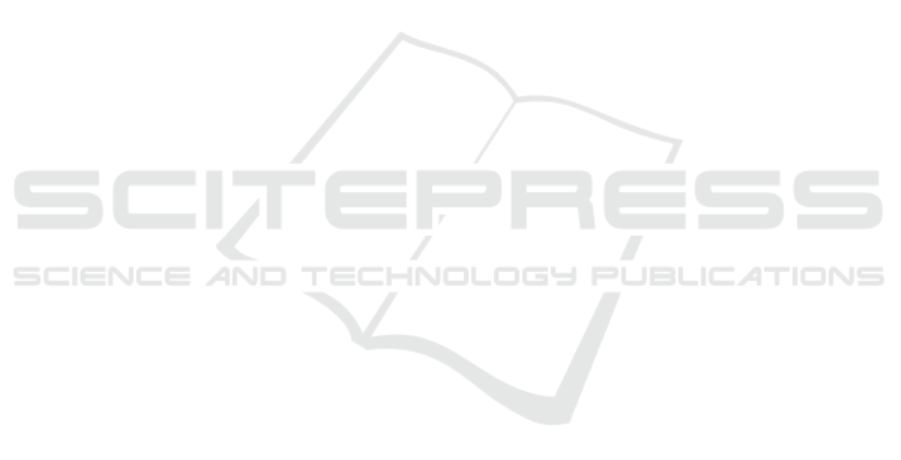
REFERENCES
Abras, C., Maloney-Krichmar, D., Preece, J., et al. (2004).
User-Centered Design. Bainbridge, W. Encyclope-
dia of Human-Computer Interaction. Thousand Oaks:
Sage Publications, pages 445–456.
Ali, M., Alqahtani, A., Jones, M. W., and Xie, X. (2019).
Clustering and Classification for Time Series Data in
Visual Analytics: A Survey. IEEE Access, pages
181314–181338.
Batch, A. and Elmqvist, N. (2018). The Interactive Vi-
sualization Gap in Initial Exploratory Data Analy-
sis. IEEE Transactions on Visualization and Com-
puter Graphics, pages 278–287.
Bernard, J., Zeppelzauer, M., Sedlmair, M., and Aigner, W.
(2018). Vial: a unified process for visual interactive
labeling. The Visual Computer, pages 1189–1207.
Cuturi, M. and Blondel, M. (2017). Soft-DTW: a differen-
tiable loss function for time-series. In 34th Interna-
tional Conference on Machine Learning, pages 894–
903.
Deng, H., Runger, G., Tuv, E., and Vladimir, M. (2013). A
time series forest for classification and feature extrac-
tion. Information Sciences, pages 142 – 153.
DIN 8584-3 (2003). Manufacturing processes forming un-
der combination of tensile and compressive conditions
- Part 3: Deep drawing; Classification, subdivision,
terms and definitions. Beuth Verlag, Berlin.
Eirich, J., J
¨
ackle, D., Schreck, T., Bonart, J., Posegga,
O., and Fischbach, K. (2020). VIMA: Modeling and
Visualization of High Dimensional Machine Sensor
Data Leveraging Multiple Sources of Domain Knowl-
edge. IEEE Transactions on Visualization and Com-
puter Graphics.
Granger, C. W. J. and Newbold, P. (2014). Forecasting eco-
nomic time series. Academic Press.
Hamilton, J. D. (2020). Time Series Analysis. Princeton
university press.
Holmes, G., Donkin, A., and Witten, I. H. (1994). Weka:
A machine learning workbench. In IEEE Australian
New Zealnd Intelligent Information Systems Confer-
ence, pages 357–361.
Hu, R., Granderson, J., Auslander, D., and Agogino, A.
(2019). Design of machine learning models with do-
main experts for automated sensor selection for en-
ergy fault detection. Applied Energy, pages 117–128.
Jeschke, S., Brecher, C., Meisen, T.,
¨
Ozdemir, D., and Es-
chert, T. (2017). Industrial internet of things and cy-
ber manufacturing systems. In Industrial internet of
things, pages 3–19. Springer.
Keim, D., Andrienko, G., Fekete, J.-D., G
¨
org, C., Kohlham-
mer, J., and Melanc¸on, G. (2008). Visual analytics:
Definition, process, and challenges. In Information
visualization, pages 154–175. Springer.
Law, S. M. (2019). STUMPY: A Powerful and Scalable
Python Library for Time Series Data Mining. The
Journal of Open Source Software, page 1504.
L
¨
oning, M., Bagnall, A., Ganesh, S., Kazakov, V., Lines, J.,
and Kir
´
aly, F. J. (2019). sktime: A Unified Interface
for Machine Learning with Time Series. In Workshop
on Systems for ML at NeurIPS 2019.
Meyes, R., Donauer, J., Schmeing, A., and Meisen, T.
(2019). A Recurrent Neural Network Architecture for
Failure Prediction in Deep Drawing Sensory Time Se-
ries Data. Procedia Manufacturing, pages 789 – 797.
47th North American Manufacturing Research Conf.
Pedregosa, F., Varoquaux, G., Gramfort, A., Michel, V.,
Thirion, B., Grisel, O., Blondel, M., Prettenhofer, P.,
Weiss, R., Dubourg, V., et al. (2011). Scikit-learn:
Machine learning in Python. the Journal of machine
Learning research, pages 2825–2830.
Pfeiffer, A., Bausch-Gall, I., and Otter, M. (2012). Proposal
for a Standard Time Series File Format in HDF5. 9th
International MODELICA Conf., pages 495–505.
Psuj, G. (2018). Multi-sensor data integration using deep
learning for characterization of defects in steel ele-
ments. Sensors, page 292.
Ronao, C. A. and Cho, S.-B. (2016). Human activity recog-
nition with smartphone sensors using deep learning
neural networks. Expert systems with applications,
pages 235–244.
Sacha, D., Stoffel, A., Stoffel, F., Kwon, B. C., Ellis, G., and
Keim, D. A. (2014). Knowledge Generation Model
for Visual Analytics. IEEE Transactions on Visual-
ization and Computer Graphics, pages 1604–1613.
Sakoe, H. and Chiba, S. (1978). Dynamic programming
algorithm optimization for spoken word recognition.
IEEE transactions on acoustics, speech, and signal
processing, pages 43–49.
Salvador, S. and Chan, P. (2007). FastDTW: Toward Ac-
curate Dynamic Time Warping in Linear Time and
Space. Intelligent Data Analysis, pages 561–580.
Tavenard, R., Faouzi, J., Vandewiele, G., Divo, F., Androz,
G., Holtz, C., Payne, M., Yurchak, R., Rußwurm, M.,
Kolar, K., and Woods, E. (2020). Tslearn, A Machine
Learning Toolkit for Time Series Data. Journal of Ma-
chine Learning Research, pages 1–6.
Van Someren, M., Barnard, Y., and Sandberg, J. (1994). The
think aloud method: a practical approach to modelling
cognitive. London: AcademicPress.
Wu, W., Zheng, Y., Chen, K., Wang, X., and Cao, N. (2018).
A visual analytics approach for equipment condition
monitoring in smart factories of process industry. In
2018 IEEE Pacific Visualization Symposium, pages
140–149.
Xu, P., Mei, H., Ren, L., and Chen, W. (2016). ViDX:
Visual diagnostics of assembly line performance in
smart factories. IEEE transactions on visualization
and computer graphics, pages 291–300.
Yeh, C.-C. M., Zhu, Y., Ulanova, L., Begum, N., Ding, Y.,
Dau, H. A., Silva, D. F., Mueen, A., and Keogh, E.
(2016). Matrix profile I: all pairs similarity joins for
time series: a unifying view that includes motifs, dis-
cords and shapelets. In IEEE 16th international con-
ference on data mining, pages 1317–1322.
Zhou, F., Lin, X., Liu, C., Zhao, Y., Xu, P., Ren, L., Xue,
T., and Ren, L. (2019). A survey of visualization for
smart manufacturing. Journal of Visualization, pages
419–435.
Visual Analytics for Industrial Sensor Data Analysis
593