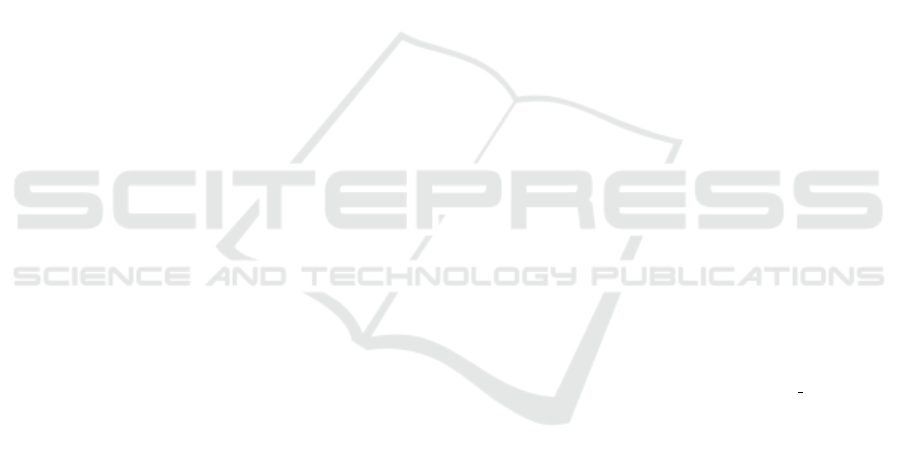
Clark, M. (03 July 2020 (accessed August 17, 2020)). The
Rise Of The Railway Drone.
CN (2019). Pest management plan for integrated vegetation
control, Canadian National Railway Company (CN)
pest management plan. 5-yearly plan, BC, Canada.
Duarte-Carvajalino, J., Alzate, D., Ramirez, A., Santa, J.,
Fajardo-Rojas, A., and Soto-Su
´
arez, M. (2018). Eval-
uating late blight severity in potato crops using un-
manned aerial vehicles and machine learning algo-
rithms. Remote Sensing, 10.
Graz University of Technology (2020). Semantic drone
dataset. http://dronedataset.icg.tugraz.at. Retrieved
2020-06-01.
Grigorev, A., Liu, S., Tian, Z., Xiong, J., Rho, S., and Feng,
J. (2020). Delving deeper in drone-based person re-
id by employing deep decision forest and attributes
fusion. ACM Trans. Multimedia Comput. Commun.
Appl., 16(1).
Huang, H., Deng, J., Lan, Y., Yang, A., Deng, X., and
Zhang, L. (2018). A fully convolutional network for
weed mapping of unmanned aerial vehicle (UAV) im-
agery. PLOS ONE.
Hulin, B. and Schussler, S. (2005). Measuring vegetation
along railway tracks. In Proc. 2005 IEEE Intelligent
Transportation Systems, pages 561–565.
K
¨
uchhold, M., Simon, M., Eiselein, V., and Sikora, T.
(2018). Scale-adaptive real-time crowd detection and
counting for drone images. In 2018 25th IEEE In-
ternational Conference on Image Processing (ICIP),
pages 943–947.
Lin, T., Doll
´
ar, P., Girshick, R., He, K., Hariharan, B., and
Belongie, S. (2017). Feature pyramid networks for
object detection. In CVPR.
Nyberg, R. (2016a). A machine learning approach for
recognising woody plants on railway trackbeds. In
Proc. of International Conference on Railway Engi-
neering (ICRE).
Nyberg, R., Gupta, N., Yella, S., and Dougherty, M. (2013).
Detecting plants on railway embankments. Journal of
Software Engineering and Applications, pages 8–12.
Nyberg, R. G. (2016b). Automating Condition Monitor-
ing of Vegetation on Railway Trackbeds and Embank-
ments. PhD thesis.
Perazzi, F., Kr
¨
ahenb
¨
uhl, P., Pritch, Y., and Hornung, A.
(2012). Saliency filters: Contrast based filtering for
salient region detection. In CVPR.
Quan, A., Herrmann, C., and Soliman, H. (2019). Project
vulture: A prototype for using drones in search and
rescue operations. In 2019 15th International Con-
ference on Distributed Computing in Sensor Systems
(DCOSS), pages 619–624.
Ronneberger, O., P.Fischer, and Brox, T. (2015). U-
Net: convolutional networks for biomedical image
segmentation. In Medical Image Computing and
Computer-Assisted Intervention (MICCAI), volume
9351 of LNCS, pages 234–241.
Russakovsky, O., Deng, J., Su, H., Krause, J., Satheesh,
S., Ma, S., Huang, Z., Karpathy, A., Khosla, A.,
Bernstein, M., Berg, A. C., and Fei-Fei, L. (2015).
ImageNet Large Scale Visual Recognition Challenge.
International Journal of Computer Vision (IJCV),
115(3):211–252.
Scott, J., Loveridge, F., and O’Brien, A. (2007). Influ-
ence of climate and vegetation on railway embank-
ments. In Geotechnical Engineering in Urban Envi-
ronments: Proceedings of the 14th European Confer-
ence on Soil Mechanics and Geotechnical Engineer-
ing, pages 659–664.
Seferbekov, S., Iglovikov, V., Buslaev, A., and Shvets, A.
(2018). Feature pyramid network for multi-class land
segmentation. In CVPR.
Shelhamer, E., Long, J., and Darrell, T. (2017). Fully con-
volutional networks for semantic segmentation. IEEE
Trans. Pattern Anal. Mach. Intell., 39(4):640–651.
Singh, A. K., Swarup, A., Agarwal, A., and Singh, D.
(2019). Vision based rail track extraction and mon-
itoring through drone imagery. ICT Express, 5(4):250
– 255.
Song, W., Li, S., Chang, T., Hao, A., Zhao, Q., and Qin,
H. (2020). Cross-view contextual relation transferred
network for unsupervised vehicle tracking in drone
videos. In WACV.
THALES (2019). How drones will change the fu-
ture of railways. https://www.thalesgroup.
com/en/worldwide/transport/magazine/
how-drones-will-change-future-railways. Retrieved
2020-08-17.
Wahab, I., Hall, O., and Jirstr
¨
om, M. (2018). Remote sens-
ing of yields: Application of UAV imagery-derived
NDVI for estimating maize vigor and yields in com-
plex farming systems in sub-saharan Africa. Drones,
2(3):28.
Wang, X., Girshick, R., Gupta, A., and He, K. (2018). Non-
local neural networks. In CVPR.
Xie, S., Girshick, R., Dollar, P., Tu, Z., and He, K. (2017).
Aggregated residual transformations for deep neural
networks. In CVPR.
Yakubovskiy, P. (2020). Segmentation models pytorch.
https://github.com/qubvel/segmentation
models.
pytorch.
Yella, S., Nyberg, R., Payvar, B., Dougherty, M., and Gupta,
N. (2013). Machine vision approach for automating
vegetation detection on railway tracks. Journal of In-
telligent Systems, pages 179–196.
VEHITS 2021 - 7th International Conference on Vehicle Technology and Intelligent Transport Systems
464