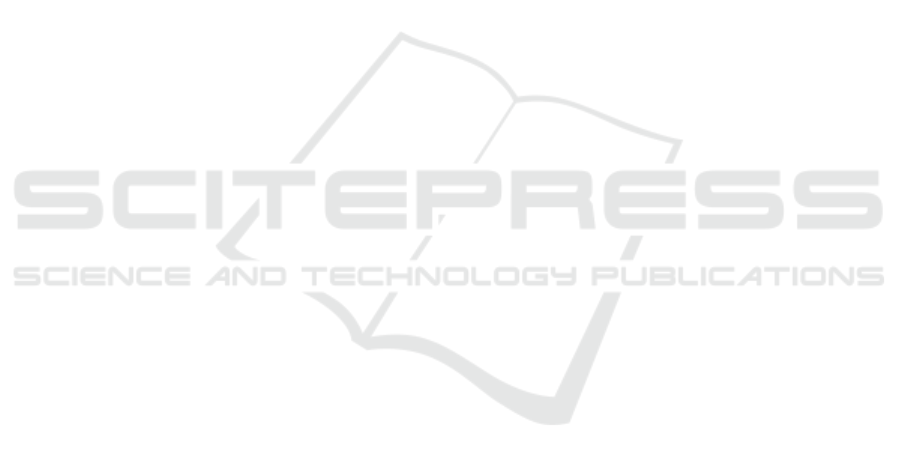
has being modeled in one document model can eas-
ily be used (with or without adaptations) in another
document model as the operators needed and their or-
der of application are known, only changes to the en-
try traces (as model’s parts are not the same) should
be adapted, as well as visualization modes, if neces-
sary/desired from the new context.
Repeatability: the property of an analysis process
to be carried out several times, on the same set of data
and with the same configurations, having the same
output results. In other words, this quality makes it
possible to trace the results produced and their con-
sistency (Lebis et al., 2018). Checking the repeata-
bility quality of implemented indicators should be fa-
cilitated as the same implementation would be avail-
able/tested in various practical contexts.
Reuse: the ability of software (or an analysis pro-
cess) to be reused in an application other than that
for which it was designed or in another context. The
same process must then be easily reused on another
data set which is more or less similar to the initial
one. Thus, possible adaptations can be made in order
to adapt the analysis to a similar context, for example,
to another document model, but with particular atten-
tion to guarantee that the foundation of the analysis
process (a learning, theory from which the indicator
was envisaged, for example) are respected and remain
consistent.
Lastly, by using our approach, a large variety of
indicators can be modeled, but it is also possible to
envisage the proposition of a given set of an indica-
tors ready to use (as a single “primitive”/component).
Specifically, an indicator, or series of indicators seen
as most useful/standards, can be modeled with the
primitives we propose and, at a later time, be “con-
densed” as a single primitive to be added to model-
ing processes, facilitating even more their implemen-
tation into other document models.
7 DISCUSSION
In this work we analyse the potential benefits of
implementing learning analytics solutions using a
model-driven approach in conjunction with digital
publishing chains that are also based on the same ap-
proach. The metamodel proposed aims at being suffi-
ciently abstract in order to allow the implementation
of the vast majority of learning analytics indicators,
with the advantage of counting on the prior knowl-
edge of documents semantics and structure.
Future work will be conducted aiming at modeling
a variety of indicators and measuring in more detail
the benefits discussed here.
REFERENCES
Arribe, T., Crozat, S., Bachimont, B., and Spinelli, S.
(2012). Cha
ˆ
ınes
´
editoriales num
´
eriques : allier effi-
cacit
´
e et variabilit
´
e gr
ˆ
ace
`
a des primitives documen-
taires. In Actes du colloque CIDE, pages 1–12, Tunis,
Tunisie.
Bachimont, B. and Crozat, S. (2004). Instrumentation
num
´
erique des documents : pour une s
´
eparation
fonds/forme. Revue I3 - Information Interaction In-
telligence, 4(1):95.
Choquet, C. and Iksal, S. (2007). Mod
´
elisation et
construction de traces d’utilisation d’une activit
´
e
d’apprentissage : une approche langage pour la
r
´
eing
´
enierie d’un EIAH. Sciences et Technolo-
gies de l’Information et de la Communication pour
l’
´
Education et la Formation, 14(1):419–456.
Combemale, B. (2008). Ing
´
enierie Dirig
´
ee par les Mod
`
eles
(IDM) –
´
Etat de l’art.
Crozat, S. (2007). Scenari, la cha
ˆ
ıne
´
editoriale libre. Ey-
rolles.
Dabbebi, I., Iksal, S., Gilliot, J.-M., May, M., and Garlatti,
S. (2017). Towards Adaptive Dashboards for Learning
Analytic: An Approach for Conceptual Design and
Implementation. In Proceedings of the 9th Interna-
tional Conference on Computer Supported Education
(CSEDU), pages 120–131, Porto, Portugal.
Djouad, T., Mille, A., Reffay, C., and Benmohamed, M.
(2009). Ing
´
enierie des indicateurs d’activit
´
es
`
a partir
de traces mod
´
elis
´
ees pour un Environnement informa-
tique d’apprentissage humain. Sciences et Technolo-
gies de l’Information et de la Communication pour
l’
´
Education et la Formation, 16(1):103–139.
Dyckhoff, A. L., Zielke, D., Chatti, M. A., and Schroeder,
U. (2012). Design and Implementation of a Learning
Analytics Toolkit for Teachers. Educational Technol-
ogy & Society, 15(01).
Ga
ˇ
sevi
´
c, D., Kovanovi
´
c, V., and Joksimovi
´
c, S. (2017).
Piecing the learning analytics puzzle: a consolidated
model of a field of research and practice. Learning:
Research and Practice, 3(1):63–78.
Guillaume, D., Crozat, S., Rivet, L., Majada, M., and Hen-
nequin, X. (2015). Cha
ˆ
ınes
´
editoriales num
´
eriques.
Hutchinson, J., Rouncefield, M., and Whittle, J. (2011).
Model-driven engineering practices in industry. In
Proceedings - International Conference on Software
Engineering, pages 633–640, New York, New York,
USA. ACM Press.
J
´
ez
´
equel, J.-M., Combemale, B., and Vojtisek, D. (2012).
Ing
´
enierie dirig
´
ee par les mod
`
eles - Des concepts
`
a la
pratique. Ellipses, Paris.
Lebis, A., Lefevre, M., Luengo, V., and Guin, N. (2018).
Capitalisation of Analysis Processes : Enabling Re-
producibility , Openess and Adaptability thanks to
Narration. In LAK ’18 - 8th International Conference
on Learning Analytics and Knowledge, pages 245–
254, Sydney, Australia.
Siemens, G. (2011). Learning and Academic Analytics.
Wise, A. F. and Vytasek, J. (2017). Learning Analytics Im-
plementation Design. In Lang, C., Siemens, G., Wise,
A., and Ga
ˇ
sevi
´
c, D., editors, Handbook of Learning
Analytics, chapter 13, pages 151–160. SoLAR, first
edition.
CSEDU 2021 - 13th International Conference on Computer Supported Education
54