Recog-
nition Workshops (ICDARW), volume 5, pages 146–
151.
Redmon, J. and Farhadi, A. (2018). Yolov3: An incremental
improvement. arXiv.
Reizei, T. (1994). Tales of Ise (photocopy). Kasama Shoin.
Sando, K., Suzuki, T., and Aiba, A. (2018). A con-
straint solving web service for a handwritten japanese
historical kana reprint support system. In van den
Herik, H. J. and Rocha, A. P., editors, Agents and
Artificial Intelligence - 10th International Conference,
ICAART 2018, Funchal, Madeira, Portugal, January
16-18, 2018, Revised Selected Papers, volume 11352
of Lecture Notes in Computer Science, pages 422–
442. Springer.
Yamazaki, A., Sando, K., Suzuki, T., and Aiba, A. (2018).
A handwritten japanese historical kana reprint support
system: Development of a graphical user interface. In
Proceedings of the ACM Symposium on Document En-
gineering 2018, New York, NY, USA. Association for
Computing Machinery.
ICPRAM 2021 - 10th International Conference on Pattern Recognition Applications and Methods
634