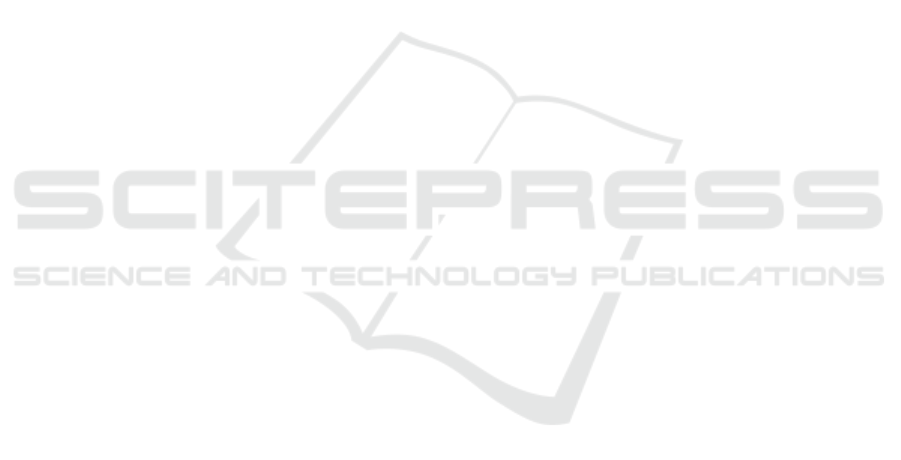
Echo360 (2020). Echo360- definitions of course analytics
metrics. https://learn.echo360.com/hc/en-us/articles/
360035037312. Accessed 25 11 2020.
Foldnes, N. (2016). The flipped classroom and cooperative
learning: Evidence from a randomised experiment.
Active Learning in Higher Education, 17:39–49.
Fuller, U., Johnson, C., Ahoniemi, T., Cukierman, D.,
Hern
´
an-Losada, I., Jackova, J., Lahtinen, E., Lewis,
T., Thompson, D., Riedesel, C., and Thompson, E.
(2007). Developing a computer science-specific learn-
ing taxonomy. ACM SIGCSE Bulletin, 39(4):152–170.
Granello, D. (2001). Promoting cognitive complexity in
graduate written work: Using bloom’s taxonomy as a
pedagogical tool to improve literature reviews. Coun-
selor Education and Supervision, 40(4):292–307.
Hussain, M., Zhu, W., Zhang, W., and Abidi, S. (2018). Stu-
dent engagement predictions in an e-learning system
andtheir impact on student course assessment scores.
Computational Intelligence and Neuroscience, 6:1–
21.
Isljamovic, S. and Suknovic, M. (2014). Predicting stu-
dents’ academic performance using artificial neural
network: a case study from faculty of organizational
sciences. In ICEMST 2014: International Conference
on Education in Mathematics, Science & Technology,
pages 68–72. ISRES Publishing.
Joffrion, H. (2005). Conceptual and procedural understand-
ing of algebra concepts in the middle grades. Master’s
thesis, Office of Graduate Studies of Texas A&M Uni-
versity.
Kardan, A. A., H, S., Ghidary, S., and Sani, M. (2013).
Prediction of student course selection in online higher
education institutes using neural network. Computers
& Education, 65:1–11.
Kostons, D. and Werf, G. (2015). The effects of activat-
ing prior topic and metacognitive knowledge on text
comprehension scores. British Journal of Educational
Psychology, 85(3):264–275.
Lau, E., Sun, L., and Yang, Q. (2019). Modelling, pre-
diction and classification of student academic perfor-
mance using artificial neural networks. SN Applied
Sciences, 1(982):1–10.
Macarini, L., Cechinel, C., Machado, M., Ramos, V.,
and Munoz, R. (2019). Predicting students success
in blended learning—evaluating different interactions
inside learning management systems. Applied Sci-
ences, 9:1–23.
MathWorks (2019). trainlm - Levernberg-Marquardt back-
propagation. http://uk.mathworks.com/help/nnet/ref/
trainlm.html. Accessed 17 6 2019.
Michalsky, T. and Zion, M. (2007). Developing students’
metacognitive awareness in asynchronous learning
networks in comparison to face-to-face discussion
groups. Journal of Educational Computing Research,
36(4):395–424.
Okubo, F., Yamashita, T., Shimada, A., and Ogata, H.
(2017). A neural network approach for students’ per-
formance prediction. In LAK17 - The Seventh Interna-
tional Learning Analytics & Knowledge Conference,
pages 598–599. Association for Computing Machin-
ery (ACM).
Othman, M. and Zain, N. (2015). Online collaboration
for programming: Assessing students’ cognitive abil-
ities. Turkish Online Journal of Distance Education-
TOJDE, 16(4):84–97.
¨
Ozc¸elik, S. and Hardalac¸, N. (2011). The statistical mea-
surements and neural network analysis of the effect
of musical education to musical hearing and sensing.
Expert Systems with Applications, 38:9517–9521.
Peter, D., Leth, T., and Bent, T. (2016). Assessing problem-
based learning in a software engineering curriculum
using bloom’s taxonomy and the ieee software engi-
neering body of knowledge. ACM Transactions on
Computing Education, 16(3):1–41.
Peterson, D. (2016). The flipped classroom improves stu-
dent achievement and course satisfaction in a statistics
course: A quasi-experimental study. Teaching of Psy-
chology, 43:10–15.
Qadir, J. and al-Furqaha, A. (2020). A student primer on
how to thrive in engineering education during and be-
yond covid-19. Education Sciences, 10(9):236–258.
Rashid, T. and Ahmad, H. (2016). Using neural network
with particle swarm optimization. Computing Appli-
cation Engineering Education, 24:629–638.
Sheth, S., Ganesh, A., Nagendra, S., Kumar, K., Tejdeepika,
R., Likhitha, C., Murthy, P., and Chand, P. (2020).
Development of a mobile responsive online learn-
ing module on psychosocial and mental health issues
related to covid 19. Asian Journal of Psychiatry,
54:236–258.
United Nations (2020). Policy brief: Education during
covid-19 and beyond - august 2020. Technical report,
United Nations.
Vandamme, J., Meskens, N., and Superby, J. (2007). Pre-
dicting academic performance by data mining meth-
ods. Education economics, 15(4):405–419.
Wilson, P. and Mantooth, H. (2013). Model-based engi-
neering for complex electronic systems. Newnes.
Wu, D., Bieber, M., and Hiltz, S. (2008). Engaging students
with constructivist participatory examinations in asyn-
chronous learning networks. Journal of Information
Systems Education, 19(3):321–330.
Yu, H. and Wilamowski, B. (2011). Levernberg Mar-
quardt training industrial electronic handbook, intel-
ligent systems, volume 5. CRC Press, 2 edition.
Zhang, Q., Kuldip, C., and Devabhaktuni, V. (2003). Ar-
tificial neural network for rf and microwave design –
from theory to practice. IEEE Transactions on Mi-
crowave Theory and Techniques, 51(4):1339–1350.
A Neural Network Modelling and Prediction of Students’ Progression in Learning: A Hybrid Pedagogic Method
91