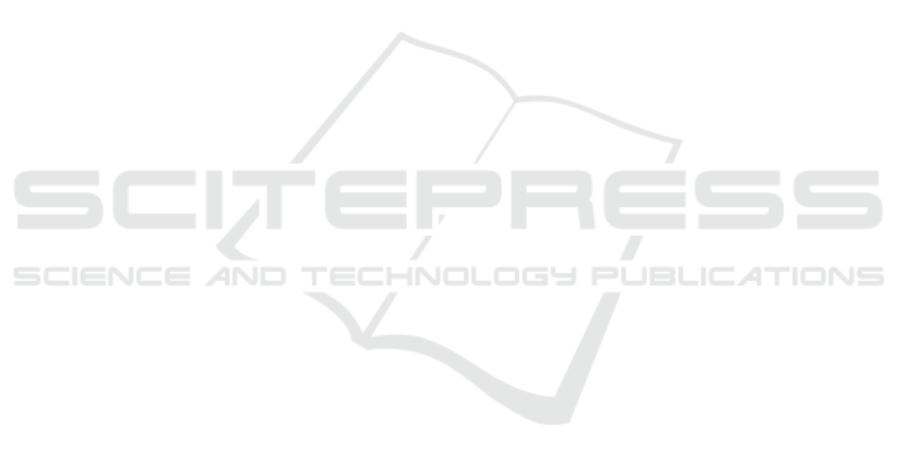
5 CONCLUSIONS AND FUTURE
WORK
We have applied a joint learning approach to integrat-
ing the training of a pair of TMs in a unified learn-
ing process with the help of monolingual data from
both source and target sides. A joint-EM learning
technique is employed to optimize two TMs cooper-
atively. The resulting framework enables two models
to jointly boost each other’s translation performance.
Translation probabilities associated with each model
are used to compute weights that estimate the transla-
tion accuracy and punish the low-quality translations.
As a future work, we are interested in extend-
ing the present method to jointly learn multiple NMT
systems for several languages employing massive
amount of monolingual datasets.
ACKNOWLEDGEMENTS
We thank the anonymous reviewers for their valu-
able feedback and discussions. We also would like to
acknowledge the financial support received from the
Linguistics Department at UC Davis (USA).
REFERENCES
Ahmadnia, B. and Dorr, B. J. (2019). Augmenting neu-
ral machine translation through round-trip training ap-
proach. Open Computer Science, 9(1):268–278.
Ahmadnia, B., Kordjamshidi, P., and Haffari, G. (2018).
Neural machine translation advised by statistical ma-
chine translation: The case of farsi-spanish bilin-
gually low-resource scenario. In Proceedings of the
2018 17th IEEE International Conference on Machine
Learning and Applications (ICMLA), pages 1209–
1213.
Ahmadnia, B., Serrano, J., and Haffari, G. (2017). Persian-
Spanish low-resource statistical machine translation
through english as pivot language. In Proceedings
of Recent Advances in Natural Language Processing,
pages 24–30.
Artetxe, M., Labaka, G., and Agirre, E. (2019). An effec-
tive approach to unsupervised machine translation. In
Proceedings of the 57th Annual Meeting of the Associ-
ation for Computational Linguistics, pages 194–203.
Bahdanau, D., Cho, K., and Bengio, Y. (2015). Neural ma-
chine translation by jointly learning to align and trans-
late. In Proceedings of the International Conference
on Learning Representations.
Cheng, Y., Xu, W., He, Z., He, W., Wu, H., Sun, M., and
Liu, Y. (2016). Semi-supervised learning for neu-
ral machine translation. In Proceedings of the 54th
Annual Meeting of the Association for Computational
Linguistics, pages 1965–1974.
Chiang, D. (2007). Hierarchical phrase-based translation.
Computational Linguistics, 33(2):201–228.
Cho, K., merrienboer, B. V., G
¨
ulc¸ehre, C¸ ., Bahdanau, D.,
Bougares, F., Schwenk, H., and Bengio, Y. (2014).
Learning phrase representations using rnn encoder-
decoder for statistical machine translation. In Pro-
ceedings of the conference on Empirical Methods in
Natural Language Processing, pages 1724–1734.
Currey, A., Barone, M., and andK. Heafield, A. V. (2017).
Copied monolingual data improves low-resource neu-
ral machine translation. In Proceedings of the Second
Conference on Machine Translation, pages 148–156.
Dorr, B. J. (1994). Machine translation divergences: A
formal description and proposed solution. Computa-
tional Linguistics, 20(4):597–633.
Dorr, B. J., Pearl, L., Hwa, R., and Habash, N. (2002).
Duster: A method for unraveling cross-language di-
vergences for statistical word-level alignment. In Pro-
ceedings of the 5th conference of the Association for
Machine Translation in the Americas.
G
¨
ulc¸ehre, C¸ ., Firat, O., Xu, K., Cho, K., Barrault, L.,
Lin, H., Bougares, F., Schwenk, H., and Bengio, Y.
(2015). On using monolingual corpora in neural ma-
chine translation. ArXiv, abs/1503.03535.
He, D., Xia, Y., Qin, T., Wang, L., Yu, N., Liu, T., and Ma,
W. (2016). Dual learning for machine translation. In
Proceedings of the 30th Conference on Neural Infor-
mation Processing Systems.
Kiros, R., Zhu, Y., Salakhutdinov, R. R., Zemel, R., Ur-
tasun, R., Torralba, A., and Fidler, S. (2015). Skip-
thought vectors. In Proceedings of the 29th Confer-
ence on Advances in Neural Information Processing
Systems, pages 3294–3302.
Koehn, P., Och, F. J., and Marcu, D. (2003). Statistical
phrase-based translation. In Proceedings of the Con-
ference of the North American Chapter of the Associ-
ation for Computational Linguistics on Human Lan-
guage Technology, pages 48–54.
Lison, P. and Tiedemann, J. (2016). Opensubtitles2016: Ex-
tracting large parallel corpora from movie and tv sub-
titles. In Proceedings of the 10th edition of the Lan-
guage Resources and Evaluation Conference.
Luong, T., Sutskever, I., Le, Q., Vinyals, O., and Zaremba,
W. (2015). Addressing the rare word problem in neu-
ral machine translation. In Proceedings of the 53rd
Annual Meeting of the Association for Computational
Linguistics and the 7th International Joint Conference
on Natural Language Processing, pages 11–19.
Papineni, K., Roukos, S., Ward, T., and Zhu, W. (2001).
Bleu: A method for automatic evaluation of ma-
chine translation. In Proceedings of the 40th Annual
Meeting on Association for Computational Linguis-
tics, pages 311–318.
Ramachandran, P., Liu, P., and Le, Q. (2017). Unsupervised
pretraining for sequence to sequence learning. In Pro-
ceedings of the Conference on Empirical Methods in
Natural Language Processing, pages 383–391.
Robbins, H. and Monro, S. (1951). A stochastic approx-
imation method. Annals of Mathematical Statistics,
22:400–407.
NLPinAI 2021 - Special Session on Natural Language Processing in Artificial Intelligence
480