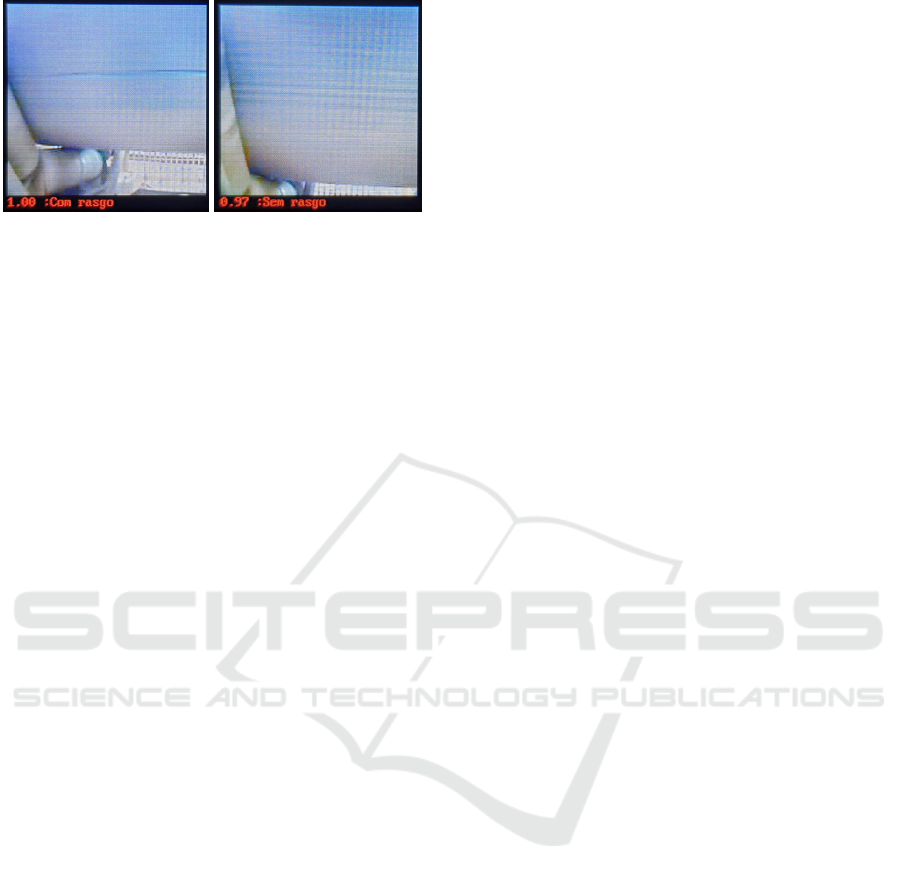
(a) Tear detected. (b) Normal condition.
Figure 20: Images of SiPEED prototype in operation.
Collaboratory and executed by SiPEED’s KPU.
The results of precision (100%), recall (93%) and
total overall accuracy (96%) obtained during the 9
field tests performed were satisfactory and indicate
the feasibility of using edge AI with the MobileNet
deep learning model for the detection longitudinal rip
on belt. With these positive results we understand that
other failure modes, with distinct visual characteris-
tics such as misalignment, contamination of the belt
return and seam failures can be investigated.
As the objectives of the work were achieved, the
process of building 5 more prototypes for definitive
installation on 2 conveyor belts and continuous moni-
toring of their performance was initiated, considering
the normal operational conditions of the iron ore ben-
eficiation plant environment.
Continuing the development of the belt failure de-
tection system, new functionalities will be developed,
such as automatic verification of the cleaning condi-
tion of the optical system lens, detection of the correct
positioning of the sensor and detection of failures in
the lighting conditions. These improvements are nec-
essary to guarantee the reliability of the solution in
the operational conditions verified in the area.
ACKNOWLEDGMENT
This study was financed in part by the Coordenac¸
˜
ao
de Aperfeic¸oamento de Pessoal de N
´
ıvel Superior
- Brasil (CAPES) - Finance Code 001, the Con-
selho Nacional de Desenvolvimento Cient
´
ıfico e Tec-
nol
´
ogico (CNPQ), the Instituto Tecnol
´
ogico Vale
(ITV) and the Universidade Federal de Ouro Preto
(UFOP).
REFERENCES
Bertino, E. and Banerjee, S. (2020). Artificial intelligence
at the edge. arXiv preprint arXiv:2012.05410.
Corporation, I. (2020). Openvino toolkit.
Deng, L., Li, G., Han, S., Shi, L., and Xie, Y. (2020a).
Model compression and hardware acceleration for
neural networks: A comprehensive survey. Proceed-
ings of the IEEE, 108(4):485–532.
Deng, S., Zhao, H., Fang, W., Yin, J., Dustdar, S., and
Zomaya, A. Y. (2020b). Edge intelligence: the con-
fluence of edge computing and artificial intelligence.
IEEE Internet of Things Journal.
Gruenhagen, J. H. and Parker, R. (2020). Factors driving
or impeding the diffusion and adoption of innovation
in mining: A systematic review of the literature. Re-
sources Policy, 65:101540.
Hardyg
´
ora, M., Wachowicz, J., Czaplicka-Kolarz, K.,
and Markusik, S. (1999). Conveyor belts. WNT
Warszawa.
Hou, C., Qiao, T., Qiao, M., Xiong, X., Yang, Y., and
Zhang, H. (2019). Research on audio-visual detec-
tion method for conveyor belt longitudinal tear. IEEE
Access, 7:120202–120213.
Howard, A. G., Zhu, M., Chen, B., Kalenichenko, D.,
Wang, W., Weyand, T., Andreetto, M., and Adam,
H. (2017). Mobilenets: Efficient convolutional neu-
ral networks for mobile vision applications. arXiv
preprint arXiv:1704.04861.
Jurdziak, L., Blazej, R., and Bajda, M. (2018). Conveyor
belt 4.0. In International Conference on Intelligent
Systems in Production Engineering and Maintenance,
pages 645–654. Springer.
Koul, A., Ganju, S., and Kasan, M. (2020). Pratical deep
learning for cloud, mobile, and edge: Real world ai &
computer vision projects using pyhton, keras & ten-
sorflow.
Krizhevsky, A., Sutskever, I., and Hinton, G. E. (2017). Im-
agenet classification with deep convolutional neural
networks. Communications of the ACM, 60(6):84–90.
LLC, G. (2020). Coral ai.
Majidifard, H., Jin, P., Adu-Gyamfi, Y., and Buttlar, W. G.
(2020). Pavement image datasets: A new benchmark
dataset to classify and densify pavement distresses.
Transportation Research Record, 2674(2):328–339.
Maslov, D. (2020). Image recognition with k210 boards and
arduino ide/micropython.
Raghu, M. and Schmidt, E. (2020). A survey of deep
learning for scientific discovery. arXiv preprint
arXiv:2003.11755.
Santos, A. A., Rocha, F. A. S., Azp
´
urua, H., Reis, A. J. R.,
and G., G. F. (2019). Automatic system for visual
inspection of belt conveyors. Intelligent Automation
Symposium, pages 1192–1197.
Sunnycase (2020). Kendrite nncase.
Xianguo, L., Lifang, S., Zixu, M., Can, Z., and Hangqi, J.
(2018). Laser-based on-line machine vision detection
for longitudinal rip of conveyor belt. Optik, 168:360–
369.
Embedded Edge Artificial Intelligence for Longitudinal Rip Detection in Conveyor Belt Applied at the Industrial Mining Environment
505