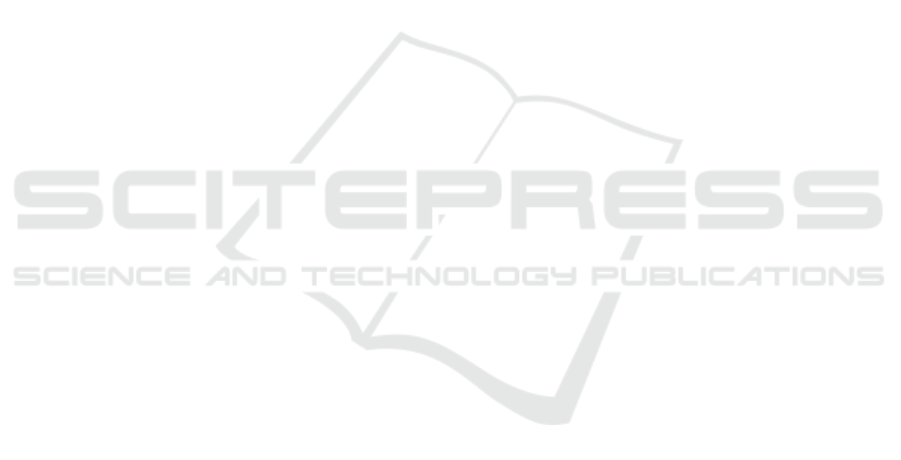
Ho, T. K. and Basu, M. (2002). Complexity measures of
supervised classification problems. IEEE Transac-
tions on Pattern Analysis and Machine Intelligence,
24(3):289–300.
Ishibuchi, H., Nakashima, T., and Nii, M. (2005). Clas-
sification and Modeling with Linguistic Information
Granules, Advanced Approaches to Linguistic Data
Mining. Advanced Information Processing. Springer,
Berlin.
Kohavi, R. (1995). A study of cross-validation and boot-
strap for accuracy estimation and model selection. In
Proceedings of the 14th International Joint Confer-
ence on Artificial Intelligence - Volume 2, IJCAI’95,
page 1137–1143, San Francisco, CA, USA. Morgan
Kaufmann PUBLISHERs Inc.
Kotsiantis, S. and Pintelas, P. (2004). A hybrid decision sup-
port tool. In Proceedings of 6th International Confer-
ence on Enterprise Information Systems, pages 448–
453, Porto, Portugal. Springer.
Kuncheva, L. I. and Whitaker, C. J. (2003). Measures
of diversity in classifier ensembles and their rela-
tionship with the ensemble accuracy. Mach. Learn.,
51(2):181—-207.
Lanes, M., Borges, E. N., and Galante, R. (2017a). The ef-
fects of classifiers diversity on the accuracy of stack-
ing. In SEKE, pages 323–328, New York, USA. ACM
Press.
Lanes, M., Schiavo, P. F., Pereira Jr, S. F., Borges, E. N.,
and Galante, R. (2017b). An analysis of the impact of
diversity on stacking supervised classifiers. In ICEIS
(1), pages 233–240, Set
´
ubal, Portugal. ScitePress.
Lee, E. S. (2017). Exploring the performance of stacking
classifier to predict depression among the elderly. In
2017 IEEE International Conference on Healthcare
Informatics (ICHI), pages 13–20, Park City, UT, USA.
IEEE.
Li, W. and Zou, L. (2017). Classifier stacking for native lan-
guage identification. In Proceedings of the 12th Work-
shop on Innovative Use of NLP for Building Educa-
tional Applications, pages 390–397, Park City, Utah,
USA. Association for Computational Linguistics.
Loh, W.-Y. (2011). Classification and regression trees.
Wiley Interdisciplinary Reviews: Data Mining and
Knowledge Discovery, 1(1):14–23.
Lucca, G., Sanz, J., Dimuro, G. P., Bedregal, B., and
Bustince, H. (2018). Analyzing the behavior of
aggregation and pre-aggregation functions in fuzzy
rule-based classification systems with data complex-
ity measures. In Kacprzyk, J., Szmidt, E., Zadro
˙
zny,
S., Atanassov, K. T., and Krawczak, M., editors, Ad-
vances in Fuzzy Logic and Technology 2017, pages
443–455, Cham. Springer International Publishing.
Makhtar, M., Yang, L., Neagu, D., and Ridley, M. (2012).
Optimisation of classifier ensemble for predictive tox-
icology applications. In 14th International Confer-
ence on Computer Modelling and Simulation, pages
236–241, Washington, USA. IEEE Computer Society.
Merz, C. J. (1999). Using correspondence analysis to com-
bine classifiers. Machine Learning, 36(1-2):33–58.
Michie, D., Spiegelhalter, D. J., Taylor, C. C., and Camp-
bell, J., editors (1994). Machine Learning, Neural and
Statistical Classification. Ellis Horwood, Upper Sad-
dle River, NJ, USA.
Muhammad, A. T. and Jim, S. (2010). Creating di-
verse nearest-neighbour ensembles using simultane-
ous metaheuristic feature selection. Pattern Recog-
nition Letters, 31(11):1470–1480.
Nelder, J. A. and Wedderburn, R. W. (1972). Generalized
linear models. Journal of the Royal Statistical Society:
Series A (General), 135(3):370–384.
Opitz, D. and Maclin, R. (1999). Popular ensemble meth-
ods: An empirical study. Journal of Artificial Intelli-
gence Research, 11:169–198.
Quinlan, J. (1986). Induction of decision trees. Machine
Learning, 1:81–106.
Shipp, C. A. and Kuncheva, L. I. (2002). Relationships
between combination methods and measures of di-
versity in combining classifiers. Information Fusion,
3(2):135 – 148.
Skalak, D. B. et al. (1996). The sources of increased
accuracy for two proposed boosting algorithms. In
Proc. American Association for Artificial Intelligence,
AAAI-96, Integrating Multiple Learned Models Work-
shop, volume 1129, page 1133, Menlo Park, CA,
USA. Citeseer, AAAI Press.
Steinwart, I. and Christmann, A. (2008). Support Vector
Machines. Springer Publishing Company, Incorpo-
rated, New York, USA, 1st edition.
Ting, K. M. and Witten, I. H. (1999). Issues in stacked
generalization. Journal of Artificial Intelligence Re-
search, 10:271–289.
Wang, S. and Yao, X. (2009). Diversity analysis on imbal-
anced data sets by using ensemble models. In 2009
IEEE Symposium on Computational Intelligence and
Data Mining, pages 324–331, New York, USA. IEEE,
IEEE.
Whalen, S. and Pandey, G. (2013). A comparative analy-
sis of ensemble classifiers: Case studies in genomics.
In 2013 IEEE 13th International Conference on Data
Mining, pages 807–816, Washington, USA. IEEE
Computer Society.
Wolpert, D. H. (1992). Stacked generalization. Neural net-
works, 5(2):241–259.
Wolpert, D. H. (1996). The lack of a priori distinctions
between learning algorithms. Neural computation,
8(7):1341–1390.
Zhang, H. (2005). Exploring conditions for the optimal-
ity of naive bayes. International Journal of Pattern
Recognition and Artificial Intelligence, 19(02):183–
198.
Exploring the Relationships between Data Complexity and Classification Diversity in Ensembles
659