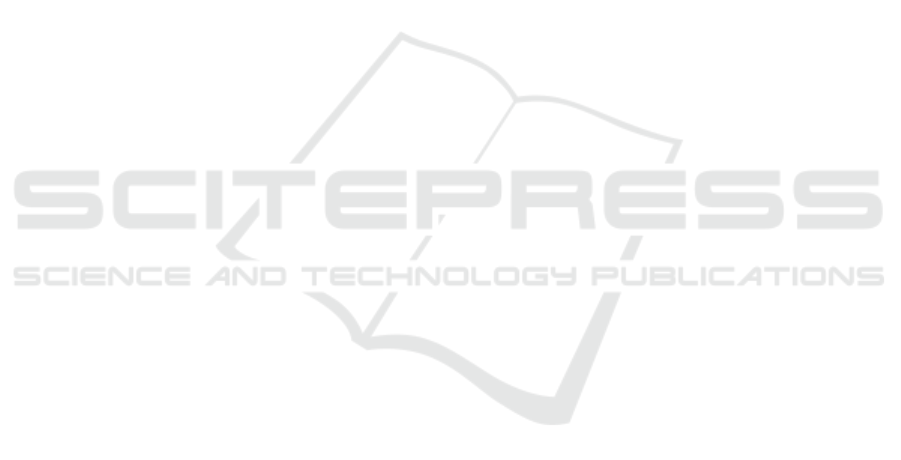
REFERENCES
Agarwal, A., Corvalan, A., Jensen, J., and Rambow, O.
(2012). Social network analysis of alice in wonder-
land. In Proceedings of the NAACL-HLT 2012 Work-
shop on computational linguistics for literature, pages
88–96.
Al Hasan, M., Chaoji, V., Salem, S., and Zaki, M. (2006).
Link prediction using supervised learning. In SDM06:
workshop on link analysis, counter-terrorism and se-
curity.
Balazevic, I., Allen, C., and Hospedales, T. M. (2018).
Hypernetwork knowledge graph embeddings. arXiv
preprint arXiv:1808.07018.
Becker, C., Rigamonti, R., Lepetit, V., and Fua, P. (2013).
Supervised feature learning for curvilinear structure
segmentation. In International conference on medical
image computing and computer-assisted intervention,
pages 526–533. Springer.
Berton, L., Valverde-Rebaza, J., and de Andrade Lopes,
A. (2015). Link prediction in graph construction for
supervised and semi-supervised learning. In 2015
International Joint Conference on Neural Networks
(IJCNN), pages 1–8. IEEE.
Burkov, A. (2019). The Hundred-Page Machine Learning
Book. Andriy Burkov.
Chu, Z., Widjaja, I., and Wang, H. (2012). Detecting social
spam campaigns on twitter. In International Confer-
ence on Applied Cryptography and Network Security,
pages 455–472. Springer.
Chuan, P. M., Giap, C. N., Bhatt, C., Khang, T. D., et al.
(2018). Enhance link prediction in online social net-
works using similarity metrics, sampling, and classifi-
cation. In Information Systems Design and Intelligent
Applications, pages 823–833. Springer.
Cohen, J., Dolan, B., Dunlap, M., Hellerstein, J. M., and
Welton, C. (2009). Mad skills: new analysis practices
for big data. Proceedings of the VLDB Endowment,
2(2):1481–1492.
Dettmers, T., Minervini, P., Stenetorp, P., and Riedel, S.
(2018). Convolutional 2d knowledge graph embed-
dings. In Thirty-Second AAAI Conference on Artificial
Intelligence.
Dwivedi, V. P., Joshi, C. K., Laurent, T., Bengio, Y., and
Bresson, X. (2020). Benchmarking graph neural net-
works. arXiv e-prints, pages arXiv–2003.
Goyal, P. and Ferrara, E. (2018). Graph embedding tech-
niques, applications, and performance: A survey.
Knowledge-Based Systems, 151:78–94.
Han, S. and Xu, Y. (2016). Link prediction in microblog
network using supervised learning with multiple fea-
tures. JCP, 11(1):72–82.
Kashima, H., Kato, T., Yamanishi, Y., Sugiyama, M., and
Tsuda, K. (2009). Link propagation: A fast semi-
supervised learning algorithm for link prediction. In
Proceedings of the 2009 SIAM international confer-
ence on data mining, pages 1100–1111. SIAM.
Kaur, R. and Singh, S. (2016). A survey of data mining
and social network analysis based anomaly detection
techniques. Egyptian informatics journal, 17(2):199–
216.
Kong, X., Chen, X., and Hovy, E. (2019). Decompressing
knowledge graph representations for link prediction.
arXiv preprint arXiv:1911.04053.
Kumar, A., Boehm, M., and Yang, J. (2017). Data man-
agement in machine learning: Challenges, techniques,
and systems. In Proceedings of the 2017 ACM Inter-
national Conference on Management of Data, pages
1717–1722. ACM.
Lee, J. B. and Adorna, H. (2012). Link prediction in a
modified heterogeneous bibliographic network. In
Proceedings of the 2012 International Conference on
Advances in Social Networks Analysis and Mining
(ASONAM 2012), pages 442–449. IEEE Computer
Society.
Martin, S., Brown, W. M., Klavans, R., and Boyack, K. W.
(2011). Openord: an open-source toolbox for large
graph layout. In Visualization and Data Analysis
2011, volume 7868, page 786806. International So-
ciety for Optics and Photonics.
Mutlu, E. C. and Oghaz, T. A. (2019). Review on graph
feature learning and feature extraction techniques for
link prediction. arXiv preprint arXiv:1901.03425.
Ngo, H. Q. and Nguyen, X. (2020). Learning models over
relational data: A brief tutorial. In Scalable Uncer-
tainty Management: 13th International Conference,
SUM 2019, Compi
`
egne, France, December 16–18,
2019, Proceedings, volume 11940, page 423. Springer
Nature.
Pinter, Y. and Eisenstein, J. (2018). Predicting semantic
relations using global graph properties. arXiv preprint
arXiv:1808.08644.
Shoham, Y., Perrault, R., Brynjolfsson, E., Clark, J.,
Manyika, J., Niebles, J. C., Lyons, T., Etchemendy,
J., and Bauer, Z. (2018). The ai index 2018 an-
nual report. AI Index Steering Committee, Human-
Centered AI Initiative, Stanford University. Available
at http://cdn. aiindex. org/2018/AI% 20Index, 202018.
Sinha, A., Shen, Z., Song, Y., Ma, H., Eide, D., Hsu, B.-j. P.,
and Wang, K. (2015). An overview of microsoft aca-
demic service (mas) and applications. In Proceedings
of the 24th international conference on world wide
web, pages 243–246. ACM.
Tang, J., Chang, Y., Aggarwal, C., and Liu, H. (2016).
A survey of signed network mining in social media.
ACM Computing Surveys (CSUR), 49(3):42.
Vartak, S. (2008). A survey on link prediction. State Uni-
versity of New York.
Vashishth, S., Sanyal, S., Nitin, V., Agrawal, N.,
and Talukdar, P. (2019). Interacte: Improv-
ing convolution-based knowledge graph embeddings
by increasing feature interactions. arXiv preprint
arXiv:1911.00219.
Wang, D., Cui, P., and Zhu, W. (2016). Structural deep net-
work embedding. In Proceedings of the 22nd ACM
SIGKDD international conference on Knowledge dis-
covery and data mining, pages 1225–1234. ACM.
Xu, C. and Li, R. (2019). Relation embedding with di-
hedral group in knowledge graph. arXiv preprint
arXiv:1906.00687.
ICEIS 2021 - 23rd International Conference on Enterprise Information Systems
166