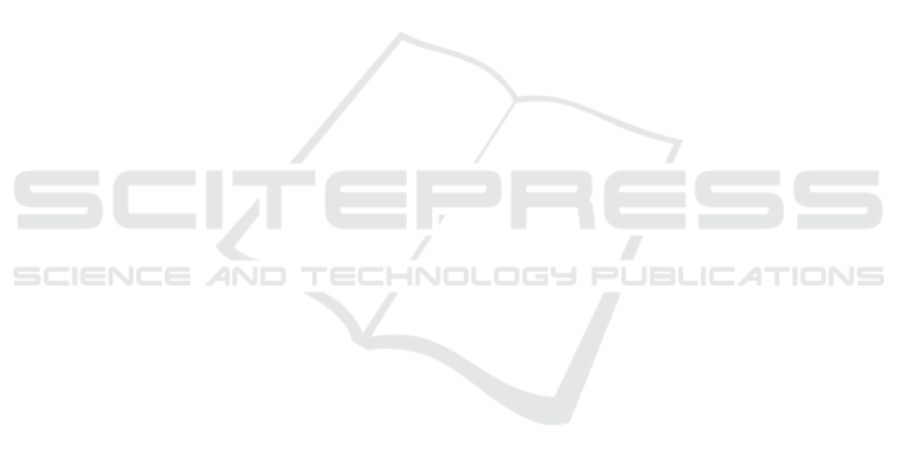
noise within the received UWB signal. For this pur-
pose, features are extracted using the DWT. These
descriptors are used as inputs of the LSTM network
which is exploited as a classifier. According to the
extensive experiments carried out on a large dataset.
Promising results are found, which outperform the
performances of the related techniques. For future
work, the employment of CNN to extract features
from the UWB signals can be investigated.
REFERENCES
(2020). Umain. http://www.umain.co.kr/en/uwb-radar-
sensor-module/.
Ahmed, S. and Cho, S. H. (2020). Hand gesture recognition
using an ir-uwb radar with an inception module-based
classifier. Sensors, 20(2):564.
Ameur, S., Khalifa, A. B., and Bouhlel, M. S. (2020). A
novel hybrid bidirectional unidirectional lstm network
for dynamic hand gesture recognition with leap mo-
tion. Entertainment Computing, 35:100373.
Blake, S. (1988). Os-cfar theory for multiple targets and
nonuniform clutter. IEEE transactions on aerospace
and electronic systems, 24(6):785–790.
Bock, S., Goppold, J., and Weiß, M. (2018). An improve-
ment of the convergence proof of the adam-optimizer.
arXiv preprint arXiv:1804.10587.
Briso, C., Calvo, C., and Xu, Y. (2019). Uwb propagation
measurements and modelling in large indoor environ-
ments. IEEE Access, 7:41913–41920.
Cai, L., Ma, X., Yan, S., Hao, C., and Wang, R. (2010).
Some analysis of fuzzy cago/so cfar detector in non-
gaussian background. In 2010 2nd International
Workshop on Intelligent Systems and Applications,
pages 1–4. IEEE.
Chen, S., Fan, C., Huang, X., and Cao, C. (2019). Low
prf low frequency radar sensor for fall detection by
using deep learning. In 2019 IEEE 4th International
Conference on Signal and Image Processing (ICSIP),
pages 400–404. IEEE.
Choi, J. W., Yim, D. H., and Cho, S. H. (2017). People
counting based on an ir-uwb radar sensor. IEEE Sen-
sors Journal, 17(17):5717–5727.
Conte, E., Longo, M., and Lops, M. (1988). Performance
analysis of ca-cfar in the presence of compound gaus-
sian clutter. Electronics Letters, 24(13):782–783.
Hakobyan, G. and Yang, B. (2019). High-performance au-
tomotive radar: A review of signal processing algo-
rithms and modulation schemes. IEEE Signal Pro-
cessing Magazine, 36(5):32–44.
Hochreiter, S. and Schmidhuber, J. (1997). Long short-term
memory. Neural computation, 9(8):1735–1780.
Jegham, I., Khalifa, A. B., Alouani, I., and Mahjoub, M. A.
(2018). Safe driving: driver action recognition using
surf keypoints. In 2018 30th International Conference
on Microelectronics (ICM), pages 60–63. IEEE.
Jiang, C., Shen, J., Chen, S., Chen, Y., Liu, D., and
Bo, Y. (2020). Uwb nlos/los classification using
deep learning method. IEEE Communications Letters,
24(10):2226–2230.
Khalifa, A. B., Alouani, I., Mahjoub, M. A., and Rivenq,
A. (2020). A novel multi-view pedestrian detec-
tion database for collaborative intelligent transporta-
tion systems. Future Generation Computer Systems,
113:506–527.
Kim, D.-H. (2020). Lane detection method with impulse
radio ultra-wideband radar and metal lane reflectors.
Sensors, 20(1):324.
Ko, S.-K. and Lee, B.-T. (2016). Object classification of
uwb responses using s t-cnn. In 2016 International
Conference on Information and Communication Tech-
nology Convergence (ICTC), pages 794–796. IEEE.
Koci
´
c, J., Jovi
ˇ
ci
´
c, N., and Drndarevi
´
c, V. (2018). Sensors
and sensor fusion in autonomous vehicles. In 2018
26th Telecommunications Forum (TELFOR), pages
420–425.
Kumar, D., Sarkar, A., Kerketta, S. R., and Ghosh, D.
(2019). Human activity classification based on breath-
ing patterns using ir-uwb radar. In 2019 IEEE 16th
India Council International Conference (INDICON),
pages 1–4.
Lee, D., Shaker, G., and Melek, W. (2020). Imaging of hu-
man walking behind the obstacle utilizing pulsed radar
technique in the c-band for military surveillance appli-
cations. JOURNAL OF ELECTRICAL ENGINEER-
ING & TECHNOLOGY, 15(3):1431–1439.
Lim, S., Jung, J., Kim, S.-C., and Lee, S. (2020). Deep neu-
ral network-based in-vehicle people localization using
ultra-wideband radar. IEEE Access.
Maitre, J., Bouchard, K., Bertuglia, C., and Gaboury, S.
(2021). Recognizing activities of daily living from
uwb radars and deep learning. Expert Systems with
Applications, 164:113994.
Mendel, J. M. (1991). Tutorial on higher-order statistics
(spectra) in signal processing and system theory: the-
oretical results and some applications. Proceedings of
the IEEE, 79(3):278–305.
Mimouna, A., Alouani, I., Ben Khalifa, A., El Hillali,
Y., Taleb-Ahmed, A., Menhaj, A., Ouahabi, A., and
Ben Amara, N. E. (2020). Olimp: A heterogeneous
multimodal dataset for advanced environment percep-
tion. Electronics, 9(4):560.
Nguyen, V.-H. and Pyun, J.-Y. (2015). Location detection
and tracking of moving targets by a 2d ir-uwb radar
system. Sensors, 15(3):6740–6762.
Piriyajitakonkij, M., Warin, P., Lakhan, P., Leelaarporn, P.,
Kumchaiseemak, N., Suwajanakorn, S., Pianpanit, T.,
Niparnan, N., Mukhopadhyay, S. C., and Wilaiprasit-
porn, T. (2020). Sleepposenet: Multi-view learning
for sleep postural transition recognition using uwb.
IEEE Journal of Biomedical and Health Informatics.
Poulose, A. and Han, D. S. (2020). Uwb indoor localization
using deep learning lstm networks. Applied Sciences,
10(18):6290.
Quan, X., Choi, J. W., and Cho, S. H. (2020). A new thresh-
ICAART 2021 - 13th International Conference on Agents and Artificial Intelligence
424