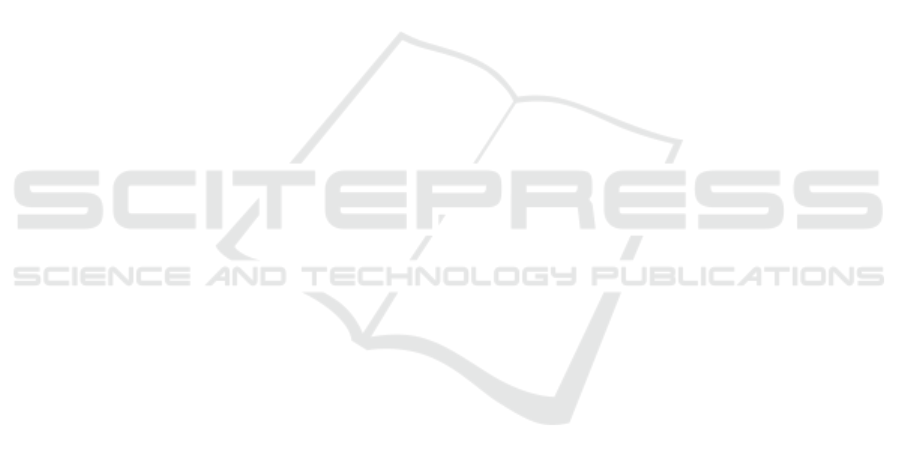
by the BOR ontology. Finally, performance analyses
on our classification methods, showed that they are
particularly suitable for textual classification.
For the future, there is still room for further im-
provements, such as exploit deep neural network al-
gorithms, such as those based in the Transformer ar-
chitecture, for nemad entity extraction. Another plan
is to assess the effectiveness of alternative learning
techniques for textual classification, as well as the use
of additional resources, particularly by adding new la-
beled bases.
ACKNOWLEDGEMENTS
The present work was carried out with the support
of the Coordenac¸
˜
ao de Aperfeic¸oamento de Pessoal
de N
´
ıvel Superior - Brazil (CAPES) - Financing
Code 001. The authors thank the partial support of
the CNPq (Brazilian National Council for Scientific
and Technological Development), FAPEMIG (Foun-
dation for Research and Scientific and Technological
Development of Minas Gerais), CEMIG, FUMEC,
LIAISE and PUC Minas.
REFERENCES
Aggarwal, C. C. and Zhai, C. (2012). A Survey of Text Clas-
sification Algorithms, pages 163–222. Springer US.
An, J., Quercia, D., and Crowcroft, J. (2014). Recommend-
ing investors for crowdfunding projects. In Proceed-
ings of the 23rd International Conference on World
Wide Web, WWW’14, pages 261–270.
Baeza-Yates, R. and Ribeiro-Neto, B. (2011). Modern in-
formation retrieval: the concepts and technology be-
hind search. Pearson Education.
Bai, J., Paradis, F., and yun Nie, J. (2004). Web-supported
matching and classification of business opportunities.
In Proceedings of the 2nd International Workshop on
Web-based Support Systems, WSS’04, pages 28–36.
Brand
˜
ao, W. C., Santos, R. L. T., Ziviani, N., Moura, E. S.,
and Silva, A. S. (2014). Learning to expand queries
using entities. Journal of the Association for Informa-
tion Science and Technology, 9:1870–1883.
Cowie, J. and Lehnert, W. (1996). Information extraction.
Communications of the ACM, pages 80–91.
Cutts, M. (2012). Spotlight keynote. In Proceedings of
Search Engines Strategies, SES’12.
Dr. K. Iyakutti, J. U. (2017). Mining association rules for
web crawling using genetic algorithm. International
Journal of Engineering and Computer Science, pages
2635–2640.
Duarte, J., Cavalcante, R., and Milidi
´
u, R. (2007). Ma-
chine learning algorithms for portuguese named en-
tity recognition. Inteligencia artificial: Revista
Iberoamericana de Inteligencia Artificial, pages 67–
75.
Falci, D., Dutra, F., Brand
˜
ao, W., Ferreira, E., and Parreiras,
F. (2020). Integrating ontologies for business expan-
sion information gathering. In Proceedings of the 13rd
Brazilian Seminar on Ontologies, ONTOBRAS’20.
Gruber, T. R. (1993). A translation approach to portable on-
tology specifications. Knowledge Acquisition, pages
199–220.
Jain, R. (1991). The art of computer systems perfor-
mance analysis - techniques for experimental de-
sign, measurement, simulation, and modeling. Wiley-
Interscience.
Kokossis, A., Ba
˜
nares-Alc
´
antara, R., Jim
´
enez, L., and
Linke, P. (2005). h-Techsight: a knowledge man-
agement platform for technology intensive industries.
In Proceedings of the 38th European Symposium on
Computer-Aided Process Engineering, ESCAPE’05,
pages 1345–1350.
Lai, Y.-A., Zhu, X., Zhang, Y., and Diab, M. (2020). Di-
versity, density, and homogeneity: Quantitative char-
acteristic metrics for text collections. In Proceedings
of the 12th Conference on Language Resources and
Evaluation, LREC’20, pages 1739–1746.
Minaee, S., Kalchbrenner, N., Cambria, E., Nikzad, N.,
Chenaghlu, M., and Gao, J. (2020). Deep learning
based text classification: A comprehensive review.
CoRR, abs/2004.03705.
Mladeni, D., Brank, J., and Grobelnik, M. (2010). Docu-
ment Classification, pages 289–293. Springer US.
Nadeau, D. and Sekine, S. (2007). A survey of named entity
recognition and classification. Lingvisticae Investiga-
tiones, pages 3–26.
Pant, G., Srinivasan, P., and Menczer, F. (2004). Crawling
the Web. In In Web dynamics: Adapting to change
in content, size, topology and use, pages 153–178.
Springer-Verlag New York, Inc.
Paradis, F., Nie, J.-Y., and Tajarobi, A. (2005). Discovery
of business opportunities on the internet with infor-
mation extraction. In Proceedings of the Workshop on
Multi-Agent Information Retrieval and Recommender
Systems, IJCAI’05.
Pirovani, J. and Oliveira, E. (2018). Portuguese named en-
tity recognition using conditional random fields and
local grammars. In Proceedings of the 11th Interna-
tional Conference on Language Resources and Evalu-
ation, LREC’18.
Saggion, H., Funk, A., Maynard, D., and Bontcheva, K.
(2007). Ontology-based information extraction for
business intelligence. In Proceedings of the 6th Inter-
national Semantic Web Conference, ISWC’07, pages
843–856. Springer Berlin Heidelberg.
Tajarobi, A., Garneau, J.-F., and Paradis, F. (2005). MBOI:
Discovery of business opportunities on the inter-
net. In Proceedings of HLT/EMNLP 2005 Interactive
Demonstrations, HLT-Demo’05, pages 30–31.
Yu, H., Guo, J., Yu, Z., Xian, Y., and Yan, X. (2014).
A novel method for extracting entity data from deep
web precisely. In Proceedings of the 26th Chinese
Control and Decision Conference, CCDC’14, pages
5049–5053.
Ontology-based Approach for Business Opportunities Recognition
601