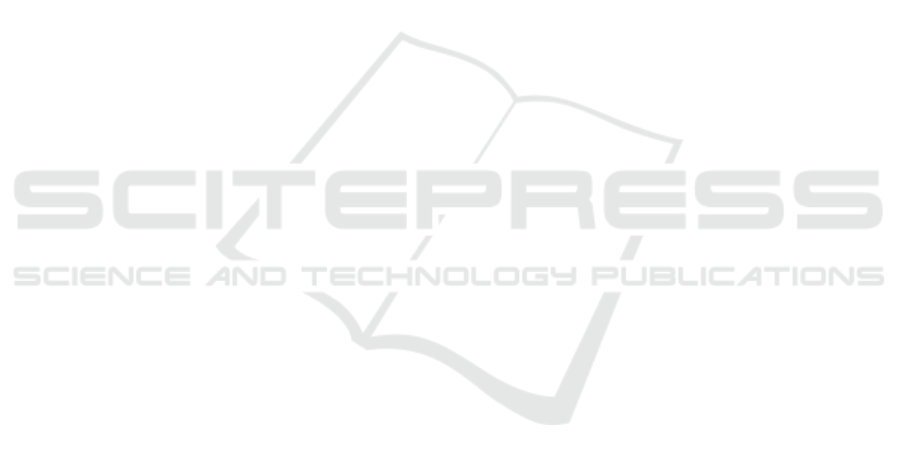
5 CONCLUSION AND FUTURE
WORK
In this paper, we proposed an approach that exploits
unsupervised adversarial domain adaptation guided
with target clustering, in order to improve the gener-
alization ability for face PAD. Specifically, our frame-
work utilizes UDA to learn domain invariant features
that could leverage from the labeled source samples
to classify the unlabeled samples from target domain.
Yet, the approach succeeds to preserve the intrinsic
properties of the target domain via deep clustering of
target embedding features. Our approach is trained
in an end-to-end fashion and succeeds to reach per-
fect adaptation to the target domain when evaluated
on public benchmark datasets, reaching only 0 - 2%
cross-dataset error. Our future work would focus on
evaluating on more variable datasets, in addition to
reducing the dependency of the model during training
on target domain samples from both classes, trying
to let the model focuses on learning from bona-fide
samples with minimal attack samples contribution.
REFERENCES
Anjos, A., Shafey, L. E., Wallace, R., G
¨
unther, M., Mc-
Cool, C., and Marcel, S. (2012). Bob: a free sig-
nal processing and machine learning toolbox for re-
searchers. In 20th ACM Conference on Multimedia
Systems (ACMMM), Nara, Japan.
Boulkenafet, Z., Komulainen, J., and Hadid, A. (2016).
Face spoofing detection using colour texture analysis.
IEEE Transactions on Information Forensics and Se-
curity, 11(8):1818–1830.
Boulkenafet, Z., Komulainen, J., and Hadid, A. (2017).
Face antispoofing using speeded-up robust features
and fisher vector encoding. IEEE Signal Processing
Letters, 24(2):141–145.
Chingovska, I., Anjos, A., and Marcel, S. (2012). On
the effectiveness of local binary patterns in face anti-
spoofing. In 2012 BIOSIG - Proceedings of the In-
ternational Conference of Biometrics Special Interest
Group (BIOSIG), pages 1–7.
Costa-Pazo, A., Bhattacharjee, S., Vazquez-Fernandez, E.,
and Marcel, S. (2016). The replay-mobile face
presentation-attack database. In 2016 International
Conference of the Biometrics Special Interest Group
(BIOSIG), pages 1–7.
Dizaji, K. G., Herandi, A., Deng, C., Cai, W., and Huang,
H. (2017). Deep clustering via joint convolutional au-
toencoder embedding and relative entropy minimiza-
tion. In IEEE international conference on Computer
Vision, pages 5747–5756.
El-Din, Y. S., Moustaf, M. N., and Mahdi, H. (2020a). On
the effectiveness of adversarial unsupervised domain
adaptation for iris presentation attack detection in mo-
bile devices. In ICMV’20.
El-Din, Y. S., Moustafa, M. N., and Mahdi, H. (2020b).
Deep convolutional neural networks for face and iris
presentation attack detection: survey and case study.
IET Biometrics, 9:179–193(14).
Feng, L., Po, L.-M., Li, Y., Xu, X., Yuan, F., Cheung, T.
C.-H., and Cheung, K.-W. (2016). Integration of im-
age quality and motion cues for face anti-spoofing: A
neural network approach. Journal of Visual Commu-
nication and Image Representation, 38:451 – 460.
Ganin, Y., Ustinova, E., Ajakan, H., Germain, P.,
Larochelle, H., Laviolette, F., Marchand, M., and
Lempitsky, V. (2016). Domain-adversarial train-
ing of neural networks. J. Mach. Learn. Res.,
17(1):2096–2030.
Goodfellow, I., Pouget-Abadie, J., Mirza, M., Xu, B.,
Warde-Farley, D., Ozair, S., Courville, A., and Ben-
gio, Y. (2014). Generative adversarial nets. In Ghahra-
mani, Z., Welling, M., Cortes, C., Lawrence, N. D.,
and Weinberger, K. Q., editors, Advances in Neu-
ral Information Processing Systems 27, pages 2672–
2680. Curran Associates, Inc.
Guo, X., Zhu, E., Liu, X., and Yin, J. (2018). Deep em-
bedded clustering with data augmentation. volume 95
of Proceedings of Machine Learning Research, pages
550–565. PMLR.
He, K., Zhang, X., Ren, S., and Sun, J. (2016). Deep resid-
ual learning for image recognition. In 2016 IEEE Con-
ference on Computer Vision and Pattern Recognition
(CVPR), pages 770–778.
Howard, A., Sandler, M., Chen, B., Wang, W., Chen, L.,
Tan, M., Chu, G., Vasudevan, V., Zhu, Y., Pang, R.,
Adam, H., and Le, Q. (2019). Searching for mo-
bilenetv3. In 2019 IEEE/CVF International Confer-
ence on Computer Vision (ICCV), pages 1314–1324.
Jia, Y., Zhang, J., Shan, S., and Chen, X. (2020). Single-
side domain generalization for face anti-spoofing. In
Proc. IEEE Conference on Computer Vision and Pat-
tern Recognition (CVPR).
Kang, G., Jiang, L., Wei, Y., Yang, Y., and Hauptmann,
A. G. (2020). Contrastive adaptation network for
single-and multi-source domain adaptation. IEEE
transactions on pattern analysis and machine intelli-
gence.
Krause, A., Perona, P., and Gomes, R. G. (2010). Discrim-
inative clustering by regularized information maxi-
mization. In Lafferty, J. D., Williams, C. K. I., Shawe-
Taylor, J., Zemel, R. S., and Culotta, A., editors, Ad-
vances in Neural Information Processing Systems 23,
pages 775–783. Curran Associates, Inc.
Kurmi, V. K. and Namboodiri, V. P. (2019). Looking back
at labels: A class based domain adaptation technique.
In International Joint Conference on Neural Networks
(IJCNN).
Li, H., He, P., Wang, S., Rocha, A., Jiang, X., and Kot,
A. C. (2018a). Learning generalized deep feature rep-
resentation for face anti-spoofing. IEEE Transactions
on Information Forensics and Security, 13(10):2639–
2652.
IMPROVE 2021 - International Conference on Image Processing and Vision Engineering
44