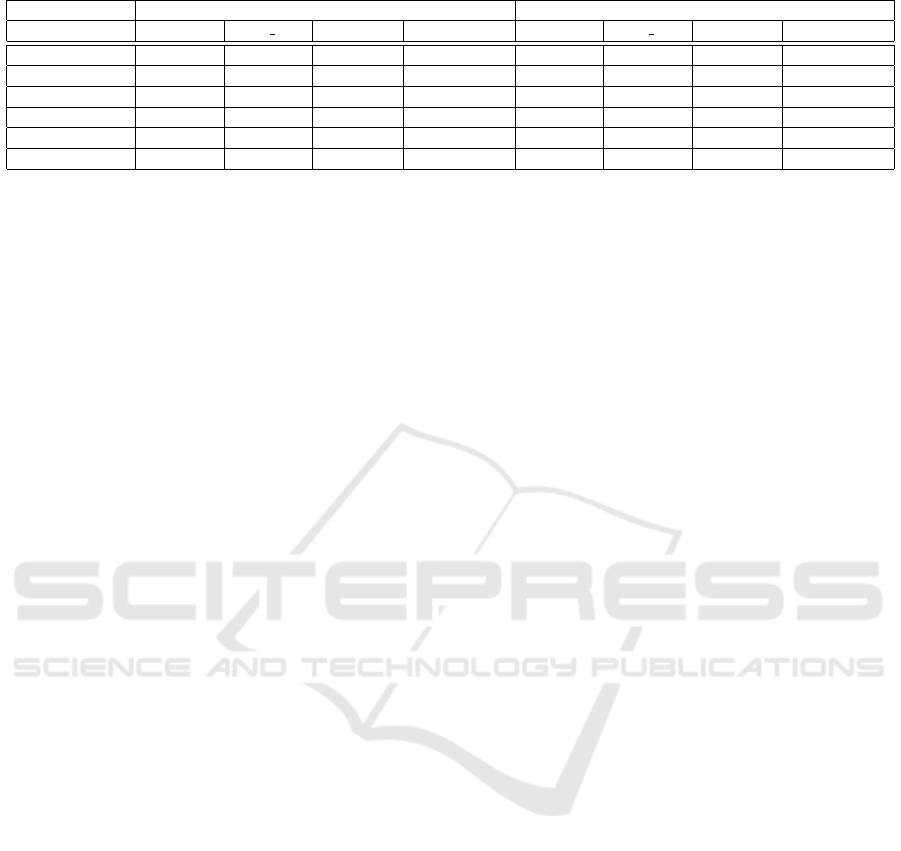
Table 3: Computational complexity comparison for the tested methods.
Input RA RAD
Model PolarNet FCN tiny FCN DeepLabv3+ PolarNet FCN tiny FCN DeepLabv3+
GPU (fps) 575.01 324.50 300.75 275.15 364.89 249.79 224.56 199.83
CPU (fps) 271.39 267.58 118.56 61.91 208.14 189.12 133.78 61.93
TX2 GPU (fps) 54.65 31.91 29.18 22.39 48.18 28.87 28.91 21.46
TX2 CPU (fps) 25.90 41.62 22.20 10.46 17.97 36.86 19.68 10.09
# parameters 562,472 210,279 2,933,449 3,223,865 598,758 214,817 2,951,593 3,242,009
Memory cost 29.85 Mb 16.02 Mb 59.64 Mb 134.86 Mb 39.79 Mb 23.04 Mb 70.81 Mb 143.74 Mb
Chen, L.-C., Papandreou, G., Kokkinos, I., Murphy, K.,
and Yuille, A. L. (2018a). Deeplab: Semantic im-
age segmentation with deep convolutional nets, atrous
convolution, and fully connected crfs. IEEE Trans-
actions on Pattern Analysis and Machine Intelligence,
40(4):834–848.
Chen, L.-C., Papandreou, G., Schroff, F., and Adam, H.
(2017). Rethinking atrous convolution for semantic
image segmentation.
Chen, L.-C., Zhu, Y., Papandreou, G., Schroff, F., and
Adam, H. (2018b). Encoder-decoder with atrous sep-
arable convolution for semantic image segmentation.
Lecture Notes in Computer Science, page 833–851.
Farina, A. and Studer, F. A. (1986). A review of cfar detec-
tion techniques in radar systems. Microwave Journal,
29:115.
He, K., Gkioxari, G., Doll
´
ar, P., and Girshick, R. (2017).
Mask r-cnn.
Ioffe, S. and Szegedy, C. (2015). Batch normalization: Ac-
celerating deep network training by reducing internal
covariate shift. arXiv preprint arXiv:1502.03167.
Levi, D., Garnett, N., Fetaya, E., and Herzlyia, I. (2015).
Stixelnet: A deep convolutional network for obstacle
detection and road segmentation.
Lin, T.-Y., Goyal, P., Girshick, R., He, K., and Doll
´
ar, P.
(2017). Focal loss for dense object detection.
Long, J., Shelhamer, E., and Darrell, T. (2015). Fully con-
volutional networks for semantic segmentation.
Minkler, G. and Minkler, J. (1990). Cfar: the principles of
automatic radar detection in clutter. NASA STI/Recon
Technical Report A, 90.
Noh, H., Hong, S., and Han, B. (2015). Learning deconvo-
lution network for semantic segmentation.
Nowruzi, F. E., Kolhatkar, D., Kapoor, P., Heravi, E. J., La-
ganiere, R., Rebut, J., and Malik, W. (2020). Deep
open space segmentation using automotive radar.
Qi, C. R., Yi, L., Su, H., and Guibas, L. J. (2017). Point-
net++: Deep hierarchical feature learning on point sets
in a metric space.
Redmon, J. and Farhadi, A. (2018). Yolov3: An incremental
improvement.
Ren, S., He, K., Girshick, R., and Sun, J. (2015). Faster
r-cnn: Towards real-time object detection with region
proposal networks.
Ronneberger, O., Fischer, P., and Brox, T. (2015). U-net:
Convolutional networks for biomedical image seg-
mentation. Medical Image Computing and Computer-
Assisted Intervention – MICCAI 2015, page 234–241.
Schneider, L., Cordts, M., Rehfeld, T., Pfeiffer, D., En-
zweiler, M., Franke, U., Pollefeys, M., and Roth, S.
(2016). Semantic stixels: Depth is not enough.
Sless, L., Cohen, G., Shlomo, B. E., and Oron, S. (2019).
Road scene understanding by occupancy grid learning
from sparse radar clusters using semantic segmenta-
tion.
Srivastava, N., Hinton, G., Krizhevsky, A., Sutskever, I.,
and Salakhutdinov, R. (2014). Dropout: a simple way
to prevent neural networks from overfitting. The jour-
nal of machine learning research, 15(1):1929–1958.
Szegedy, C., Ioffe, S., Vanhoucke, V., and Alemi, A. (2016).
Inception-v4, inception-resnet and the impact of resid-
ual connections on learning.
Zhao, H., Shi, J., Qi, X., Wang, X., and Jia, J. (2017). Pyra-
mid scene parsing network. 2017 IEEE Conference on
Computer Vision and Pattern Recognition (CVPR).
Zhou, Z., Siddiquee, M. M. R., Tajbakhsh, N., and Liang,
J. (2018). Unet++: A nested u-net architecture for
medical image segmentation.
VEHITS 2021 - 7th International Conference on Vehicle Technology and Intelligent Transport Systems
420