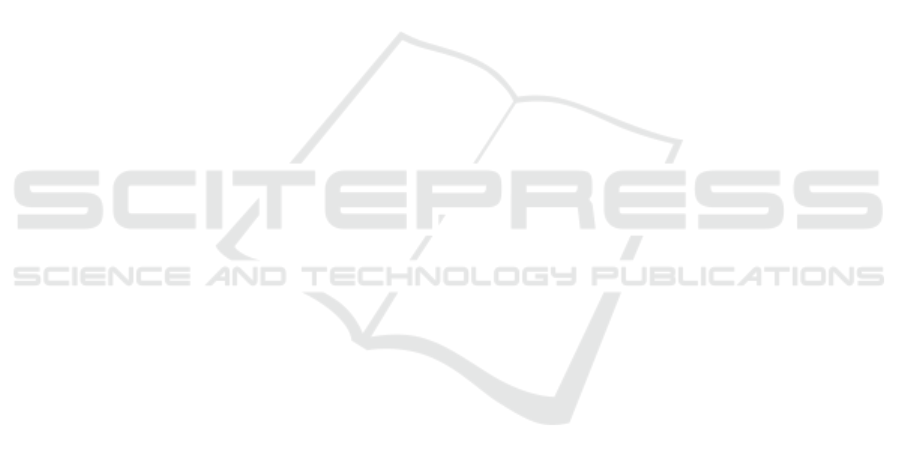
MM 2019 - Proceedings of the 27th ACM Interna-
tional Conference on Multimedia, pages 2687–2691.
Kang, P., Lin, Z., Teng, S., Zhang, G., Guo, L., and Zhang,
W. (2019). Catboost-based framework with additional
user information for social media popularity predic-
tion. MM 2019 - Proceedings of the 27th ACM In-
ternational Conference on Multimedia, pages 2677–
2681.
Ke, G., Meng, Q., Finley, T., Wang, T., Chen, W., Ma,
W., Ye, Q., and Liu, T. Y. (2017). LightGBM: A
highly efficient gradient boosting decision tree. Ad-
vances in Neural Information Processing Systems,
2017-Decem(Nips):3147–3155.
Khosla, A., Das Sarma, A., and Hamid, R. (2014). What
makes an image popular? In Proceedings of the 23rd
international conference on World wide web - WWW
’14, pages 867–876, New York, New York, USA.
ACM Press.
Kowalczyk, D. K. and Hansen, L. K. (2020). The complex-
ity of social media response: Statistical evidence for
one-dimensional engagement signal in twitter. In 12th
International Conference on Agents and Artificial In-
telligence, pages 918–925. SciTePress.
Kowalczyk, D. K. and Larsen, J. (2019). Scalable privacy-
compliant virality prediction on twitter. In AAAI-19
Workshop On Affective Content Analysi & CL-AFF
Happiness Shared Task, pages 12–27. CEUR-WS.
Larsson, A. O. (2019). Skiing all the way to the polls: Ex-
ploring the popularity of personalized posts on politi-
cal Instagram accounts. Convergence, 25(5-6):1096–
1110.
Lin, T., Maire, M., Belongie, S. J., Bourdev, L. D., Girshick,
R. B., Hays, J., Perona, P., Ramanan, D., Doll
´
ar, P.,
and Zitnick, C. L. (2014). Microsoft COCO: common
objects in context. CoRR, abs/1405.0312.
Lundberg, S. M. and Lee, S. I. (2017). A unified ap-
proach to interpreting model predictions. Advances
in Neural Information Processing Systems, 2017-
December(Section 2):4766–4775.
Mazloom, M., Pappi, I., and Worring, M. (2018). Cate-
gory Specific Post Popularity Prediction. In MultiMe-
dia Modeling, volume 10704 LNCS, pages 594–607.
Springer International Publishing.
Mazloom, M., Rietveld, R., Rudinac, S., Worring, M., and
Van Dolen, W. (2016). Multimodal popularity predic-
tion of brand-related social media posts. MM 2016
- Proceedings of the 2016 ACM Multimedia Confer-
ence, pages 197–201.
McParlane, P. J., Moshfeghi, Y., and Jose, J. M. (2014).
”Nobody comes here anymore, it’s too crowded”; pre-
dicting image popularity on Flickr. ICMR 2014 -
Proceedings of the ACM International Conference on
Multimedia Retrieval 2014, pages 385–391.
Mishra, S., Rizoiu, M. A., and Xie, L. (2016). Fea-
ture driven and point process approaches for popular-
ity prediction. International Conference on Informa-
tion and Knowledge Management, Proceedings, 24-
28-October-2016:1069–1078.
Ortis, A., Farinella, G. M., and Battiato, S. (2019). Predict-
ing Social Image Popularity Dynamics at Time Zero.
IEEE Access, 7:171691–171706.
Overgoor, G., Mazloom, M., Worring, M., Rietveld, R., and
Van Dolen, W. (2017). A spatio-temporal category
representation for brand popularity prediction. ICMR
2017 - Proceedings of the 2017 ACM International
Conference on Multimedia Retrieval, pages 233–241.
Patterson, G. and Hays, J. (2012). SUN attribute database:
Discovering, annotating, and recognizing scene at-
tributes. Proceedings of the IEEE Computer Society
Conference on Computer Vision and Pattern Recogni-
tion, pages 2751–2758.
Redmon, J. and Farhadi, A. (2018). YOLOv3: An Incre-
mental Improvement.
Rietveld, R., van Dolen, W., Mazloom, M., and Worring, M.
(2020). What You Feel, Is What You Like Influence
of Message Appeals on Customer Engagement on In-
stagram. Journal of Interactive Marketing, 49:20–53.
Russakovsky, O., Deng, J., Su, H., Krause, J., Satheesh, S.,
Ma, S., Huang, Z., Karpathy, A., Khosla, A., Bern-
stein, M., Berg, A. C., and Fei-Fei, L. (2015). Ima-
geNet Large Scale Visual Recognition Challenge. In-
ternational Journal of Computer Vision, 115(3):211–
252.
Song, C., Qu, Z., Blumm, N., and Barab
´
asi, A.-L. (2010).
Limits of predictability in human mobility. Science,
327(5968):1018–1021.
Tan, M. and Le, Q. V. (2019). EfficientNet: Rethinking
Model Scaling for Convolutional Neural Networks.
Wang, K., Bansal, M., and Frahm, J. M. (2018). Retweet
wars: Tweet popularity prediction via dynamic mul-
timodal regression. Proceedings - 2018 IEEE Win-
ter Conference on Applications of Computer Vision,
WACV 2018, 2018-Janua:1842–1851.
Wang, R., Yang, F., and Haigh, M. M. (2017). Let me take
a selfie: Exploring the psychological effects of post-
ing and viewing selfies and groupies on social media.
Telematics and Informatics, 34(4):274–283.
Wu, B., Cheng, W. H., Zhang, Y., Huang, Q., Li, J., and
Mei, T. (2017). Sequential prediction of social me-
dia popularity with deep temporal context networks.
IJCAI International Joint Conference on Artificial In-
telligence, 0:3062–3068.
Wu, B., Cheng, W. H., Zhang, Y., and Mei, T. (2016). Time
matters: Multi-scale temporalization of social media
popularity. MM 2016 - Proceedings of the 2016 ACM
Multimedia Conference, pages 1336–1344.
Zhang, Y. and Jatowt, A. (2019). Image tweet popu-
larity prediction with convolutional neural network.
In Advances in Information Retrieval, volume 11437
LNCS, pages 803–809. Springer International Pub-
lishing.
Zhang, Z., Chen, T., Zhou, Z., Li, J., and Luo, J. (2018).
How to Become Instagram Famous: Post Popularity
Prediction with Dual-Attention. Proceedings - 2018
IEEE International Conference on Big Data, Big Data
2018, pages 2383–2392.
Zhou, B., Lapedriza, A., Khosla, A., Oliva, A., and Tor-
ralba, A. (2018). Places: A 10 Million Image
Database for Scene Recognition. IEEE Transac-
tions on Pattern Analysis and Machine Intelligence,
40(6):1452–1464.
Zhou, B., Lapedriza, A., Xiao, J., Torralba, A., and Oliva,
A. (2014). Learning Deep Features for Scene Recog-
nition using Places Database - Supplementary Materi-
als. NIPS’14 Proceedings of the 27th International
Conference on Neural Information Processing Sys-
tems, 1:487–495.
On the Limits to Multi-modal Popularity Prediction on Instagram: A New Robust, Efficient and Explainable Baseline
1209