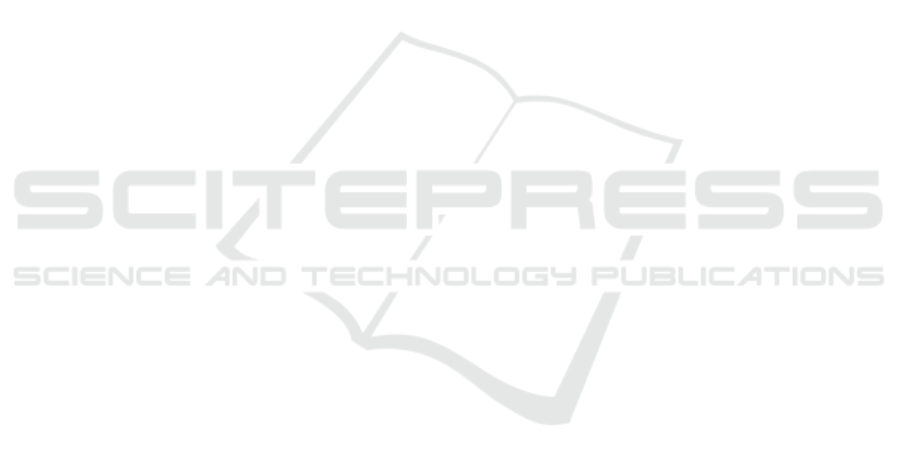
der Grant Nos. 61772210, 61806080, 62006085 and
U1911201; Guangdong Province Universities Pearl
River Scholar Funded Scheme (2018); Humanities
and Social Sciences Foundation of Ministry of Edu-
cation of China under Grant No. 18YJC72040002;
Doctoral Startup Project of Natural Science Founda-
tion of Guangdong Province of China under Grant
No. 2018A030310529; Project of Science and Tech-
nology in Guangzhou in China under Grant Nos.
201807010043 and 202007040006.
REFERENCES
Chebbah, M., Martin, A., and Yaghlane, B. B. (2015). Com-
bining partially independent belief functions. Deci-
sion Support Systems, 73:37–46.
Daniel, M. (2014). Conflict between belief functions: A
new measure based on their non-conflicting parts. In
Proceedings of the 3th International Conference on
Belief Functions: Theory and Applications, Lecture
Notes in Computer Science, volume 8764, pages 321–
330.
Dempster, A. (2008). Upper and lower probabilities in-
duced by a multivalued mapping. In Classic Works of
the Dempster-Shafer Theory of Belief Functions, vol-
ume 219 of Studies in Fuzziness and Soft Computing,
pages 57–72. Springer.
Deng, X., Deng, Y., and Chan, F. T. S. (2014). An improved
operator of combination with adapted conflict. Annals
of Operations Research, 223(1):451–459.
Deng, Y. (2015). Generalized evidence theory. Applied In-
telligence, 43(3):530–543.
Deng, Y., Shi, W., Zhu, Z., and Liu, Q. (2004). Combining
belief functions based on distance of evidence. Deci-
sion Support Systems, 38(3):489–493.
Destercke, S. and Burger, T. (2012). Toward an axiomatic
definition of conflict between belief functions. IEEE
Transactions on Systems, Man, and Cybernetics, Part
B: Cybernetics, 43(2):585–596.
Dubois, D. and Prade, H. (1988a). Default reasoning and
possibility theory. Artificial Intelligence, 35(2):243–
257.
Dubois, D. and Prade, H. (1988b). Representation and
combination of uncertainty with belief functions and
possibility measures. Computational Intelligence,
4(3):244–264.
Elouedi, Z. and Mercier, D. (2011). Towards an alarm for
opposition conflict in a conjunctive combination of
belief functions. In Proceedings on the 11th European
Conference on Symbolic and Quantitative Approaches
to Reasoning with Uncertainty, pages 314–325.
Hong, X., Huang, Y., Ma, W., Varadarajan, S., Miller, P. C.,
Liu, W., Romero, M. J. S., del Rinc
´
on, J. M., and
Zhou, H. (2016). Evidential event inference in trans-
port video surveillance. Computer Vision and Image
Understanding, 144:276–297.
Jiang, W. (2018). A correlation coefficient for belief func-
tions. International Journal of Approximate Reason-
ing, 103:94–106.
Jiang, W., Wei, B., Xie, C., and Zhou, D. (2016). An eviden-
tial sensor fusion method in fault diagnosis. Advances
in Mechanical Engineering, 8(3):1–7.
Jiang, W. and Zhan, J. (2017). A modified combination rule
in generalized evidence theory. Applied Intelligence,
46(3):630–640.
Jousselme, A.-L., Grenier, D., and Boss
´
e,
´
E. (2001). A new
distance between two bodies of evidence. Information
Fusion, 2(2):91–101.
Liu, W. (2006). Analyzing the degree of conflict among
belief functions. Artificial Intelligence, 170(11):909–
924.
Ma, W., Jiang, Y., and Luo, X. (2019). A flexible rule for
evidential combination in dempster-shafer theory of
evidence. Appled Soft Computing, 85:105512.
Ma, W., Luo, X., and Jiang, Y. (2017). Multi-criteria de-
cision making with cognitive limitations: A DS/AHP-
based approach. International Journal of Intelligent
Systems, 32(7):686–721.
Ma, W., Luo, X., and Liu, W. (2013). An ambiguity aver-
sion framework of security games under ambiguities.
In Proceedings of the 23rd International Joint Confer-
ence on Artificial Intelligence, pages 271–278.
Murphy, C. K. (2000). Combining belief functions when ev-
idence conflicts. Decision Support Systems, 29(1):1–
9.
Shafer, G. (1976). A mathematical theory of evidence, vol-
ume 1. Princeton University Press.
Smarandache, F. and Dezert, J. (2006). Advances and ap-
plications of DSmT for information fusion, volume 2.
American Research Press.
Smets, P. (2000). Data fusion in the transferable belief
model. In Proceedings of the 3rd International Con-
ference on Information Fusion, volume 1, pages 21–
33.
Smets, P. (2005). Decision making in the TBM: The ne-
cessity of the pignistic transformation. International
Journal of Approximate Reasoning, 38(2):133–147.
Smets, P. (2007). Analyzing the combination of conflicting
belief functions. Information Fusion, 8(4):387–412.
Wang, J., Xiao, F., Deng, X., Fei, L., and Deng, Y. (2016).
Weighted evidence combination based on distance of
evidence and entropy function. International Journal
of Distributed Sensor Networks, 12(7):3218784.
Yager, R. R. (1987). On the Dempster-Shafer framework
and new combination rules. Information Sciences,
41(2):93–137.
Zadeh, L. A. (1986). A simple view of the Dempster-Shafer
theory of evidence and its implication for the rule of
combination. AI Magazine, 7(2):85–90.
Zhan, J., Luo, X., Feng, C., and He, M. (2018). A
multi-demand negotiation model based on fuzzy rules
elicited via psychological experiments. Applied Soft
Computing, 67:840–864.
Zhao, Y., Jia, R., and Shi, P. (2016). A novel combination
method for conflicting evidence based on inconsistent
measurements. Information Sciences, 367-368:125–
142.
A Measurement for Essential Conflict in Dempster-Shafer Theory
1289