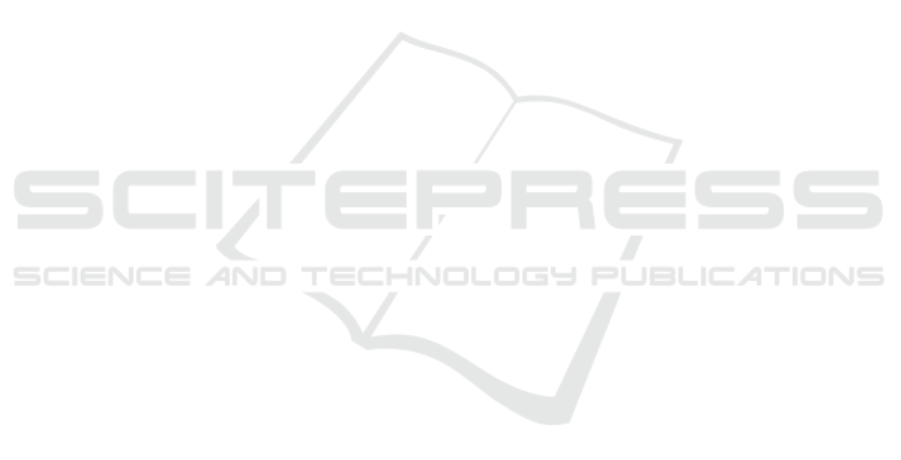
Cuff, B. M., Brown, S. J., Taylor, L., and Howat, D. J.
(2016). Empathy: A review of the concept. Emotion
review, 8(2):144–153.
Fuentes, J. M., Taverner, J., Rincon, J. A., and Botti, V.
(2020). Towards a classifier to recognize emotions us-
ing voice to improve recommendations. In Interna-
tional Conference on Practical Applications of Agents
and Multi-Agent Systems, pages 218–225. Springer.
Garc
´
ıa-Pe
˜
nalvo, F. J. and Kearney, N. A. (2016). Networked
youth research for empowerment in digital society:
The wyred project. In Proceedings of the Fourth In-
ternational Conference on Technological Ecosystems
for Enhancing Multiculturality, page 39. Association
for Computing Machinery.
G
´
omez-Gal
´
an, J., Mart
´
ınez-L
´
opez, J.
´
A., L
´
azaro-P
´
erez, C.,
and Sarasola S
´
anchez-Serrano, J. L. (2020). So-
cial networks consumption and addiction in col-
lege students during the covid-19 pandemic: Educa-
tional approach to responsible use. Sustainability,
12(18):7737.
Grawemeyer, B., Johnson, H., Brosnan, M., Ashwin, E.,
and Benton, L. (2012). Developing an embodied ped-
agogical agent with and for young people with autism
spectrum disorder. In International Conference on In-
telligent Tutoring Systems, pages 262–267. Springer.
Hoffman, M. L. (2001). Empathy and moral development:
Implications for caring and justice. Cambridge Uni-
versity Press.
Jasmontaite, L. and De Hert, P. (2015). The eu, children
under 13 years, and parental consent: a human rights
analysis of a new, age-based bright-line for the pro-
tection of children on the internet. International Data
Privacy Law, 5(1):20–33.
Karal, H., Kokoc, M., and Cakir, O. (2017). Impact of the
educational use of facebook group on the high school
students proper usage of language. Education and In-
formation Technologies, 22(2):677–695.
Kauppinen, A. (2014). Empathy, emotion regulation, and
moral judgment. Oxford University Press.
Machimbarrena, J. M., Calvete, E., Fern
´
andez-Gonz
´
alez,
L.,
´
Alvarez-Bard
´
on, A.,
´
Alvarez-Fern
´
andez, L., and
Gonz
´
alez-Cabrera, J. (2018). Internet risks: An
overview of victimization in cyberbullying, cyber dat-
ing abuse, sexting, online grooming and problematic
internet use. International journal of environmental
research and public health, 15(11):2471.
Micheli, M. (2016). Social networking sites and low-
income teenagers: between opportunity and in-
equality. Information, Communication & Society,
19(5):565–581.
Obaid, M., Aylett, R., Barendregt, W., Basedow, C., Cor-
rigan, L. J., Hall, L., Jones, A., Kappas, A., K
¨
uster,
D., Paiva, A., et al. (2018). Endowing a robotic tu-
tor with empathic qualities: Design and pilot evalu-
ation. International Journal of Humanoid Robotics,
15(06):1850025.
Paiva, A., Leite, I., Boukricha, H., and Wachsmuth, I.
(2017). Empathy in virtual agents and robots: a sur-
vey. ACM Transactions on Interactive Intelligent Sys-
tems (TiiS), 7(3):1–40.
Primack, B. A., Karim, S. A., Shensa, A., Bowman, N.,
Knight, J., and Sidani, J. E. (2019). Positive and neg-
ative experiences on social media and perceived so-
cial isolation. American Journal of Health Promotion,
33(6):859–868.
Rodrigues, S. H., Mascarenhas, S., Dias, J., and Paiva,
A. (2015). A process model of empathy for virtual
agents. Interacting with Computers, 27(4):371–391.
Ruiz-Dolz, R., Heras, S., Alemany, J., and Garc
´
ıa-Fornes,
A. (2019). Towards an argumentation system for as-
sisting users with privacy management in online social
networks. In CMNA@ PERSUASIVE, pages 17–28.
Russell, J. A. (1980). A circumplex model of affect. Journal
of Personality and Social Psychology, 39(6):1161–
1178.
Serholt, S. and Barendregt, W. (2016). Robots tutoring
children: Longitudinal evaluation of social engage-
ment in child-robot interaction. In Proceedings of
the 9th nordic conference on human-computer inter-
action, pages 1–10.
Sharifara, A., Rahim, M. S. M., and Anisi, Y. (2014). A
general review of human face detection including a
study of neural networks and haar feature-based cas-
cade classifier in face detection. In 2014 International
Symposium on Biometrics and Security Technologies
(ISBAST), pages 73–78. IEEE.
Silva, C. S., Barbosa, G. A., Silva, I. S., Silva, T. S.,
Mour
˜
ao, F., and Coutinho, F. (2017). Privacy for chil-
dren and teenagers on social networks from a usability
perspective: a case study on facebook. In Proceedings
of the 2017 ACM on Web Science Conference, pages
63–71.
Stueber, K. (2013). Empathy. In E. N. Zalta
(Ed.), The Standord Encyclopedia of Philosophy
(http://plato.stanford.edu/entries/empathy/). Stanfotd:
The Metaphysics Research Lab, Stanford University.
Taverner, J., Alfonso, B., Vivancos, E., and Botti, V. J.
(2018a). Modeling personality in the affective agent
architecture GenIA3. In International Conference on
Agents and Artificial Intelligence, pages 236–243.
Taverner, J., Ruiz, R., del Val, E., Diez, C., and Alemany,
J. (2018b). Image analysis for privacy assessment in
social networks. In International Symposium on Dis-
tributed Computing and Artificial Intelligence, pages
1–4. Springer.
Taverner, J., Vivancos, E., and Botti, V. (2020). A fuzzy ap-
praisal model for affective agents adapted to cultural
environments using the pleasure and arousal dimen-
sions. Information Sciences.
Taverner, J., Vivancos, E., and Botti, V. (2020). A
multidimensional culturally adapted representation of
emotions for affective computational simulation and
recognition. IEEE Transactions on Affective Comput-
ing, pages 1–1.
Yalc¸ın,
¨
O. N. (2020). Empathy framework for embodied
conversational agents. Cognitive Systems Research,
59:123–132.
Towards a Model of Empathic Pedagogical Agent for Educating Children and Teenagers on Good Practices in the Use of Social Networks
445