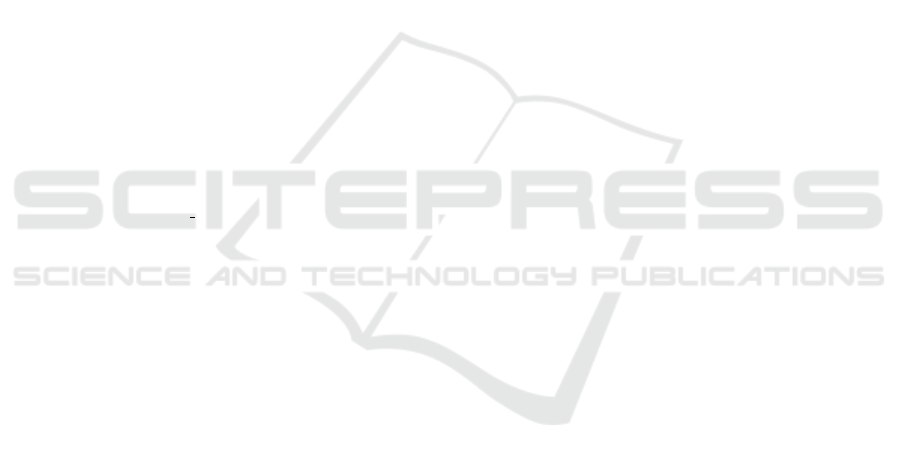
Cave, R. L. and Neuwirth, L. P. (1980). Hidden Markov
models for English. In Ferguson, J. D., editor, Hidden
Markov Models for Speech. IDA-CCR.
Chen, Y. and Wu, W. (2019). Separation of geochemical
anomalies from the sample data of unknown distribu-
tion population using gaussian mixture model. Com-
puters & Geosciences, 125:9–18.
Dang, S., Chaudhury, S., Lall, B., and Roy, P. K. (2017).
Learning effective connectivity from fMRI using au-
toregressive hidden Markov model with missing data.
Journal of Neuroscience Methods, 278:87–100.
Fraley, C. and Raftery, A. E. (2002). Model-based clus-
tering, discriminant analysis, and density estima-
tion. Journal of the American Statistical Association,
97(458):611–631.
Gallop, J. (2006). Facies probability from mixture distribu-
tions with non-stationary impedance errors. In SEG
Technical Program Expanded Abstracts 2006, pages
1801–1805. Society of Exploration Geophysicists.
Gao, Z., Sun, Z., and Liang, S. (2020). Probability density
function for wave elevation based on Gaussian mix-
ture models. Ocean Engineering, 213.
Guoning Hu and DeLiang Wang (2004). Monaural speech
segregation based on pitch tracking and amplitude
modulation. IEEE Transactions on Neural Networks,
15(5):1135–1150.
Interrante-Grant, A. M. and Kaeli, D. (2018). Gaus-
sian mixture models for dynamic malware
clustering. https://coe.northeastern.edu/wp-
content/uploads/pdfs/coe/research/embark/4-
interrante-grant.alex final.pdf.
Joyce, J. M. (2011). Kullback-Leibler divergence. In
Lovric, M., editor, International Encyclopedia of Sta-
tistical Science, pages 720–722. Springer.
Juang, B. (1985). Maximum-likelihood estimation for mix-
ture multivariate stochastic observations of Markov
chains. AT&T Technical Journal, 64(6):1235–1249.
Kalash, M., Rochan, M., Mohammed, N., Bruce, N. D. B.,
Wang, Y., and Iqbal, F. (2018). Malware classification
with deep convolutional neural networks. In 2018 9th
IFIP International Conference on New Technologies,
Mobility and Security, NTMS, pages 1–5.
Kruczkowski, M. and Szynkiewicz, E. N. (2014). Sup-
port vector machine for malware analysis and classi-
fication. In 2014 IEEE/WIC/ACM International Joint
Conferences on Web Intelligence and Intelligent Agent
Technologies, WI-IAT ’14, pages 415–420.
Laraba, S. and Tilmanne, J. (2016). Dance performance
evaluation using hidden Markov models. Computer
Animation and Virtual Worlds, 27(3-4):321–329.
McLachlan, G. and Peel, D. (2004). Finite Mixture Models.
Wiley.
Milosevic, N. (2013). History of malware. https://arxiv.org/
abs/1302.5392.
Nappa, A., Rafique, M. Z., and Caballero, J. (2015).
The MALICIA dataset: Identification and analysis of
drive-by download operations. International Journal
of Information Security, 14(1):15–33.
Neville, A. and Gibb, R. (2013). ZeroAccess Indepth. https:
//docs.broadcom.com/doc/zeroaccess-indepth-13-en.
Nguyen, L. (2016). Continuous observation hidden Markov
model. Revista Kasmera, 44(6):65–149.
Qiao, J., Cai, X., Xiao, Q., Chen, Z., Kulkarni, P., Ferris,
C., Kamarthi, S., and Sridhar, S. (2019). Data on MRI
brain lesion segmentation using k-means and Gaus-
sian mixture model-expectation maximization. Data
in Brief, 27.
Rabiner, L. R. (1989). A tutorial on hidden Markov models
and selected applications in speech recognition. Pro-
ceedings of the IEEE, 77(2):257–286.
Raitoharju, M., Garc
´
ıa-Fern
´
andez, A., Hostettler, R., Pich
´
e,
R., and S
¨
arkk
¨
a, S. (2020). Gaussian mixture models
for signal mapping and positioning. Signal Process-
ing, 168:107330.
Reynolds, D. (2015). Gaussian mixture models. In Li, S. Z.
and Jain, A. K., editors, Encyclopedia of Biometrics,
pages 827–832. Springer.
Stamp, M. (2018). A revealing introduction to hid-
den Markov models. https://www.cs.sjsu.edu/
∼
stamp/
RUA/HMM.pdf.
Stanculescu, I., Williams, C. K. I., and Freer, Y. (2014).
Autoregressive hidden Markov models for the early
detection of neonatal sepsis. IEEE Journal of Biomed-
ical and Health Informatics, 18(5):1560–1570.
Togneri, R. and DeSilva, C. J. S. (2003). Fundamentals of
Information Theory and Coding Design. CRC Press.
Truong, A. and Zaharia, T. (2017). Laban movement analy-
sis and hidden Markov models for dynamic 3D ges-
ture recognition. EURASIP Journal on Image and
Video Processing, 2017.
Winwebsec (2017). Win32/winwebsec threat descrip-
tion - Microsoft security intelligence. https:
//www.microsoft.com/en-us/wdsi/threats/malware-
encyclopedia-description?Name=Win32/Winwebsec.
Yao, Z., Ge, J., Wu, Y., Lin, X., He, R., and Ma, Y.
(2020). Encrypted traffic classification based on
Gaussian mixture models and hidden Markov models.
Journal of Network and Computer Applications, 166.
Zbot (2017). Pws:win32/zbot threat description - Microsoft
security intelligence. https://www.microsoft.com/en-
us/wdsi/threats/malware-encyclopedia-description?
Name=PWS%3AWin32%2FZbot.
Zhang, F., Han, S., Gao, H., and Wang, T. (2020). A Gaus-
sian mixture based hidden Markov model for motion
recognition with 3D vision device. Computers & Elec-
trical Engineering, 83.
ForSE 2021 - 5th International Workshop on FORmal methods for Security Engineering
762