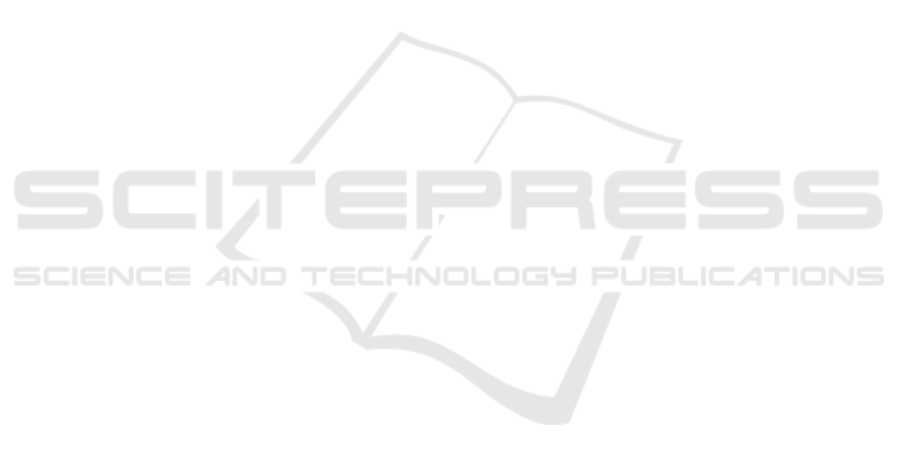
6 CONCLUSIONS
In this paper, we presented a novel approach to re-
construct an underwater surface with a mono cam-
era. The method does not require any restriction of
the camera motion or specific sensors, and the 3D co-
ordinates of underwater surface points can be deter-
mined in a least-square sense. The method is useful
to increase vehicles’ intelligence with water hazard
depth estimation both in on-road and off-road cases.
This phenomenon was illustrated in real-life scenar-
ios with onboard stereo cameras.
The method will be more elaborated for practical so-
lutions, as we would like to investigate how other ve-
hicles, transportation system can benefit from our pro-
posed method, and what is the optimal optical struc-
ture for the different vehicles.
ACKNOWLEDGEMENTS
The research presented in this paper, carried out by
Institute for Computer Science and Control was sup-
ported by the Ministry for Innovation and Technology
and the National Research, Development and Innova-
tion Office within the framework of the National Lab
for Autonomous Systems.
REFERENCES
Agrafiotis, P., Karantzalos, K., Georgopoulos, A., and Skar-
latos, D. (2020). Correcting image refraction: To-
wards accurate aerial image-based bathymetry map-
ping in shallow waters. Remote Sensing, 12(2).
Agrafiotis, P., Skarlatos, D., Georgopoulos, A., and
Karantzalos, K. (2019). Shallow water bathymetry
mapping from uav imagery based on machine learn-
ing. ISPRS - International Archives of the Photogram-
metry, Remote Sensing and Spatial Information Sci-
ences, XLII-2/W10:9–16.
Agrawal, A., Ramalingam, S., Taguchi, Y., and Chari, V.
(2012). A theory of multi-layer flat refractive geom-
etry. In 2012 IEEE Conference on Computer Vision
and Pattern Recognition, pages 3346–3353.
Chari, V. and Sturm, P. (2009). Multi-view geometry of the
refractive plane.
Chen, L., Yang, J., and Kong, H. (2017). Lidar-histogram
for fast road and obstacle detection. In 2017 IEEE
International Conference on Robotics and Automation
(ICRA), pages 1343–1348.
Chen, L.-C., Zhu, Y., Papandreou, G., Schroff, F., and
Adam, H. (2018). Encoder-decoder with atrous sep-
arable convolution for semantic image segmentation.
In Proceedings of the European Conference on Com-
puter Vision (ECCV).
Costa, B., Battista, T., and Pittman, S. (2009). Com-
parative evaluation of airborne lidar and ship-based
multibeam sonar bathymetry and intensity for map-
ping coral reef ecosystems. Remote Sensing of Envi-
ronment, 113(5):1082 – 1100.
Dietrich, J. T. (2017). Bathymetric structure-from-motion:
extracting shallow stream bathymetry from multi-
view stereo photogrammetry. Earth Surface Processes
and Landforms, 42(2):355–364.
Fryer, J. (1983). Photogrammetry through shallow water.
Australian journal of geodesy, photogrammetry, and
surveying, 38:25–38.
Fryer, J. G. and Kniest, H. T. (1985). Errors in depth
determination caused by waves in through-water
photogrammetry. The Photogrammetric Record,
11(66):745–753.
Gomez, C. and Purdie, H. (2016). UAV- based photogram-
metry and geocomputing for hazards and disaster risk
monitoring – a review. Geoenvironmental Disasters,
3.
Han, X., Nguyen, C., You, S., and Lu, J. (2018). Single
image water hazard detection using FCN with reflec-
tion attention units. In Proceedings of the European
Conference on Computer Vision (ECCV).
Haris, M. and Hou, J. (2020). Obstacle detection and safely
navigate the autonomous vehicle from unexpected ob-
stacles on the driving lane. Sensors, 20:4719.
Heikkila, J. and Silven, O. (1997). A four-step camera cal-
ibration procedure with implicit image correction. In
Proceedings of IEEE Computer Society Conference
on Computer Vision and Pattern Recognition, pages
1106–1112.
Hirschmuller, H. (2005). Accurate and efficient stereo pro-
cessing by semi-global matching and mutual informa-
tion. In 2005 IEEE Computer Society Conference on
Computer Vision and Pattern Recognition (CVPR’05),
volume 2, pages 807–814 vol. 2.
Jordt-Sedlazeck, A. and Koch, R. (2013). Refractive
structure-from-motion on underwater images. In 2013
IEEE International Conference on Computer Vision,
pages 57–64.
Kang, L., Wu, L., and Yang, Y.-H. (2012). Two-view un-
derwater structure and motion for cameras under flat
refractive interfaces. In Fitzgibbon, A., Lazebnik, S.,
Perona, P., Sato, Y., and Schmid, C., editors, Com-
puter Vision – ECCV 2012, pages 303–316, Berlin,
Heidelberg. Springer Berlin Heidelberg.
Maas, H.-G. (2015). On the accuracy potential in under-
water/multimedia photogrammetry. Sensors (Basel,
Switzerland), 15:18140–52.
Mettes, P., Tan, R. T., and Veltkamp, R. C. (2017). Water de-
tection through spatio-temporal invariant descriptors.
Computer Vision and Image Understanding, 154:182
– 191.
Murai, S., Kuo, M.-Y. J., Kawahara, R., Nobuhara, S., and
Nishino, K. (2019). Surface normals and shape from
water. In Proceedings of the IEEE/CVF International
Conference on Computer Vision (ICCV).
Mustaniemi, J., Kannala, J., S
¨
arkk
¨
a, S., Matas, J., and
Heikkil
¨
a, J. (2017). Inertial-based scale estimation
VEHITS 2021 - 7th International Conference on Vehicle Technology and Intelligent Transport Systems
98