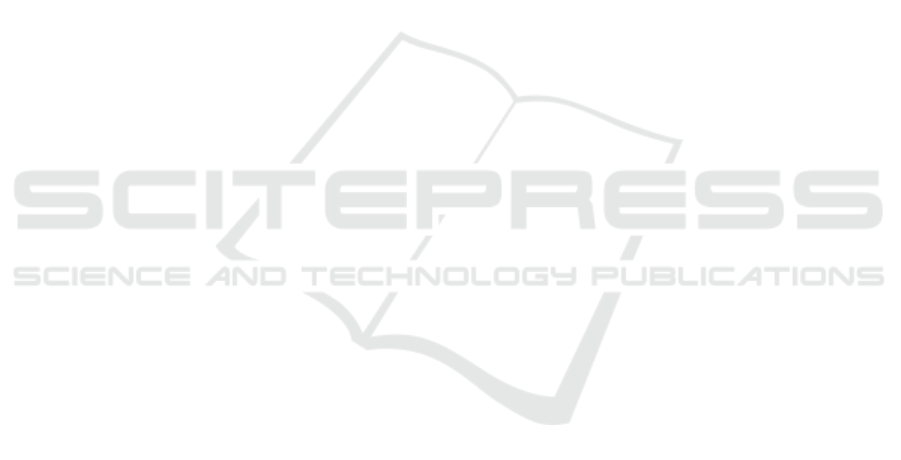
International Graduate School, Tsinghua Univer-
sity. And this work was supported by National
Natural Science Foundation of China (No.61803221
and No.U1813216) and the Basic Research Pro-
gram of Shenzhen (JCYJ20160301100921349,
JCYJ20170817152701660). We thank our partners
who provided helpful feedback and suggestions,
in particular Jian Ruan, Sicheng Liu, Anshun Xue,
Kangkang Dong, and Xiaojun Zhu.
REFERENCES
Aldoma, A., Tombari, F., Stefano, L. D., and Vincze, M.
(2012). A global hypotheses verification method for
3d object recognition. In Proceedings of the Euro-
pean Conference on Computer Vision (ECCV), vol-
ume 7574, page 511524.
Aoki, Y., Goforth, H., Srivatsan, R. A., and Lucey, S.
(2019). Pointnetlk: Robust efficient point cloud regis-
tration using pointnet. In IEEE Conference on Com-
puter Vision and Pattern Recognition (CVPR), page
71567165.
Besl, P. J. and McKay, N. D. (1992). A method for registra-
tion of 3-d shapes. IEEE Trans. Pattern Anal. Mach.
Intell., 14:239256.
Brachmann, E., Krull, A., Michel, F., Gumhold, S., Shotton,
J., and Rother, C. (2014). Learning 6d object pose
estimation using 3d object coordinates.
Brachmann, E., Michel, F., Krull, A., Yang, M. Y.,
Gumhold, S., and Rother, C. (2016). Uncertainty-
driven 6d pose estimation of ob- jects and scenes from
a single rgb image.
Bregier, R., Devernay, F., Leyrit, L., and Crowley, J. L.
(2018). Defining the pose of any 3d rigid object and
an associated distance. In Int. J. Comput. Vis., volume
126, page 571596.
Chen, S. H., Tian, D., Feng, C., Vetro, A., and Kovacevic,
J. (2018). Fast resampling of three-dimensional point
clouds via graphs. In Ieee Trans. Signal Process., vol-
ume 66, page 666681.
Deng, J., Dong, W., Socher, R., Li, L.-J., Li, K., and Fei-Fei,
L. (2010). Model globally, match locally: Efficient
and robust 3d object recognition. In 2010 IEEE Con-
ference on Computer Vision and Pattern Recognition,
pages 998–1005. IEEE.
Dovrat, O., Lang, I., and Avidan, S. (2019). Learning to
sample. Proceedings of the IEEE Conference on Com-
puter Vision and Pattern Recognition (CVPR), pages
2760–2769.
et al., S. H. (2012). Technical demonstration on model
based training, detection and pose estimation of
texture-less 3d objects in heavily cluttered scenes. In
Proceedings of the European Conference on Com-
puter Vision (ECCV), pages 593–596. IEEE.
Gall, J. and Lempitsky, V. (2009). Class-specific hough
forests for object detection. In IEEE Conference on
Computer Vision and Pattern Recognition (CVPR),
pages 1022–+.
Hinterstoisser, S., Lepetit, V., Rajkumar, N., and Konolige,
K. (2016). Going further with point pair features.
In Proceedings of the European Conference on Com-
puter Vision (ECCV), volume 9907, pages 834–848.
J. Sock, K. I. Kim, C. S. and Kim, T.-K. (2018). Multi-task
deepnetworks for depth-based 6d object pose and joint
registration in crowd scenarios. The British Machine
Vision Conference.
Michel, F., Kirillov, A., Brachmann, E., Krull, A.,
Gumhold, S., Savchynskyy, B., and Rother, C. (2017).
Global hypothesis generation for 6d object pose esti-
mation.
Moenning, C. and Dodgson, N. (2003). A new point cloud
simplification algorithm. In Proc. Int. Conf. Vis. Imag-
ing, page 810.
Qi, C. R., Litany, O., Kaiming, H., and Guibas, L. (2019).
Deep hough voting for 3d object detection in point
clouds. In IEEE International Conference on Com-
puter Vision (ICCV), page 92769285.
Qi, C. R., Su, H., Mo, K., and Guibas, L. J. (2017a). Point-
net: Deep learning on point sets for 3d classification
and segmentation. In Proceedings of the IEEE Con-
ference on Computer Vision and Pattern Recognition,
pages 652–660.
Qi, C. R., Yi, L., Su, H., and Guibas, L. J. (2017b). Point-
net++: Deep hierarchical feature learning on point sets
in a metric space. In Advances in neural information
processing systems, pages 5099–5108.
R. B. Rusu, N. B. and Beetz, M. (2009). Fast point feature
histograms (fpfh) for 3d registration. In Ieee Interna-
tional Conference on Robotics and Automation, page
18481853.
Xiang, Y., Schmidt, T., Narayanan, V., and Fox, D. (2018).
Posecnn: A convolutional neural network for 6d ob-
ject pose estimation in cluttered scenes.
Z, D., ann Zhou. T, L. S., H, C., L, Z., YuXing.Y, and
Liu.HD (2019). Ppr-net: Point-wise pose regression
network for instance segmentation and 6d pose es-
timation in bin-picking scenarios. In International
Conference on Intelligent Robots and Systems (IROS),
pages 1773–1780. IEEE.
Zhang, D. J., He, F. Z., Tu, Z. G., Zou, L., and Chen, Y. L.
(2020). Pointwise geometric and semantic learning
network on 3d point clouds. In Integr. Comput. Aided.
Eng., volume 27, page 5775.
ICAART 2021 - 13th International Conference on Agents and Artificial Intelligence
1240