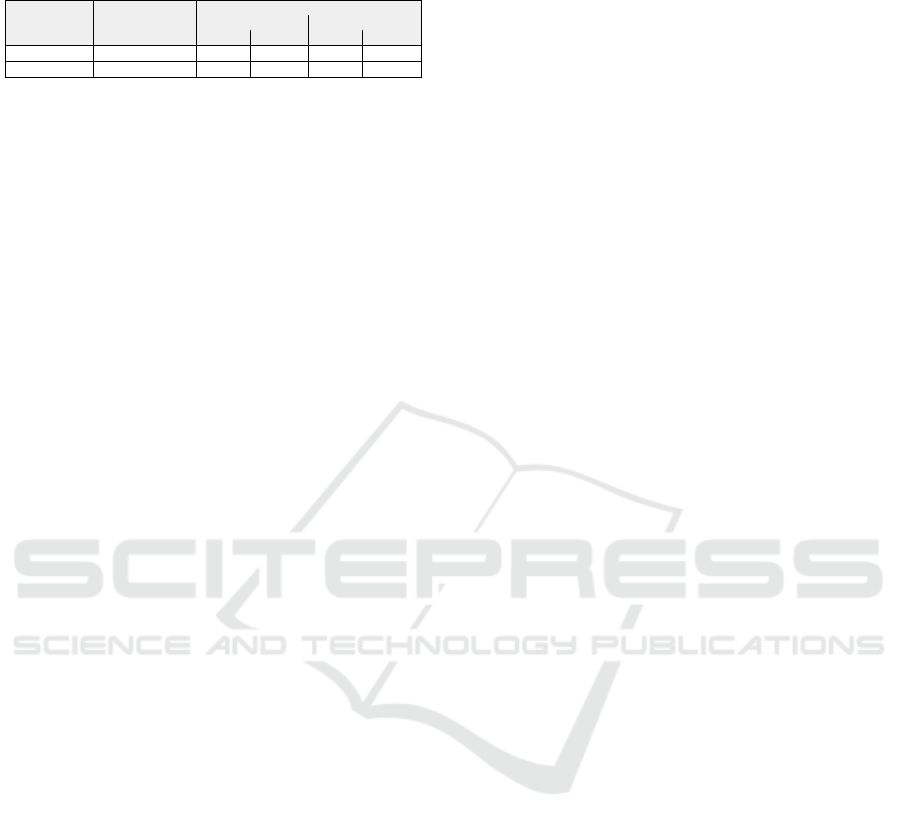
Table 6: Results obtained with the metrics M
FGW
and M
GW
,
considering the experiment configurations E1 and E2.
Proposed Method
E1 E2
Controlled
Vocabulary
Bretcheneider
(Naive)
M
GW
M
FGW
M
GW
M
FGW
O
1
0,255 0,478 0,455 0,660 0,620
O
2
0,275 0,430 0,430 0,655 0,595
use of a controlled vocabulary over a crime type do-
main may be a way towards the identification of suspi-
cious persons. It is important to highlight that despite
the AUC values presented by INSPECTION are not
comparable to the ones produced by machine learn-
ing methods, the proposed method does not demand
labeled data as these methods do.
7 CONCLUSIONS
One of the main concerns in the analysis of social net-
works is to identify suspicious people. It is a hard
task to find out who makes use of these networks to
practice crimes or spread risk to other people. There
are already several machine learning based methods
to identify suspicious people in social networks. Al-
though most of them have shown promising results,
they demand previously labeled data (indicating who
are the suspects) to build their classification models.
Such demand hampers their use in real applications
because labeled data in the context of virtual crimes
are usually rare and difficult to obtain. Given this
scenario, the present work proposed INSPECTION, a
method that uses a controlled vocabulary, specifically
built according to the crime type in focus, to identify
suspicious people in the social network, without the
need of previously labeled data sets.
To evaluate the proposed method, this work re-
ports on experiments for the pedophilia criminal sce-
nario. To perform these experiments, a prototype was
implemented. Also, a specific controlled vocabulary
was built (in Portuguese), based on other existing vo-
cabularies. The results show that the INSPECTION
method is a promising approach to identify suspi-
cious people without depending on a previously la-
beled data.
Future works include evaluating the performance
of the proposed method applied to other social net-
works, and other crime types. In addition, an on-
going work is the extension of the proposed method
to include social network topological analysis. Such
analysis may lead to improvements on the INSPEC-
TION’s performance. Moreover, the inclusion of a
new stage in the INSPECTION process to enrich the
controlled vocabulary is foreseen, as well as, to con-
sider semantic information while weighting the con-
trolled vocabulary.
ACKNOWLEDGEMENTS
This study was partially funded by the Cybernetic
Research Subproject of the Brazilian Army Strategic
Project. In addition, the authors would like to thank
Dr. Paulo Renato da Costa Pereira, a specialist in pe-
dophilia crimes of the Brazilian Federal Police, for his
valuable support throughout the experiments.
REFERENCES
Andrijauskas, A., Shimabukuro, A., and Maia, R. F. (2017).
Desenvolvimento de base de dados em língua por-
tuguesa sobre crimes sexuais (in Portuguese). VII
Simpósio de Iniciação Científica, Didática e Ações
Sociais da FEI.
Berry Michael, W. (2004). Automatic discovery of similar
words. Survey of Text Mining: Clustering, Classifica-
tion and Retrieval”, Springer Verlag, New York, LLC,
pages 24–43.
Bretschneider, U. and Peters, R. (2016). Detecting cyber-
bullying in online communities. European Confer-
ence on Information Systems.
Bretschneider, U., Wöhner, T., and Peters, R. (2014). De-
tecting online harassment in social networks. Inter-
national Conference on Information Systems.
Chandrasekaran, B., Josephson, J. R., and Benjamins, V. R.
(1999). What are ontologies, and why do we need
them? IEEE Intelligent Systems and their applica-
tions, 14(1):20–26.
Dong, Y., Tang, J., Wu, S., Tian, J., Chawla, N. V., Rao, J.,
and Cao, H. (2012). Link prediction and recommen-
dation across heterogeneous social networks. In 2012
IEEE 12th International conference on data mining,
pages 181–190. IEEE.
Dorogovtsev, S. N. and Mendes, J. F. (2002). Evolution of
networks. Advances in physics, 51(4):1079–1187.
Elzinga, P., Wolff, K. E., and Poelmans, J. (2012). Ana-
lyzing chat conversations of pedophiles with temporal
relational semantic systems. In Europ. Intel. and Se-
curity Informatics Conf., 2012, pages 242–249. IEEE.
Figueiredo, D. R. (2011). Introdução a redes complexas (in
Portuguese), pages 303–358. Sociedade Brasileira de
Computação, Rio de Janeiro.
Fire, M., Katz, G., and Elovici, Y. (2012). Strangers intru-
sion detection-detecting spammers and fake profiles in
social networks based on topology anomalies. Human
Journal, pages 26–39.
Hobbs, J. R. and Pan, F. (2006). Time ontology in owl.
W3C working draft, 27:133.
Kronk, C., Tran, G. Q., and Wu, D. T. (2019). Creating a
queer ontology: The gender, sex, and sexual orienta-
tion (gsso) ontology. Studies in health technology and
informatics, 264:208–212.
Kuang, Z., Yu, J., Li, Z., Zhang, B., and Fan, J. (2018). In-
tegrating multi-level deep learning and concept ontol-
ogy for large-scale visual recognition. Pattern Recog-
nition, 78:198–214.
Identifying Suspects on Social Networks: An Approach based on Non-structured and Non-labeled Data
61