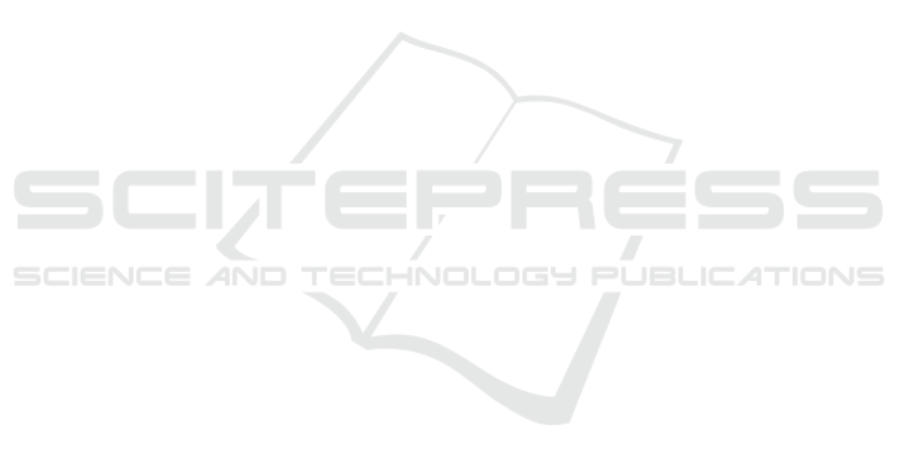
the most convenient supplier at every moment on the
basis of accurate predictions. This can be very rele-
vant when treating patients with urgent needs, as well
is fast-changing medical conditions, as the ones we
are currently facing in the COVID-19 pandemic.
Future research will incorporate forecasting of in-
ternal supply chain lead times of real service pro-
cesses. In this way, the forecast of lead time for pur-
chasing products will be coupled with the forecast of
the entire supply chain lead time, providing decision
makers with a larger instrument of analysis. In addi-
tion, more sophisticated approaches to lead time fore-
casting could be exploited, with simulation of non-
linear systems to investigate how machine faults and
maintenance procedures can influence lead time.
ACKNOWLEDGMENT
The research described in this paper was carried
out with funding from the Brazilian State Funding
Agency of Goi
´
as (FAPEG), Brazilian National Coun-
cil of State Funding Agencies (CONFAP-ITALY) and
Higher Education Personnel Improvement Coordina-
tion (CAPES).
REFERENCES
Albers, C. J., Critchley, F., and Gower, J. C. (2011).
Quadratic minimisation problems in statistics. Jour-
nal of Multivariate Analysis, 102(3):698–713.
Berling, P. and Farvid, M. (2014). Lead-time investiga-
tion and estimation in divergent supply chains. Inter-
national Journal of Production Economics, 157:177–
189.
Breiman, L., Friedman, J., Stone, C. J., and Olshen, R. A.
(1984). Classification and regression trees. CRC
press.
Brown, M. T., Bussell, J., Dutta, S., Davis, K., Strong, S.,
and Mathew, S. (2016). Medication adherence: truth
and consequences. The American journal of the med-
ical sciences, 351(4):387–399.
Cabrera-S
´
anchez, J.-P. and Villarejo-Ramos,
´
A. F. (2020).
Acceptance and use of big data techniques in services
companies. Journal of Retailing and Consumer Ser-
vices, 52(C):101888.
Chamikara, M., Bertok, P., Liu, D., Camtepe, S., and Khalil,
I. (2020). Efficient privacy preservation of big data for
accurate data mining. Information Sciences, 527:420–
443.
Chang, F.-C. (1997). Heuristics for dynamic job shop
scheduling with real-time updated queueing time esti-
mates. International Journal of Production Research,
35(3):651–665.
Chung, W., Talluri, S., and Kov
´
acs, G. (2018). Investigat-
ing the effects of lead-time uncertainties and safety
stocks on logistical performance in a border-crossing
jit supply chain. Computers & Industrial Engineering,
118:440–450.
Dean, J. (2014). Big data, data mining, and machine learn-
ing: value creation for business leaders and practi-
tioners. John Wiley & Sons.
Drucker, H., Burges, C. J., Kaufman, L., Smola, A. J.,
and Vapnik, V. (1997). Support vector regression ma-
chines. In Mozer, M. C., Jordan, M. I., and Petsche,
T., editors, Advances in neural information processing
systems 9, pages 155–161. MIT Press.
Eberle, L. G., Sugiyama, H., and Schmidt, R. (2014). Im-
proving lead time of pharmaceutical production pro-
cesses using monte carlo simulation. Computers &
Chemical Engineering, 68:255–263.
Fayyad, U., Piatetsky-Shapiro, G., and Smyth, P.
(1996). From data mining to knowledge discovery in
databases. AI magazine, 17(3):37.
Frank, A. G., Dalenogare, L. S., and Ayala, N. F. (2019).
Industry 4.0 technologies: Implementation patterns in
manufacturing companies. International Journal of
Production Economics, 210:15–26.
Gatica, G., Shah, N., and Papageorgiou, L. G. (2001). Ca-
pacity planning under clinical trials uncertainty for the
pharmaceutical industry. In Gani, R. and Jørgensen,
S. B., editors, European Symposium on Computer
Aided Process Engineering - 11, volume 9 of Com-
puter Aided Chemical Engineering, pages 865 – 870.
Elsevier.
Goltsos, T. E., Ponte, B., Wang, S., Liu, Y., Naim, M. M.,
and Syntetos, A. A. (2019). The boomerang returns?
accounting for the impact of uncertainties on the dy-
namics of remanufacturing systems. International
Journal of Production Research, 57(23):7361–7394.
Gyulai, D., Pfeiffer, A., Nick, G., Gallina, V., Sihn, W., and
Monostori, L. (2018). Lead time prediction in a flow-
shop environment with analytical and machine learn-
ing approaches. IFAC-PapersOnLine, 51(11):1029–
1034.
Harapan, H., Itoh, N., Yufika, A., Winardi, W., Keam, S.,
Te, H., Megawati, D., Hayati, Z., Wagner, A. L.,
and Mudatsir, M. (2020). Coronavirus disease 2019
(covid-19): A literature review. Journal of Infection
and Public Health, 13(5):667 – 673.
Haugh, K. H. (2014). Medication adherence in older adults:
The pillbox half full. Nursing Clinics of North Amer-
ica, 49(2):183–199.
Hosoda, T. and Disney, S. M. (2018). A unified theory of
the dynamics of closed-loop supply chains. European
Journal of Operational Research, 269(1):313–326.
Hu, X., Pedrycz, W., and Wang, X. (2018). Fuzzy classifiers
with information granules in feature space and logic-
based computing. Pattern Recognition, 80:156–167.
Ioannou, G. and Dimitriou, S. (2012). Lead time estima-
tion in mrp/erp for make-to-order manufacturing sys-
tems. International Journal of Production Economics,
139(2):551–563.
Jun, H.-B., Park, J.-Y., and Suh, H.-W. (2006). Lead time
estimation method for complex product development
process. Concurrent Engineering, 14(4):313–328.
ICEIS 2021 - 23rd International Conference on Enterprise Information Systems
640