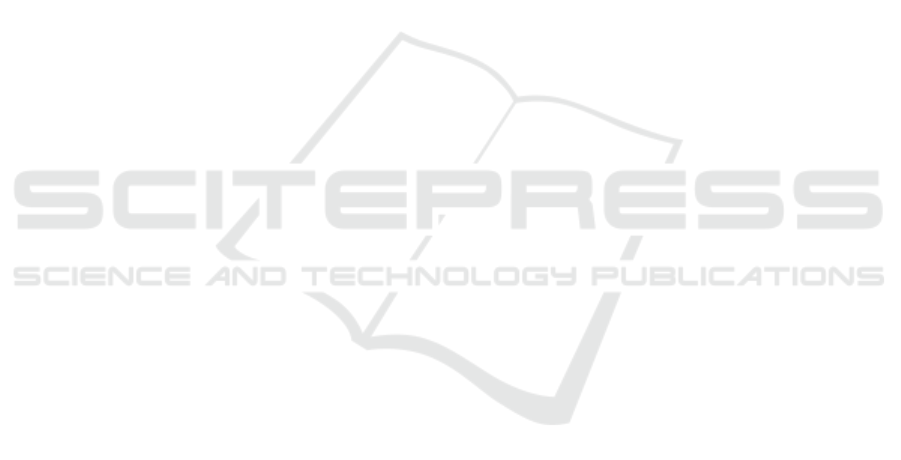
The content of this research refers to a generic
view to all modes of transports. However, this means
that the generation of new GPS data is not only based
on the existing car or bicycle routes. The generated
data can for example vary from roads or enter green
areas as well. So, for an outsider, it is still impossible
to distinguish between artificially generated data or
real data because all modes of transportation can be
combined for tracking the routes in the application.
Finally, the EXCL-Algorithm preserves data that
can be used for further studies and protects the pri-
vacy of each user who contributes by tracking the
daily trips. Further studies can focus to generate only
those GPS data points lying on roads for cars or pave-
ments for pedestrians depending on the provided data.
This studies would precise the EXCL-Algorithm and
its data generation and would focus on only one mode
of transportation.
ACKNOWLEDGEMENTS
This paper is based on the research and develop-
ment joint project NUMIC - New Urban Awareness
of Mobility in Chemnitz. It is founded by the Fed-
eral Ministry of Education and Research (BMBF) and
the European Social Fund under the grant number
01UR1804A. The responsibility for this publication
lies with the authors.
REFERENCES
Bundesministerium f
¨
ur Verkehr und digitale Infrastruk-
tur (2020). Mobilit
¨
at in Deutschland (MiD). ttps:
//www.bmvi.de/SaredDocs/DE/Artikel/G/ mobilitaet-
in-deutschland.html. [Online, accessed 2-November-
2020].
Chemnitz, Stadt der Moderne (2020). Mobilit
¨
atsverhalten
- Untersuchung “Mobilit
¨
at in St
¨
adten”. URL:
https://www.chemnitz.de/chemnitz/de/unsere-stadt/
verkehr/verkehrsplanung/mobilittsverhalten/index.
html. [Online, accessed 30-October-2020].
Chow, C.-Y. and Mokbel, M. F. (2011). Privacy of spatial
trajectories. In Computing with spatial trajectories,
pages 109–141. Springer.
Claudio, B., Sean Wang, X., and Jajodia, S. (2005). Pro-
tecting privacy against location-based personal iden-
tification. Jonker, Petkovi
´
c (Hg.) 2005 – Secure Data
Management, page 185–199.
Feng, T. and Timmermans, H. J. (2017). Using recurrent
spatio-temporal profiles in gps panel data for enhanc-
ing imputation of activity type. Big Data for Regional
Science, pages 121–130.
Ghinita, G., Kalnis, P., and Skiadopoulos, S. (2007). Pro-
ceedings of the 16th international conference on world
wide web. page 371.
G
´
eron, A. (2018). Machine Learning mit Scikit-Learn und
Tensorflow. O’Reilly, Heidelberg.
Hoh, B., Gruteser, M., Xiong, H., and Alrabady, A. (2007).
Preserving privacy in gps traces via uncertainty-aware
path cloaking. page 161.
Hua, Y., Zhao, Z., Li, R., Chen, X., Liu, Z., and Zhang,
H. (2019). Deep learning with long short-term mem-
ory for time series prediction. IEEE Communications
Magazine, 57(6):114–119.
Jeansoulin, R., Papini, O., Prade, H., and Schockaert, S.
(2010). Methods for handling imperfect spatial infor-
mation, volume 256. Springer.
Martens, K. (2007). Promoting bike-and-ride: The dutch
experience. Transportation Research Part A: Policy
and Practice, 41(4):326–338.
Merkel, D. A. (2018). Rede von bundeskanzlerin dr. angela
merkel beim ix. petersberger klimadialog am 19. juni
2018 in berlin. Bulletin, die Bundesregierung, 68.
Niu, B., Li, Q., Zhu, X., Cao, G., and Li, H. (2014). Achiev-
ing k-anonymity in privacy-aware location-based ser-
vices. IEEE INFOCOM 2014 2014, page 754–762.
Ortmeyer, T. H. and Pillay, P. (2001). Trends in transporta-
tion sector technology energy use and greenhouse gas
emissions. pages 1837–1847.
Pan, X., den Elzen, M., H
¨
ohne, N., Teng, F., and Wang,
L. (2017). Exploring fair and ambitious mitigation
contributions under the paris agreement. pages 49–56.
Ritchie, H. (2020). Cars, planes, trains: where
do CO2 emissions from transport come
from? URL: ttps://ourworldindata.org/
co2-emissions-from-transport.\newblock[Online,
accessed28-October-2020].\bibitem[Sceider et al.,
2020]ExtendTrack Scheider, S., Wang, J., Mol, M.,
Schmitz, O., and Karssenberg, D. (2020). Obfus-
cating spatial point tracks with simulated crowding.
International Journal of Geographical Information
Science, 34(7):1398–1427.
Seber, G. and Lee, A. (2012). Linear Regression Analysis.
Wiley Series in Probability and Statistics. Wiley.
Sumo (2020). Simulation of urban mobility. URL: eclipse.
org/sumo/. [Online, accessed 07-September-2020].
Sweeney, L. (2012). k-anonymity: A model for protecting
privacy: International journal of uncertainty, fuzziness
and knowledge-based systems, 10(05), 557-570.
Trujillo-Rasua, R. and Domingo-Ferrer, J. (2015). Privacy
in spatio-temporal databases: A microaggregation-
based approach. 567:197–214.
Wang, S. and Wang, X. S. (2010). In-device spatial cloaking
for mobile user privacy assisted by the cloud. pages
381–386.
Wardman, M., T. M. . P. M. (2007). Factors influencing the
propensity to cycle to work. Transportation Research
Part A: Policy and Practice, 41(4):339 – 350.
Zhu, X., Chi, H., Niu, B., Zhang, W., Li, Z., and Li, H.
(2013). Mobicache: When k-anonymity meets cache.
pages 820–825. IEEE.
Use of Machine Learning for Expanding Realistic and Usable Routes for Data Analysis on Sustainable Mobility
163