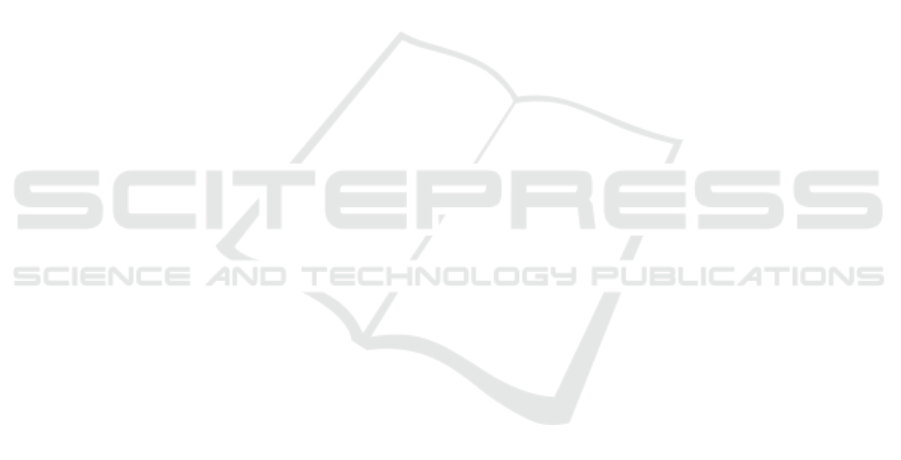
taining 9745 images from 153 species. We also gen-
erated random synthetic damage on the leaf masks
to predict the original shape and calculate the dam-
aged area. Even with more leaves from more species,
the algorithm maintained the RMSE factor in 1.76 (±
3.02), indicating the proposed solution’s generalism.
Also, in this case, the dice coefficient average indi-
cates that the shape reconstruction was accurate.
ACKNOWLEDGMENT
The authors would like to thank CAPES, CNPq,
FAPEMIG and the Federal University of Ouro Preto
for supporting this work. This study was financed in
part by the Coordenac¸
˜
ao de Aperfeic¸oamento de Pes-
soal de N
´
ıvel Superior - Brasil (CAPES) - Finance
Code 001.
REFERENCES
Al-Saddik, H., Laybros, A., Billiot, B., and Cointault, F.
(2018). Using image texture and spectral reflectance
analysis to detect yellowness and esca in grapevines at
leaf-level. Remote Sensing, 10(4):618.
Antholzer, S., Haltmeier, M., Nuster, R., and Schwab, J.
(2018). Photoacoustic image reconstruction via deep
learning. In Photons Plus Ultrasound: Imaging and
Sensing 2018, volume 10494, page 104944U. Interna-
tional Society for Optics and Photonics.
Bangare, S. L., Dubal, A., Bangare, P. S., and Patil, S.
(2015). Reviewing otsu’s method for image thresh-
olding. International Journal of Applied Engineering
Research, 10(9):21777–21783.
Baudron, F., Zaman-Allah, M. A., Chaipa, I., Chari, N.,
and Chinwada, P. (2019). Understanding the factors
influencing fall armyworm (spodoptera frugiperda je
smith) damage in african smallholder maize fields and
quantifying its impact on yield. a case study in eastern
zimbabwe. Crop Protection, 120:141–150.
Bauer, J., Jarmer, T., Schittenhelm, S., Siegmann, B., and
Aschenbruck, N. (2019). Processing and filtering of
leaf area index time series assessed by in-situ wireless
sensor networks. Computers and Electronics in Agri-
culture, 165:104867.
Ben
´
ıtez-Malvido, J., L
´
azaro, A., and Ferraz, I. D. (2018).
Effect of distance to edge and edge interaction on
seedling regeneration and biotic damage in tropical
rainforest fragments: A long-term experiment. Jour-
nal of Ecology, 106(6):2204–2217.
C
´
ardenas, R. E., H
¨
attenschwiler, S., Valencia, R., Argoti,
A., and Dangles, O. (2015). Plant herbivory responses
through changes in leaf quality have no effect on sub-
sequent leaf-litter decomposition in a neotropical rain
forest tree community. New Phytologist, 207(3):817–
829.
Clement, A., Verfaille, T., Lormel, C., and Jaloux, B.
(2015). A new colour vision system to quantify
automatically foliar discolouration caused by insect
pests feeding on leaf cells. Biosystems Engineering,
133:128–140.
da Silva, L. A., Bressan, P. O., Gonc¸alves, D. N., Freitas,
D. M., Machado, B. B., and Gonc¸alves, W. N. (2019).
Estimating soybean leaf defoliation using convolu-
tional neural networks and synthetic images. Com-
puters and electronics in agriculture, 156:360–368.
Delabrida, S., Billinghurst, M., Thomas, B. H., Rabelo,
R. A., and Ribeiro, S. P. (2017). Design of a wear-
able system for 3d data acquisition and reconstruction
for tree climbers. In SIGGRAPH Asia 2017 Mobile
Graphics & Interactive Applications, page 26. ACM.
Delabrida, S., D’Angelo, T., Oliveira, R. A., and Loureiro,
A. A. (2016a). Building wearables for geology: An
operating system approach. ACM SIGOPS Operating
Systems Review, 50(1):31–45.
Delabrida, S., D’Angelo, T., Oliveira, R. A. R., and
Loureiro, A. A. F. (2016b). Wearable hud for eco-
logical field research applications. Mobile Networks
and Applications, 21(4):677–687.
Delgado, J. A., Kowalski, K., and Tebbe, C. (2013). The
first nitrogen index app for mobile devices: Using
portable technology for smart agricultural manage-
ment. Computers and electronics in agriculture,
91:121–123.
Dong, H., Yang, G., Liu, F., Mo, Y., and Guo, Y. (2017).
Automatic brain tumor detection and segmentation us-
ing u-net based fully convolutional networks. In an-
nual conference on medical image understanding and
analysis, pages 506–517. Springer.
Genc¸Tav, A., Aksoy, S., and
¨
ONder, S. (2012). Unsuper-
vised segmentation and classification of cervical cell
images. Pattern recognition, 45(12):4151–4168.
Gunnarsson, B., Wallin, J., and Klingberg, J. (2018). Pre-
dation by avian insectivores on caterpillars is linked
to leaf damage on oak (quercus robur). Oecologia,
188(3):733–741.
Hou, X., Shen, L., Sun, K., and Qiu, G. (2017). Deep fea-
ture consistent variational autoencoder. In 2017 IEEE
Winter Conference on Applications of Computer Vi-
sion (WACV), pages 1133–1141. IEEE.
Hyun, C. M., Kim, H. P., Lee, S. M., Lee, S., and
Seo, J. K. (2018). Deep learning for undersampled
mri reconstruction. Physics in Medicine & Biology,
63(13):135007.
Isola, P., Zhu, J.-Y., Zhou, T., and Efros, A. A. (2017).
Image-to-image translation with conditional adversar-
ial networks. In Proceedings of the IEEE conference
on computer vision and pattern recognition, pages
1125–1134.
Kozlov, M. V., Lanta, V., Zverev, V., and Zvereva, E. L.
(2015). Background losses of woody plant foliage to
insects show variable relationships with plant func-
tional traits across the globe. Journal of Ecology,
103(6):1519–1528.
Leite, M. L. d. M. V., Lucena, L. R. R. d., Cruz, M. G. d.,
S
´
a J
´
unior, E. H. d., and Sim
˜
oes, V. J. L. P. (2019).
Leaf area estimate of pennisetum glaucum by linear
dimensions. Acta Scientiarum. Animal Sciences, 41.
ICEIS 2021 - 23rd International Conference on Enterprise Information Systems
494