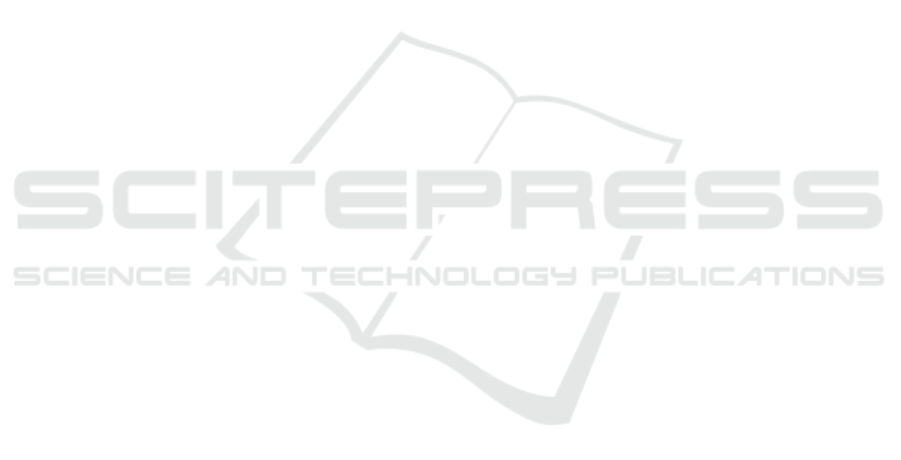
Applications and Techniques in Information Security,
pages 181–189, Singapore. Springer Singapore.
Antonakakis, M., April, T., Bailey, M., Bernhard, M.,
Bursztein, E., Cochran, J., Durumeric, Z., Halderman,
J. A., Invernizzi, L., Kallitsis, M., Kumar, D., Lever,
C., Ma, Z., Mason, J., Menscher, D., Seaman, C., Sul-
livan, N., Thomas, K., and Zhou, Y. (2017). Under-
standing the Mirai botnet. In 26th USENIX Security
Symposium (USENIX Security 17), pages 1093–1110,
Vancouver, BC. USENIX Association.
Aslan, O. A. and Samet, R. (2020). A comprehensive re-
view on malware detection approaches. IEEE Access,
8:6249–6271.
Check Point Software Technologies Ltd. (2020). Cy-
ber security report. https://www.ntsc.org/assets/
pdfs/cyber-security-report-2020.pdf, Last accessed:
26.10.2020.
Cozzi, E., Vervier, P.-A., Dell’Amico, M., Shen, Y., Bigle,
L., and Balzarotti, D. (2020). The tangled genealogy
of IoT malware. In Annual Computer Security Appli-
cations Conference (ACSAC2020), Austin, USA. At
the time of writing (Nov 18, 2020), the paper has been
accepted to the conference but not yet published. The
authors made the paper available to the public.
Ding, F., Li, H., Luo, F., Hu, H., Cheng, L., Xiao, H.,
and Ge, R. (2020). DeepPower: Non-intrusive and
deep learning-based detection of IoT malware using
power side channels. In Proceedings of the 15th ACM
Asia Conference on Computer and Communications
Security, ASIA CCS ’20, page 33–46, New York, NY,
USA. Association for Computing Machinery.
Dovom, E. M., Azmoodeh, A., Dehghantanha, A., Newton,
D. E., Parizi, R. M., and Karimipour, H. (2019). Fuzzy
pattern tree for edge malware detection and catego-
rization in IoT. Journal of Systems Architecture, 97:1
– 7.
Gibert, D., Mateu, C., and Planes, J. (2020). The rise of
machine learning for detection and classification of
malware: Research developments, trends and chal-
lenges. Journal of Network and Computer Applica-
tions, 153:102526.
Goyal, M., Sahoo, I., and Geethakumari, G. (2019). Http
botnet detection in IoT devices using network traffic
analysis. In 2019 International Conference on Recent
Advances in Energy-efficient Computing and Commu-
nication (ICRAECC), pages 1–6.
HaddadPajouh, H., Dehghantanha, A., Khayami, R., and
Choo, K.-K. R. (2018). A deep recurrent neural net-
work based approach for Internet of Things malware
threat hunting. Future Generation Computer Systems,
85:88 – 96.
Meidan, Y., Bohadana, M., Mathov, Y., Mirsky, Y., Shab-
tai, A., Breitenbacher, D., and Elovici, Y. (2018). N-
BaIoT — network-based detection of IoT botnet at-
tacks using deep autoencoders. IEEE Pervasive Com-
puting, 17(3):12–22.
Oliver, J., Cheng, C., and Chen, Y. (2013). TLSH – A Lo-
cality Sensitive Hash. In 2013 Fourth Cybercrime and
Trustworthy Computing Workshop, pages 7–13, Syd-
ney NSW, Australia. IEEE.
Shobana, M. and Poonkuzhali, S. (2020). A novel ap-
proach to detect IoT malware by system calls using
deep learning techniques. In 2020 International Con-
ference on Innovative Trends in Information Technol-
ogy (ICITIIT), pages 1–5.
Soliman, S. W., Sobh, M. A., and Bahaa-Eldin, A. M.
(2017). Taxonomy of malware analysis in the IoT.
In 2017 12th International Conference on Computer
Engineering and Systems (ICCES), pages 519–529.
Sophos Ltd. (2019). Sophoslabs 2019 Threat Report.
https://www.sophos.com/en-us/medialibrary/pdfs/
technical-papers/sophoslabs-2019-threat-report.pdf,
Last accessed: 26.10.2020.
Su, J., Vasconcellos, D. V., Prasad, S., Sgandurra, D., Feng,
Y., and Sakurai, K. (2018). Lightweight classifica-
tion of IoT malware based on image recognition. In
2018 IEEE 42nd Annual Computer Software and Ap-
plications Conference (COMPSAC), volume 02, pages
664–669.
Sun, H., Wang, X., Buyya, R., and Su, J. (2017). Cloudeyes:
Cloud-based malware detection with reversible sketch
for resource-constrained internet of things (IoT) de-
vices. Software: Practice and Experience, 47(3):421–
441.
Takase, H., Kobayashi, R., Kato, M., and Ohmura, R.
(2020). A prototype implementation and evaluation
of the malware detection mechanism for IoT devices
using the processor information. International Jour-
nal of Information Security, 19.
Ucci, D., Aniello, L., and Baldoni, R. (2019). Survey of ma-
chine learning techniques for malware analysis. Com-
puters & Security, 81:123 – 147.
Van der Elzen, I. and van Heugten, J. (2017). Techniques for
detecting compromised IoT devices. Technical report,
University of Amsterdam.
Ye, Y., Li, T., Adjeroh, D., and Iyengar, S. S. (2017). A
survey on malware detection using data mining tech-
niques. ACM Comput. Surv., 50(3).
IoTBDS 2021 - 6th International Conference on Internet of Things, Big Data and Security
68