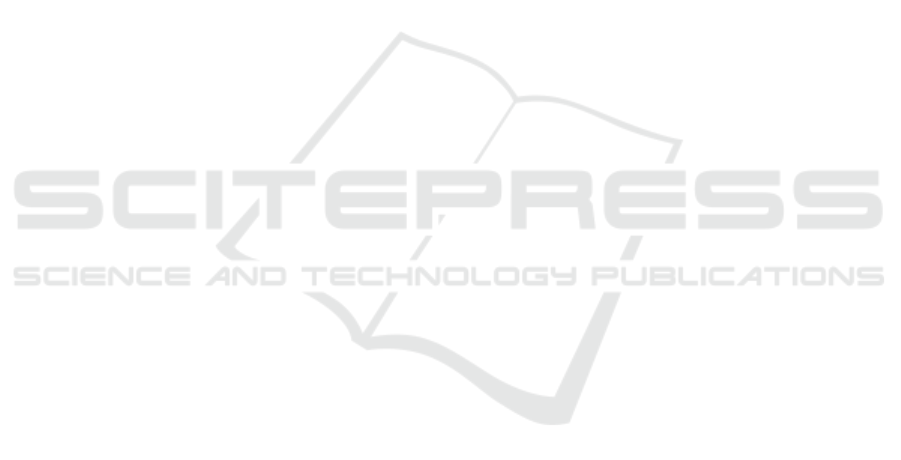
RFs and MLPs revealing themselves capable of pro-
ducing accurate forecasts. Furthermore, the used fea-
tures revealed themselves to have a significant impact
on the outcomes.
This research shows that it is possible to antici-
pate problematic situations and reduce their negative
impacts, with satisfactory accuracy. As future work,
the goal is to forecast the CO air concentration using
additional models, as well as distinct attributes. Ad-
ditionally, a major goal focuses on forecasting more
parameters related to Environmental Sustainability, in
order to promote a more sustainable and green soci-
ety.
ACKNOWLEDGEMENTS
This work was supported by National Funds through
the Portuguese funding agency, FCT - Founda-
tion for Science and Technology, within the project
DSAIPA/AI/0099/2019. The work of Bruno Fernan-
des is also supported by a Portuguese doctoral grant,
SFRH/BD/130125/2017, issued by FCT in Portugal.
REFERENCES
Block, M. L., Elder, A., Auten, R. L., Bilbo, S. D., Chen,
H., Chen, J. C., Cory-Slechta, D. A., Costa, D., Diaz-
Sanchez, D., Dorman, D. C., Gold, D. R., Gray, K.,
Jeng, H. A., Kaufman, J. D., Kleinman, M. T., Kir-
shner, A., Lawler, C., Miller, D. S., Nadadur, S. S.,
Ritz, B., Semmens, E. O., Tonelli, L. H., Veronesi, B.,
Wright, R. O., and Wright, R. J. (2012). The outdoor
air pollution and brain health workshop. NeuroToxi-
cology, 33(5):972–984.
Burrows, W. (1997). Cart regression models for predict-
ing uv radiation at the ground in the presence of cloud
and other environmental factors. Journal of Applied
Meteorology, 36(5):531–544. cited By 48.
Castelli, M., Clemente, F., Popovi
ˇ
c, A., Silva, S., and Van-
neschi, L. (2020). A machine learning approach to
predict air quality in california. Complexity, 2020.
cited By 0.
Cohen, A. J., Brauer, M., Burnett, R., Anderson, H. R.,
Frostad, J., Estep, K., Balakrishnan, K., Brunekreef,
B., Dandona, L., Dandona, R., Feigin, V., Freedman,
G., Hubbell, B., Jobling, A., Kan, H., Knibbs, L., Liu,
Y., Martin, R., Morawska, L., Pope, C. A., Shin, H.,
Straif, K., Shaddick, G., Thomas, M., van Dingenen,
R., van Donkelaar, A., Vos, T., Murray, C. J., and
Forouzanfar, M. H. (2017). Estimates and 25-year
trends of the global burden of disease attributable to
ambient air pollution: an analysis of data from the
Global Burden of Diseases Study 2015. The Lancet,
389(10082):1907–1918.
Dairi, A., Cheng, T., Harrou, F., Sun, Y., and Leiknes, T.
(2019). Deep learning approach for sustainable wwtp
operation: A case study on data-driven influent condi-
tions monitoring. Sustainable Cities and Society, 50.
cited By 2.
Feng, R., Zheng, H.-J., Gao, H., Zhang, A.-R., Huang, C.,
Zhang, J.-X., Luo, K., and Fan, J.-R. (2019). Recur-
rent neural network and random forest for analysis and
accurate forecast of atmospheric pollutants: A case
study in hangzhou, china. Journal of Cleaner Produc-
tion, 231:1005–1015. cited By 20.
Gawdzik, J., Szelag, B., Bezak-Mazur, E., and Stoinska,
R. (2016). Application of selected nonlinear meth-
ods to forecast the amount of excess sludge. Rocznik
Ochrona Srodowiska, 18(2):695–708.
Harrou, F., Dairi, A., Sun, Y., and Senouci, M. (2018).
Wastewater treatment plant monitoring via a deep
learning approach. volume 2018-February, pages
1544–1548. cited By 2.
Hino, M., Benami, E., and Brooks, N. (2018). Machine
learning for environmental monitoring. Nature Sus-
tainability, 1(10):583–588.
Igoe, D., Parisi, A., and Carter, B. (2013). Characterization
of a Smartphone Camera’s Response to Ultraviolet A
Radiation. International Journal of Remote Sensing,
pages 215–218.
Isabel Molina-G
´
omez, N., Rodr
´
ıguez-Rojas, K., Calder
´
on-
Rivera, D., Luis D
´
ıaz-Ar
´
evalo, J., and L
´
opez-Jim
´
enez,
P. A. (2020). Using machine learning tools to clas-
sify sustainability levels in the development of urban
ecosystems. Sustainability (Switzerland), 12(8).
Lee, K. K., Spath, N., Miller, M. R., Mills, N. L., and
Shah, A. S. (2020). Short-term exposure to carbon
monoxide and myocardial infarction: A systematic re-
view and meta-analysis. Environment International,
143(June):105901.
Norval, M., Lucas, R. M., Cullen, A., de Gruijl, F.,
Longstreth, J., Takizawa f, Y., and van der Leung, J.
(2011). The human health effects of ozone depletion
and interactions with climate change. Photochemical
and Photobiological Sciences, 10(2):173.
PAE (2013). https://www.apambiente.pt/. Accessed: 2020-
10-01.
Qiu, J., Wu, Q., Ding, G., Xu, Y., and Feng, S. (2016). A
survey of machine learning for big data processing.
Eurasip Journal on Advances in Signal Processing,
2016(1).
Sandryhaila, A. and Moura, J. M. (2014). Representa-
tion and processing of massive data sets with irregular
structure. IEEE SIGNAL PROCESSING MAGAZINE,
5(31):80–90.
Sarkodie, S. A. (2021). Environmental performance, bio-
capacity, carbon & ecological footprint of nations:
Drivers, trends and mitigation options. Science of the
Total Environment, 751:141912.
Spellman, F. R. (2013). Handbook of Water and Wastewater
Treatment Plant Operations.
Using Machine Learning to Forecast Air and Water Quality
1217