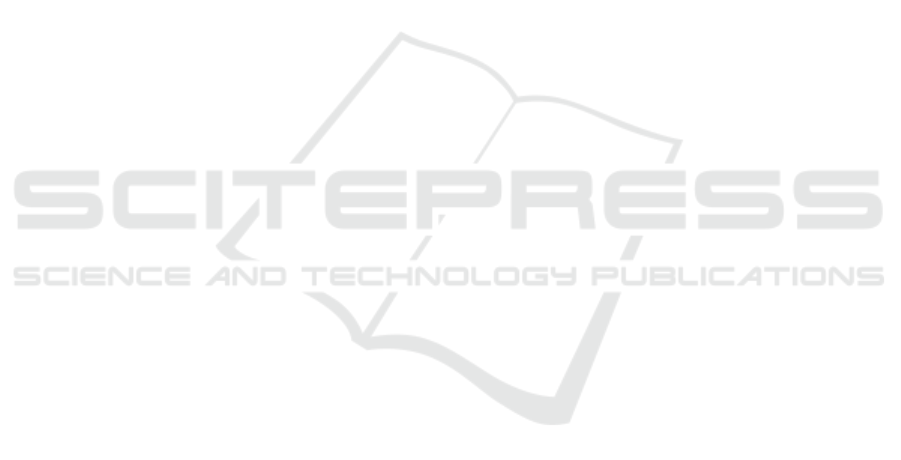
distance before and after the location of the weight
sensor to minimize the influence of vehicle dynamics
on the weight measurements as mentioned in (Weigh-
in-motion, Pocket Guide, 2018). A huge maintenance
and rehabilitation cost of WIM is required to increase
lifespan of WIM installation.
As a potential use case of ITS solution for Traffic
Safety on highways serving central and state
requirement to curb overloaded vehicle on national
and state highways, we propose a smart IoT sensor-
based technology. This system is easy to handle by its
users, portable, and requires minimum cost to
maintenance, difficult to defeat and provide reliable
information to the concerned regulators by
continuously monitoring and estimate weight from
moving vehicles on road.
Vehicle’s weight and its relationship to road
management is been identified as an important topic
of research in Transportation Engineering. In this
paper, we brief accelerometer-based sensing
techniques that are being used to determine
behaviours of vehicle dynamics in real-world
scenario of heavy-duty vehicles. The state-of-the art
Vehicle’s Weight Estimation by (Phong X. Nguyen et
al., 2018) uses smartphone’s acceleration data with
statistical features to predict the weight. However, the
method requires the vehicle to be at certain speed
range of 20-22 kmph to estimate vehicle’s weight
accurately and inconsistent for other speed ranges. In
real-time scenarios the vehicle’s weight requires to be
monitored continuously for violations, which limits
above method for such deployment.
In this paper, we aim to extend the method to
overcome the mentioned challenges, (a) evaluate
model performance consistency at different vehicle
speed profiles while vehicle is in motion on road, also
including vehicle in static state i.e., engine idling
condition; and (b) assess the optimal sensor location
for deployment on vehicle by evaluating with
heterogenous vehicle data.
Initially, a feasibility study was conducted to
estimate the vehicle’s weight method on data
obtained from different sources. The method failed to
provide a stable result due to variability of data source
as identified in Table 1. We then study in detail
different speed profiles of vehicle undergone during
the journey and propose an improved multiple linear
regression model by extracting more statistical
features from linear acceleration data which shows
high significance with load factor. The improved
method estimates vehicle’s weight with an average
error of 1800 kg, which accounts for 5.5% of true
average vehicle’s weight with vehicle in idling
condition; and 1932 kg, which accounts for 5.7% with
vehicle under constant speed range. We also compare
the model performance with other speed profile. We
extend the validation of our improvised method on
Indian road segment considering variability of data
with combinations of vehicle factors such as vehicle
class type, manufacture make, load, and goods. The
results obtained confirm the validity of applying the
improved method for determining the weight of
vehicle across vehicle class types and for all speed
ranges.
As a pilot project we likewise mount sensors on
three different locations in each vehicle to evaluate
the performance of our proposed solution and assess
which sensor location mount is ideal for our solution
considering as a system.
The rest of the paper is organized as follows.
Section 2 discusses related work on vehicle’s weight
estimation. Section 3 presents our proposed method.
In Sections 4 and 5 we present our experimental setup
and evaluation of the experimental results. In Section
6, we discuss the strengths and weaknesses of our
method. We conclude in Section 7 with a summary
and details on future work.
2 RELATED WORK
We identify that Weigh-in-Motion (WIM)
technology (Magdalena et al., 2020) is the closest
competitor to the technology under development,
some of which have accuracy more than 95%.
However, the technology is based on static sensors
over which the vehicle moves at low speed (LTBP,
2016). This solution however is limited to toll way
deployment. (Kadlecek et al., 2005) describes a
weight estimation method that measures the energy
output from engine of a given vehicle and measures
the acceleration derived of it. Here, we take the
inspiration of including energy as one of the
explanatory features to our model. (Jyotishman
Ghosh et al., 2017 and Nan Lin et al., 2019) describes
a real-time vehicle mass estimation from CAN data
and drivetrain torque observation. This technique
considers different driving forces acting on
longitudinal motion, where majority of contribution
is due to traction and braking forces. (Viengnam
Douangphachanh et al, 2014), describes collecting
sensing data from android smartphone. They find a
relationship of acceleration data with road roughness
condition and its significance partially dependent on
speed. This work is being investigated in frequency
domain to analyse the behaviour of road roughness on
an average speed. (Joshua E. Siegel et al., 2015),
explore a novel application of fault detection in
An Empirical Study on Low-cost, Portable Vehicle’s Weight Estimation Solution using Smartphone’s Acceleration Data for Developing
Countries
45