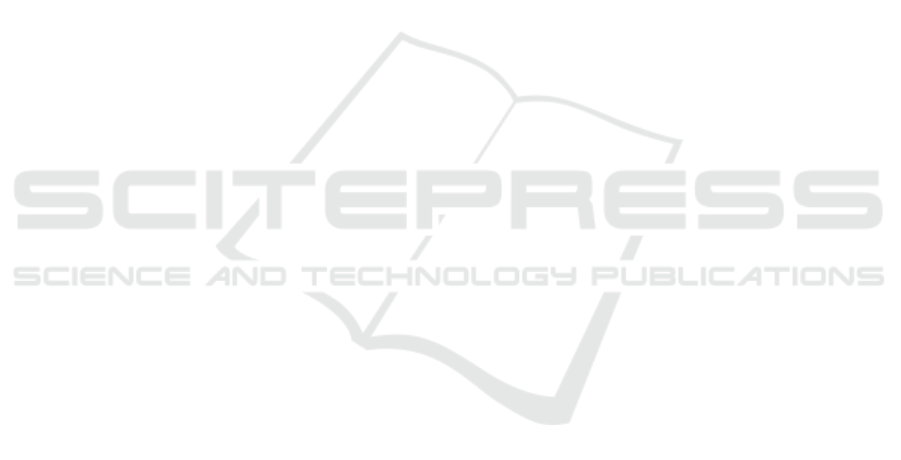
Making automatic speech recognition available
for Oral Historians and other Humanities scholars in
a research infrastructure setting (bringing the algo-
rithms to the data) to foster the analysis of audiovisual
resources, requires a deliberate technical design given
the above mentioned requirements. The design of the
DANE environment discussed in section 2.2 warrants
measures to adapt to these requirements: secure ac-
cess to and processing of audiovisual sources of in-
stitutional collections in bulk, efficient use of dedi-
cated machinery (e.g., local or cloud-based computer
clusters), dealing with dynamics with respect to ro-
bustness, latency, process management, and storage
of intermediate data, the implementation of adaptive
workflows to address the OOV problem, and keeping
track of provenance information (which version of a
speech recognition system was used).
4 CONCLUSION
In this article we have argued that there is a gap be-
tween what is possible in terms of Audio Visual Pro-
cessing and what is available to DH scholars, and that
to bridge the gap between these fields it is necessary
to make available established tools. On the one hand,
this will enable DH scholars to incorporate AVP ap-
proaches and technologies in their research, to gain a
fuller, more ’macroscopic’ perspective on audiovisual
media (Graham et al., 2015); on the other, the seman-
tically complex analyses of DH scholars can be used
as input to boost the development of semantically sen-
sitive AVP algorithms.
While a major step in this process is to make
AVP tools practically available, it is equally neces-
sary to create an environment in which tools can be
developed or customised to support answering newly
emerging research questions. By integrating DANE,
our proposed environment for deploying AVP tools,
in the Media Suite virtual research environment we
have been able to bring the algorithms to the rich
catalogue of datasets that is available in the Media
Suite. Moreover, the distributed and modular design
of DANE ensures flexibility in deploying new tools,
as well as an easy and well-documented process for
converting algorithms to tools. In embracing such an
approach we have taken the first steps in developing
an AVP environment within CLARIAH that enables a
continuous cycle of automatically annotating and en-
riching AV archives, opening the door for further col-
laboration between AVP researchers and DH scholars.
ACKNOWLEDGEMENTS
The research described in this paper was made possi-
ble by the CLARIAH-PLUS project (www.clariah.nl)
financed by NWO.
REFERENCES
Arnold, T. and Tilton, L. (2019). Distant viewing: Analyz-
ing large visual corpora. Digital Scholarship in the
Humanities.
Babenko, A. and Lempitsky, V. (2016). Efficient Index-
ing of Billion-Scale Datasets of Deep Descriptors. In
2016 IEEE Conference on Computer Vision and Pat-
tern Recognition (CVPR), pages 2055–2063, Las Ve-
gas, NV, USA. IEEE.
Barras, C., Geoffrois, E., Wu, Z., and Liberman, M. (2001).
Transcriber: development and use of a tool for assist-
ing speech corpora production. Speech Communica-
tion, 33(1-2):5–22.
Bhargav, S., van Noord, N., and Kamps, J. (2019). Deep
learning as a tool for early cinema analysis. In Pro-
ceedings of the 1st Workshop on Structuring and Un-
derstanding of Multimedia heritAge Contents, pages
61–68.
Blanke, T. and Hedges, M. (2013). Scholarly primi-
tives: Building institutional infrastructure for human-
ities e-science. Future Generation Computer Systems,
29(2):654–661.
de Jong, F. M. G., Oard, D. W., Heeren, W. F. L., and Ordel-
man, R. J. F. (2008). Access to recorded interviews:
A research agenda. ACM Journal on Computing and
Cultural Heritage (JOCCH), 1(1):3:1–3:27.
D
¨
ork, M., Carpendale, S., and Williamson, C. (2011). The
information flaneur: A fresh look at information seek-
ing. In Proceedings of the SIGCHI Conference on Hu-
man Factors in Computing Systems, CHI ’11, pages
1215–1224, New York, NY, USA. Association for
Computing Machinery.
Garofolo, J. S., Auzanne, C. G. P., and Voorhees, E. M.
(2000). The trec spoken document retrieval track: A
success story. In Content-Based Multimedia Informa-
tion Access - Volume 1, RIAO ’00, pages 1–20, Paris,
France, France. Le Centre De Hautes Etudes Interna-
tionals D’Informatique Documentaire.
Goldman, J., Renals, S., Bird, S., De Jong, F., Federico,
M., Fleischhauer, C., Kornbluh, M., Lamel, L., Oard,
D. W., Stewart, C., et al. (2005). Accessing the spo-
ken word. International Journal on Digital Libraries,
5(4):287–298.
Graham, S., Milligan, I., and Weingart, S. (2015). Explor-
ing Big Historical Data: The Historian’s Macroscope.
World Scientific Publishing Company.
Gustman, S., Soergel, D., Oard, D., Byrne, W., Picheny, M.,
Ramabhadran, B., and Greenberg, D. (2002). Sup-
porting access to large digital oral history archives. In
Proceedings of the second ACM/IEEE-CS joint con-
ference on Digital libraries - JCDL ’02, page 18, New
York, New York, USA. ACM Press.
Automatic Annotations and Enrichments for Audiovisual Archives
639