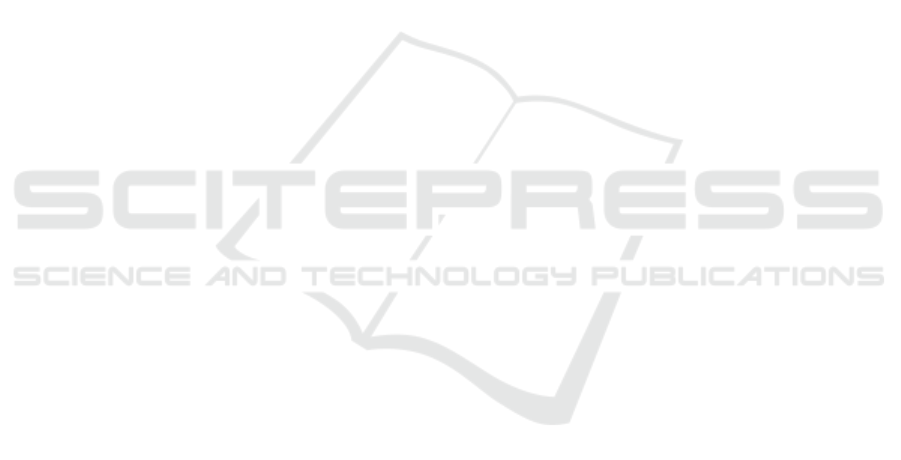
W., Fischer, I., Johnson, S., Nast, P. A., Costantinou,
E., and Fraser, V. J. (2004). Characteristics and cir-
cumstances of falls in a hospital setting: a prospec-
tive analysis. Journal of general internal medicine,
19(7):732–739.
Kitchenham, B., Brereton, O. P., Budgen, D., Turner, M.,
Bailey, J., and Linkman, S. (2009). Systematic litera-
ture reviews in software engineering–a systematic lit-
erature review. Information and software technology,
51(1):7–15.
Lee, J., Jin, Y., Piao, J., and Lee, S.-M. (2016). Devel-
opment and evaluation of an automated fall risk as-
sessment system. International Journal for Quality in
Health Care, 28(2):175–182.
Liberati, A., Altman, D. G., Tetzlaff, J., Mulrow, C.,
Gøtzsche, P. C., Ioannidis, J. P., Clarke, M., Dev-
ereaux, P. J., Kleijnen, J., and Moher, D. (2009).
The prisma statement for reporting systematic reviews
and meta-analyses of studies that evaluate health care
interventions: explanation and elaboration. PLoS
medicine, 6(7):e1–e34.
Lucero, R., Lindberg, D., Fehlberg, E., Bjarnadottir, R.,
Li, Y., Cimiotti, J., Crane, M., and Prosperi, M.
(2019). A data-driven and practice-based approach to
identify risk factors associated with hospital-acquired
falls: Applying manual and semi- and fully-automated
methods. International Journal of Medical Informat-
ics, 122:63–69.
Luther, S., McCart, J., Berndt, D., Hahm, B., Finch, D.,
Jarman, J., Foulis, P., Lapcevic, W., Campbell, R.,
Shorr, R., Valencia, K., and Powell-Cope, G. (2015).
Improving identification of fall-related injuries in am-
bulatory care using statistical text mining. American
Journal of Public Health, 105(6):1168–1173.
Marier, A., Olsho, L., Rhodes, W., and Spector, W. (2016).
Improving prediction of fall risk among nursing home
residents using electronic medical records. Jour-
nal of the American Medical Informatics Association,
23(2):276–282.
McCart, J., Berndt, D., Jarman, J., Finch, D., and Luther,
S. (2013). Finding falls in ambulatory care clini-
cal documents using statistical text mining. Jour-
nal of the American Medical Informatics Association,
20(5):906–914.
McHugh, M. L. (2012). Interrater reliability: the kappa
statistic. Biochemia medica: Biochemia medica,
22(3):276–282.
Moons, K. G., Altman, D. G., Reitsma, J. B., Ioannidis,
J. P., Macaskill, P., Steyerberg, E. W., Vickers, A. J.,
Ransohoff, D. F., and Collins, G. S. (2015). Trans-
parent reporting of a multivariable prediction model
for individual prognosis or diagnosis (tripod): expla-
nation and elaboration. Annals of internal medicine,
162(1):W1–W73.
Morse, J., Morse, R., and Tylko, S. (1989). Development
of a scale to identify the fall-prone patient. Canadian
Journal on Aging / La Revue canadienne du vieillisse-
ment, 8(4):366–377.
Mubashir, M., Shao, L., and Seed, L. (2013). A survey on
fall detection: Principles and approaches. Neurocom-
puting, 100:144 – 152. Special issue: Behaviours in
video.
Oshiro, C., Frankland, T., Rosales, A., Perrin, N., Bell, C.,
Lo, S., and Trinacty, C. (2019). Fall ascertainment and
development of a risk prediction model using elec-
tronic medical records. Journal of the American Geri-
atrics Society.
Resar, R., Rozich, J., Simmonds, T., and Haraden, C.
(2006). A trigger tool to identify adverse events in
the intensive care unit. Joint Commission Journal on
Quality and Patient Safety, 32(10):585–590.
Rochefort, C., Buckeridge, D., and Abrahamowicz, M.
(2015). Improving patient safety by optimizing the
use of nursing human resources. Implementation Sci-
ence, 10(1).
Santos, J., dos Santos, H. D. P., and Vieira, R. (2020).
Fall detection in clinical notes using language mod-
els and token classifier. In 2020 IEEE 33rd Interna-
tional Symposium on Computer-Based Medical Sys-
tems (CBMS), pages 283–288.
Shiner, B., Neily, J., Mills, P., and Watts, B. (2016). Iden-
tification of inpatient falls using automated review of
text-based medical records. Journal of Patient Safety.
Swift, C. G. and Iliffe, S. (2014). Assessment and preven-
tion of falls in older people–concise guidance. Clini-
cal medicine, 14(6):658.
Topaz, M., Murga, L., Gaddis, K., McDonald, M., Bar-
Bachar, O., Goldberg, Y., and Bowles, K. (2019).
Mining fall-related information in clinical notes:
Comparison of rule-based and novel word embedding-
based machine learning approaches. Journal of
Biomedical Informatics, 90.
Toyabe, S.-I. (2012). Detecting inpatient falls by using natu-
ral language processing of electronic medical records.
BMC Health Services Research, 12(1).
Tremblay, M., Berndt, D., Luther, S., Foulis, P., and French,
D. (2009). Identifying fall-related injuries: Text min-
ing the electronic medical record. Information Tech-
nology and Management, 10(4):253–265.
Walsh, M., Frances Horgan, N., Walsh, C., and Galvin, R.
(2016). Systematic review of risk prediction models
for falls after stroke. Journal of Epidemiology and
Community Health, 70(5):513–519.
Weed-Pfaff, S., Nutter, B., Bena, J., Forney, J., Field, R.,
Szoka, L., Karius, D., Akins, P., Colvin, C., and Al-
bert, N. (2016). Validation of predictors of fall events
in hospitalized patients with cancer. Clinical Journal
of Oncology Nursing, 20(5):E126–E131.
Yokota, S., Endo, M., and Ohe, K. (2017). Establishing
a classification system for high fall-risk among inpa-
tients using support vector machines. CIN - Comput-
ers Informatics Nursing, 35(8):408–416.
Yokota, S. and Ohe, K. (2016). Construction and evalua-
tion of find, a fall risk prediction model of inpatients
from nursing data. Japan Journal of Nursing Science,
13(2):247–255.
Zhu, V. J., Walker, T. D., Warren, R. W., Jenny, P. B.,
Meystre, S., and Lenert, L. A. (2017). Identifying
falls risk screenings not documented with administra-
tive codes using natural language processing. In AMIA
annual symposium proceedings, volume 2017, page
1923. American Medical Informatics Association.
Opportunities and Challenges in Fall Risk Management using EHRs and Artificial Intelligence: A Systematic Review
633