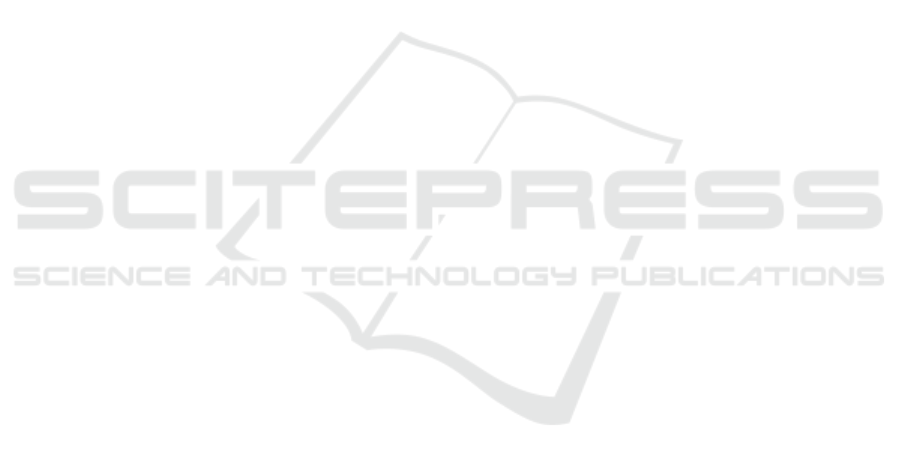
could help us to achieve better results. Also, trying a
word embedding approach may result in an improve-
ment of the models, but we have decided to focus on
studying the importance of the primary features.
Finally, we plan to test the classification models
with the best performance (best accuracy with a fewer
number of features) both for gender and for the rest
of the automatic profiling aspects in real applications
that are already doing author profiling. These tests
will allow us to evaluate efficient models versus those
that are already in use (with a higher number of fea-
tures), analyzing whether it is worth putting less time-
consuming models into production (with fewer fea-
tures) achieving similar accuracy results. Thus, we
could figure out which type of application it is appro-
priate employing the efficient model or on the con-
trary, in which applications it is better to opt for the
time-consuming solution.
ACKNOWLEDGEMENTS
This work was supported by projects RTI2018-
093336-B-C21, RTI2018-093336-B-C22 (Ministerio
de Ciencia e Innvovaci
´
on & ERDF) and the financial
support supplied by the Conseller
´
ıa de Educaci
´
on,
Universidade e Formaci
´
on Profesional (accreditation
2019-2022 ED431G/01, ED431B 2019/03) and the
European Regional Development Fund, which ac-
knowledges the CITIC Research Center in ICT of the
University of A Coru
˜
na as a Research Center of the
Galician University System.
REFERENCES
Aggarwal, J., Rabinovich, E., and Stevenson, S. (2020). Ex-
ploration of gender differences in covid-19 discourse
on reddit.
Alowibdi, J. S., Buy, U. A., and Yu, P. (2013). Empirical
evaluation of profile characteristics for gender classi-
fication on twitter. In 2013 12th International Con-
ference on Machine Learning and Applications, vol-
ume 1, pages 365–369.
Alowibdi, J. S., Buy, U. A., and Yu, P. (2013). Language
independent gender classification on twitter. In Pro-
ceedings of the 2013 IEEE/ACM International Con-
ference on Advances in Social Networks Analysis and
Mining, ASONAM ’13, page 739–743, New York,
NY, USA. Association for Computing Machinery.
´
Alvarez-Carmona, M. A., L
´
opez-Monroy, A. P., Montes-
y G
´
omez, M., Villase
˜
nor-Pineda, L., and Meza, I.
(2016). Evaluating topic-based representations for au-
thor profiling in social media. In Montes y G
´
omez, M.,
Escalante, H. J., Segura, A., and Murillo, J. d. D., ed-
itors, Advances in Artificial Intelligence - IBERAMIA
2016, pages 151–162, Cham. Springer International
Publishing.
Bacciu, A., Morgia, M. L., Mei, A., Nemmi, E. N., Neri, V.,
and Stefa, J. (2019). Bot and gender detection of twit-
ter accounts using distortion and LSA notebook for
PAN at CLEF 2019. CEUR Workshop Proceedings,
2380(July).
Blei, D. M., Ng, A. Y., and Jordan, M. I. (2003). Latent
Dirichlet allocation. Journal of Machine Learning Re-
search, 3(4–5), 993–1022.
Chopra, S., Sawhney, R., Mathur, P., and Shah, R. R.
(2020). Hindi-english hate speech detection: Author
profiling, debiasing, and practical perspectives. In
Proceedings of the AAAI Conference on Artificial In-
telligence, volume 34, pages 386–393.
Coates, J. (2015). Women, men and language: A soci-
olinguistic account of gender differences in language,
third edition (pp. 1–245). Taylor and Francis.
Dadvar, M., Jong, F. d., Ordelman, R., and Trieschnigg, D.
(2012). Improved cyberbullying detection using gen-
der information. In Proceedings of the Twelfth Dutch-
Belgian Information Retrieval Workshop (DIR 2012).
University of Ghent.
Ease, F. R. (2009). Flesch–Kincaid readability test.
Fatima, M., Hasan, K., Anwar, S., and Nawab, R. M. A.
(2017). Multilingual author profiling on facebook. Inf.
Process. Manage., 53(4):886–904.
Ke, G., Meng, Q., Finley, T., Wang, T., Chen, W., Ma,
W., Ye, Q., and Liu, T.-Y. (2017). LightGBM: A
Highly Efficient Gradient Boosting Decision Tree. In
Guyon, I., Luxburg, U. V., Bengio, S., Wallach, H.,
Fergus, R., Vishwanathan, S., and Garnett, R., editors,
Advances in Neural Information Processing Systems,
volume 30, pages 3146–3154. Curran Associates, Inc.
Kirasich, K., Smith, T. ., and Sadler, B. (2018). Random
Forest vs Logistic Regression: Binary Classification
for Heterogeneous Datasets. SMU Data Science Re-
view.
Koppel, M., Argamon, S., and Shimoni, A. R. (2002). Auto-
matically categorizing written texts by author gender.
Literary and linguistic computing, 17(4):401–412.
Levi, G. and Hassner, T. (2015). Age and gender classifica-
tion using convolutional neural networks. In Proceed-
ings of the IEEE Conference on Computer Vision and
Pattern Recognition (CVPR) Workshops.
Liu, B. (2010). Sentiment analysis and subjectivity. In In
Handbook of Natural Language Processing, Second
Edition (pp. 627–666). CRC Press.
Liu, B. (2012). Sentiment analysis and opinion mining.
Synthesis Lectures on Human Language Technologies,
5(1), 1–184.
Losada, D. E., Crestani, F., and Parapar, J. (2017). erisk
2017: Clef lab on early risk prediction on the inter-
net: Experimental foundations. In Jones, G. J., Law-
less, S., Gonzalo, J., Kelly, L., Goeuriot, L., Mandl,
T., Cappellato, L., and Ferro, N., editors, Experimen-
tal IR Meets Multilinguality, Multimodality, and Inter-
ENASE 2021 - 16th International Conference on Evaluation of Novel Approaches to Software Engineering
112