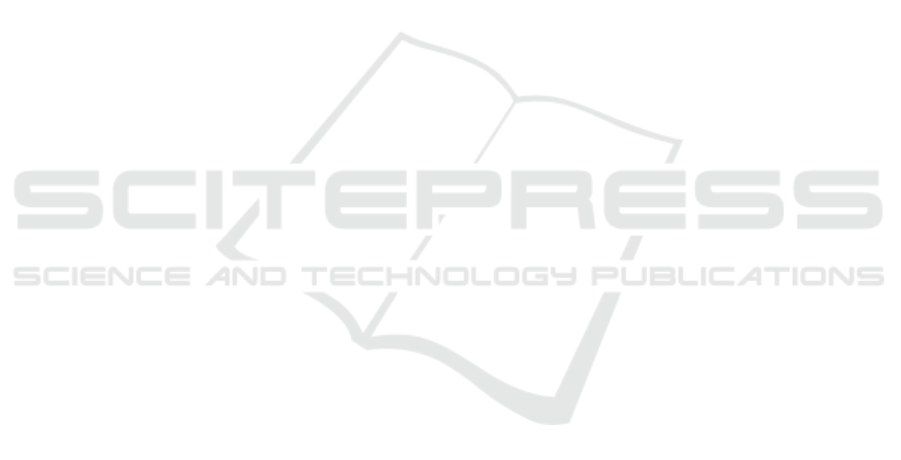
the eight basic emotions. To this end, we used
the novel concept of emotion-exchange motifs and
found that the exchange of sadness and joy are
structurally more similar than the exchange of any
other pair of emotions. Moreover, after cluster-
ing the emotion-exchange networks, two families of
networks emerged whose membership highly fluc-
tuates over time. According to their core mem-
bers we named the two families JSSIA (joy-sadness-
surprise-interlayer-aggregated) and PAF (positive-
anticipation-fear), whereby JSSIA has a more tightly
correlated core compared to PAF.
In our future work, we plan to further examine
the emergence of temporal emotion-exchange motifs
to provide an even more fine-grained analysis of the
underlying properties of human communication net-
works.
REFERENCES
Abdul-Mageed, M. and Ungar, L. (2017). Emonet: Fine-
grained emotion detection with gated recurrent neural
networks. In Proceedings of the 55th Annual Meeting
of the Association for Computational Linguistics (Vol-
ume 1: Long Papers), pages 718–728. Association for
Computational Linguistics.
Batson, C. D., Batson, J. G., Slingsby, J. K., Harrell, K. L.,
Peekna, H. M., and Todd, R. M. (1991). Empathic
joy and the empathy-altruism hypothesis. Journal of
personality and social psychology, 61(3):413–426.
Berger, J. (2011). Arousal increases social transmission of
information. Psychological Science, 22(7):891––893.
Cordella, L. P., Foggia, P., Sansone, C., and Vento, M.
(2004). A (sub)graph isomorphism algorithm for
matching large graphs. IEEE Transactions on Pat-
tern Analysis and Machine Intelligence, 26(10):1367–
1372.
Davis, J. A. and Leinhardt, S. (1972). The Structure
of Positive Interpersonal Relations in Small Groups.
Houghton Mifflin, Boston.
Flynn, B. (1997). Psychological aspects of disasters. Renal
Failure, 19(5):611–620.
Fraustino, J. D., Brooke, L., and Yan, J. (2012). Social
Media Use during Disasters: A Review of the Knowl-
edge Base and Gaps. Final Report to Human Fac-
tors/Behavioral Sciences Division, Science and Tech-
nology Directorate, U.S. Department of Homeland
Security, College Park, MD: START.
Freyd, J. J. (2002). In the wake of terrorist attack, hatred
may mask fear. Analysis of Social issues and public
policy, 2(1):5–8.
Gera, R., Alonso, L., and Crawford, B. (2018). Identify-
ing network structure similarity using spectral graph
theory. Applied Network Science, 3(2).
Hansen, L. K., Arvidsson, A., Nielsen, F. A., Colleoni, E.,
and Etter, M. (2011). Good friends, bad news - af-
fect and virality in twitter. In Park, J. J., Yang, L. T.,
and Lee, C., editors, Future Information Technology,
pages 34–43, Berlin, Heidelberg. Springer Berlin Hei-
delberg.
Holyst, J. (2016). Cyberemotions: Collective Emotions
in Cyberspace. Understanding Complex Systems.
Springer International Publishing.
Kim, H., Lee, S., Cappella, J., Vera, L., and Emery, S.
(2013). Content characteristics driving the diffusion
of antismoking messages: Implications for cancer pre-
vention in the emerging public communication envi-
ronment. Journal of National cancer institute. Mono-
graphs, 47:182–187.
Klaise, J. and Johnson, S. (2017). The origin of motif fam-
ilies in food webs. Nature Scientific Reports.
Koutra, D., JT., V., and C., F. (2013). Deltacon: A prin-
cipled massive-graph similarity function. In Proceed-
ings of the 2013 SIAM International Conference on
Data Mining, pages 162–170.
Ku
ˇ
sen, E., Cascavilla, G., Figl, K., Conti, M., and Strem-
beck, M. (2017). Identifying emotions in social me-
dia: Comparison of word-emotion lexicons. In Pro-
ceedings of the 4th International Symposium on So-
cial Networks Analysis, Management and Security
(SNAMS) (co-located with IEEE FiCloud). IEEE.
Ku
ˇ
sen, E. and Strembeck, M. (2019). An Analysis
of Emotion-Exchange Motifs in Multiplex Networks
During Emergency Events. Applied Network Science,
4(8):1–33.
Ku
ˇ
sen, E. and Strembeck, M. (2020). Evacuate everyone
south of that line: Analyzing structural communica-
tion patterns during natural disasters. Journal of Com-
putational Social Science. DOI: 10.1007/s42001-020-
00092-7.
Masoudi-Nejad, A., Schreiber, F., and Kashani, Z. (2012).
Building blocks of biological networks: A review on
major network motif discovery algorithms. IET Syst
Biol., 6(5).
Milo, R., Itzkovitz, S., Kashtan, N., Levitt, R., Shen-Orr,
S., Ayzenshtat, I., Sheffer, M., and Alon, U. (2004).
Superfamilies of evolved and designed networks. Sci-
ence, 303(5663):1538–1542.
Milo, R., Shen-Orr, S., Itzkovitz, S., Kashtan, N.,
Chklovskii, D., and Alon, U. (2002). Network motifs:
Simple building blocks of complex networks. Science,
298(5594):824–827.
Mohammad, S. M. and Turney, P. D. (2013). Crowdsourc-
ing a word-emotion association lexicon. Computa-
tional Intelligence, 29(3):436–465.
Newman, M. E. J., Strogatz, S. H., and Watts, D. J. (2001).
Random graphs with arbitrary degree distributions
and their applications. Phys. Rev. E, 64(2):026118.
Rim
´
e, B., Mesquita, B., Boca, S., and Philippot, P. (1991).
Beyond the emotional event: Six studies on the so-
cial sharing of emotion. Cognition and Emotion, 5(5-
6):435–465.
Scheve, Christian Salmella, M. (2014). Collective emotions.
Oxford University Press, Oxford, United Kingdom, 1
edition.
Shi, Y. and Macy, M. (2016). Measuring structural similar-
ity in large online networks. Social Science Research,
Structural Similarities of Emotion-exchange Networks: Evidence from 18 Crisis Events
35