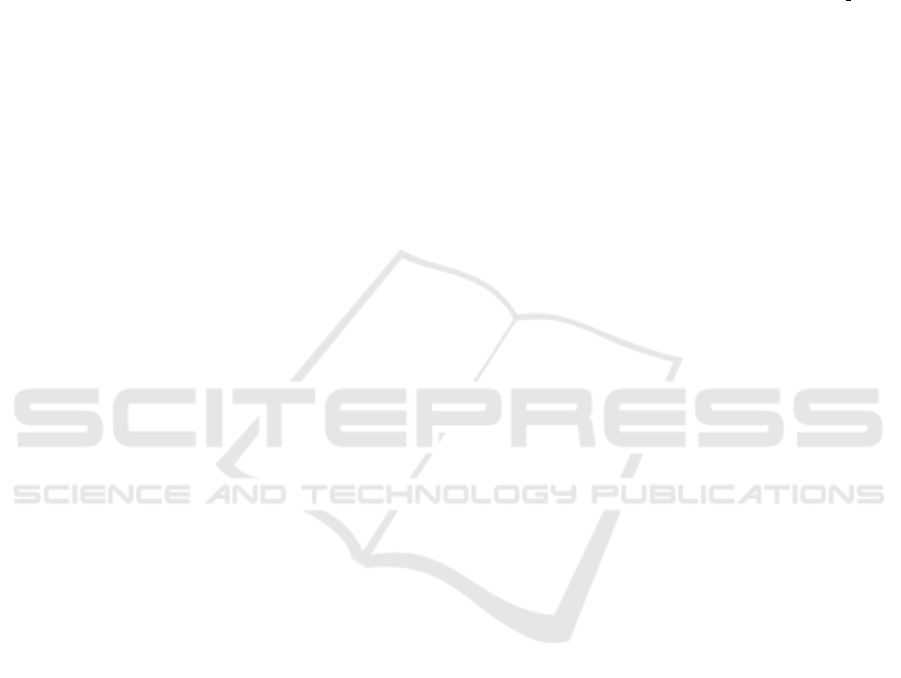
4 DISCUSSION
In this paper, we explored SFA for the extraction
of generative latent factors. We developed different
models and evaluated them on a variety of datasets
with different data-generating attributes. In this eval-
uation, we found that the extraction principle of slow-
ness is in general not contrary to reconstructability
from low-dimensional representations, while provid-
ing the corresponding space with additional proper-
ties beneficial for generative tasks as has been demon-
strated in Section 3.4. However, while slow features
live in a structured, continuous and complete space,
the specific nature of the extracted features is gov-
erned by the types of latent variables used in data gen-
eration and can negatively impact the overall quality
of the reconstruction in specific cases.
One of these cases is identified as the mixing of
continuous with categorical latent variables and is
subsequently addressed in Section 3.4.3 by develop-
ment of the What-Where Encode-Decoder model us-
ing two qualitatively different extraction paths.
Finally, to complete a possible generative model
based on SFA, a prior distribution had to be con-
structed. As construction of suitable prior distribu-
tions is in general a hard problem, the chosen ap-
proach leveraged known structural properties of SFA-
extracted features and was successfully applied for
the case of single samples of a synthetic dataset
when using a low-dimensional feature space in Sec-
tion 3.5.1. A possible ansatz to also generate se-
quences was discussed in Section 3.5.2.
Future Directions. We see potential in continued
investigation of SFA representations as foundation for
generative models, as it also has been shown to ex-
tract useful representations even in the case of high-
dimensional data. One limitation here might lie in the
use of very low-dimensional latent spaces: While ef-
fective prior distributions can be constructed, not all
interesting latent factors might be captured. There-
fore, the authors regard the possible generalization of
the identified construction principles to higher dimen-
sions as the most promising research direction at this
point.
REFERENCES
B
¨
ohmer, W., Gr
¨
unew
¨
alder, S., Nickisch, H., and Ober-
mayer, K. (2011). Regularized sparse kernel slow
feature analysis. In Gunopulos, D., Hofmann, T.,
Malerba, D., and Vazirgiannis, M., editors, Machine
Learning and Knowledge Discovery in Databases,
pages 235–248, Berlin, Heidelberg. Springer Berlin
Heidelberg.
Denton, E. and Fergus, R. (2018). Stochastic video gener-
ation with a learned prior. In Dy, J. and Krause, A.,
editors, Proceedings of the 35th International Confer-
ence on Machine Learning, volume 80 of Proceedings
of Machine Learning Research, pages 1174–1183,
Stockholmsm
¨
assan, Stockholm Sweden. PMLR.
Dozat, T. (2015). Incorporating nesterov momentum into
adam. http://cs229.stanford.edu/proj2015/054
report.
pdf.
Escalante-B, A. N. and Wiskott, L. (2020). Improved graph-
based sfa: Information preservation complements the
slowness principle. Machine Learning, 109(5):999–
1037.
Franzius, M., Sprekeler, H., and Wiskott, L. (2007). Slow-
ness and sparseness lead to place, head-direction,
and spatial-view cells. PLoS Computational Biology,
3(8):1–18.
Franzius, M., Wilbert, N., and Wiskott, L. (2011). Invariant
object recognition and pose estimation with slow fea-
ture analysis. Neural Computation, 23(9):2289–2323.
Goodfellow, I., Bengio, Y., and Courville, A. (2016). Deep
Learning. MIT Press. http://www.deeplearningbook.
org.
Goodfellow, I., Pouget-Abadie, J., Mirza, M., Xu, B.,
Warde-Farley, D., Ozair, S., Courville, A., and Ben-
gio, Y. (2014). Generative adversarial nets. In Ghahra-
mani, Z., Welling, M., Cortes, C., Lawrence, N. D.,
and Weinberger, K. Q., editors, Advances in Neu-
ral Information Processing Systems 27, pages 2672–
2680. Curran Associates, Inc.
Hafner, D., Lillicrap, T., Ba, J., and Norouzi, M. (2020).
Dream to control: Learning behaviors by latent imag-
ination. In International Conference on Learning Rep-
resentations.
Karras, T., Aila, T., Laine, S., and Lehtinen, J. (2018). Pro-
gressive growing of GANs for improved quality, sta-
bility, and variation. In International Conference on
Learning Representations.
Kingma, D. P. and Ba, J. (2014). Adam: A
method for stochastic optimization. arXiv preprint
arXiv:1412.6980.
Kingma, D. P., Salimans, T., Jozefowicz, R., Chen, X.,
Sutskever, I., and Welling, M. (2016). Improved vari-
ational inference with inverse autoregressive flow. In
Advances in neural information processing systems,
pages 4743–4751.
Kingma, D. P. and Welling, M. (2013). Auto-encoding vari-
ational bayes. arXiv preprint arXiv:1312.6114.
Kingma, D. P. and Welling, M. (2019). An introduction to
variational autoencoders. Foundations and Trends
R
in Machine Learning, 12(4):307–392.
LeCun, Y., Cortes, C., and Burges, C. (2010). Mnist hand-
written digit database. http://yann.lecun.com/exdb/
mnist.
Mehri, S., Kumar, K., Gulrajani, I., Kumar, R., Jain, S.,
Sotelo, J., Courville, A., and Bengio, Y. (2016). Sam-
plernn: An unconditional end-to-end neural audio
generation model. arXiv preprint arXiv:1612.07837.
ICPRAM 2021 - 10th International Conference on Pattern Recognition Applications and Methods
130