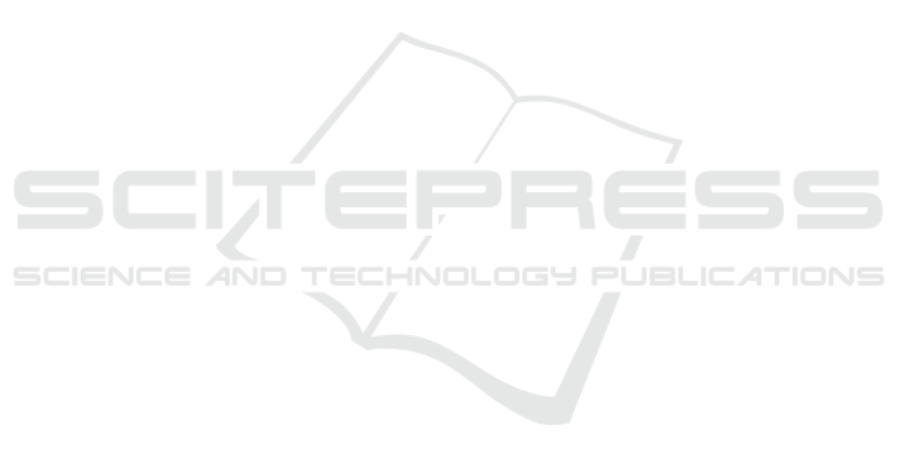
ages have been considered. Preliminary and extensive
tests performed on a publicly available database have
shown that the proposed feature has some potential in
contributing to the solution of the source identifica-
tion problem and it is able to reach high success rates
whenever properly estimated. On the other hand, it
has the advantage of being independent of image size,
so that artificial operations are useless whenever two
PRNU images have to be compared; finally, due to
its nature, it can show some robustness to background
noise sources. Future research will be then devoted to
refine the proposed feature, making it able to capture
both global and local image characteristics; to extend
it to natural images and to evaluate its performance on
natural images and different datasets.
REFERENCES
Akshatha, K., A.K. Karunakar, H. A., Raghavendra, U., and
Shetty, D. (2016). Digital camera identification using
prnu: A feature based approach, digital investigation.
In Digital Investigation.
Al-Ani, M. and Khelifi, F. (2017). On the spn estimation in
image forensics: A systematic empirical evaluation.
In IEEE Trans. on Inf. Forensics and Security.
Caldelli, R., Amerini, I., and Tsun, L. C. (2018). Prnu-based
image classification of origin social network with cnn.
In Proc. of EUSIPCO 2018.
Chen, M., Fridrich, J., Goljan, M., and Lukas, J. (2008).
Determining image origin and integrity using sensor
noise. In IEEE Trans. on Inf. Forensics and Security.
Chierchia, G., Parrilli, S., Poggi, G., Sansone, C., and Ver-
doliva, L. (2010). On the influence of denoising in
prnu based forgery detection. In Proc. of the 2nd ACM
workshop on Multimedia in forensics, security and in-
telligence.
Chierchia, G., Poggi, G., Sansone, C., and Verdoliva, L.
(2014). A bayesian-mrf approach for prnu-based im-
age forgery detection. In IEEE Trans. on Information
Forensics and Security.
Duff, G. F. D. (1967). Differences, derivatives, and decreas-
ing rearrangements. In Canadian Journal of Mathe-
matics.
Ferreira, P. (2001). Sorting continuous-time signals: Ana-
log median and median-type filters. In IEEE Trans. on
Signal Processing.
Fridrich, J. (2009). Digital image forensics. In IEEE Signal
Processing Magazine.
Georgievska, S., Bakhshi, R., Gavai, A., Sclocco, A., and
van Werkhoven, B. (2017). Clustering image noise
patterns by embedding and visualization for common
source camera detection. In Digital Investigation.
Gloe, T. and Bhme, R. (2010). The dresden image database
for benchmarking digital image forensics. In Journal
of Digital Forensic Practice.
Huang, Y., Zhang, J., and Huang, H. (2015). Camera model
identification with unknown models. In IEEE Trans.
on Information Forensics and Security.
Korus, P. and Huang, J. (2016). Multi-scale analysis strate-
gies in prnu-based tampering localization. In IEEE
Trans. on Information Forensics and Security.
Kumar, B. V. K. V. and Hassebrook, L. (1990). Performance
measures for correlation filters. In Appl. Opt.
Li, R., Li, C. T., and Guan, Y. (2018). Inference of a com-
pact representation of sensor fingerprint for source
camera identification. In Pattern Recognition.
Lukas, J., Fridrich, J., and Goljan, M. (2006). Digital cam-
era identification from sensor pattern noise. In IEEE
Trans. on Information Forensics and Security.
Mallat, S. (1998). A wavelet tour of signal processing. In
Academic Press.
Marra, F., Poggi, G., Sansone, C., and Verdoliva, L. (2017).
Blind prnu-based image clustering for source identifi-
cation. In IEEE Trans. on Inf. For. and Sec.
Salvi, V. B. A. and Vitulano, D. (2019). Joint correlation
measurements for prnu-based source identification. In
Lecture Notes in Computer Science, (Proc. of CAIP
2019).
Thaia, T. H., Retraintband, F., and Cogranne, R. (2015).
Camera model identification based on the generalized
noise model in natural images. In Digital Signal Pro-
cessing.
Tiwari, M. and Gupta, B. (2018). Efficient prnu extraction
using joint edge-preserving filtering for source cam-
era identification and verification. In Proc. of IEEE
ASPCON 2018.
Valsesia, D., Coluccia, G., Bianchi, T., and Magli, E.
(2017). User authentication via prnu-based physical
unclonable functions. In IEEE Trans. on Information
Forensics and Security.
Xu, B., Wang, X., Zhou, X., Xi, J., and Wang, S. (2016).
Source camera identification from image texture fea-
tures. In Neurocomputing.
Zhao, Y., Zheng, N., and T. Qiao, M. X. (2019). Source
camera identification via low dimensional prnu fea-
tures. In Multimedia Tools and Applications.
IMPROVE 2021 - International Conference on Image Processing and Vision Engineering
106