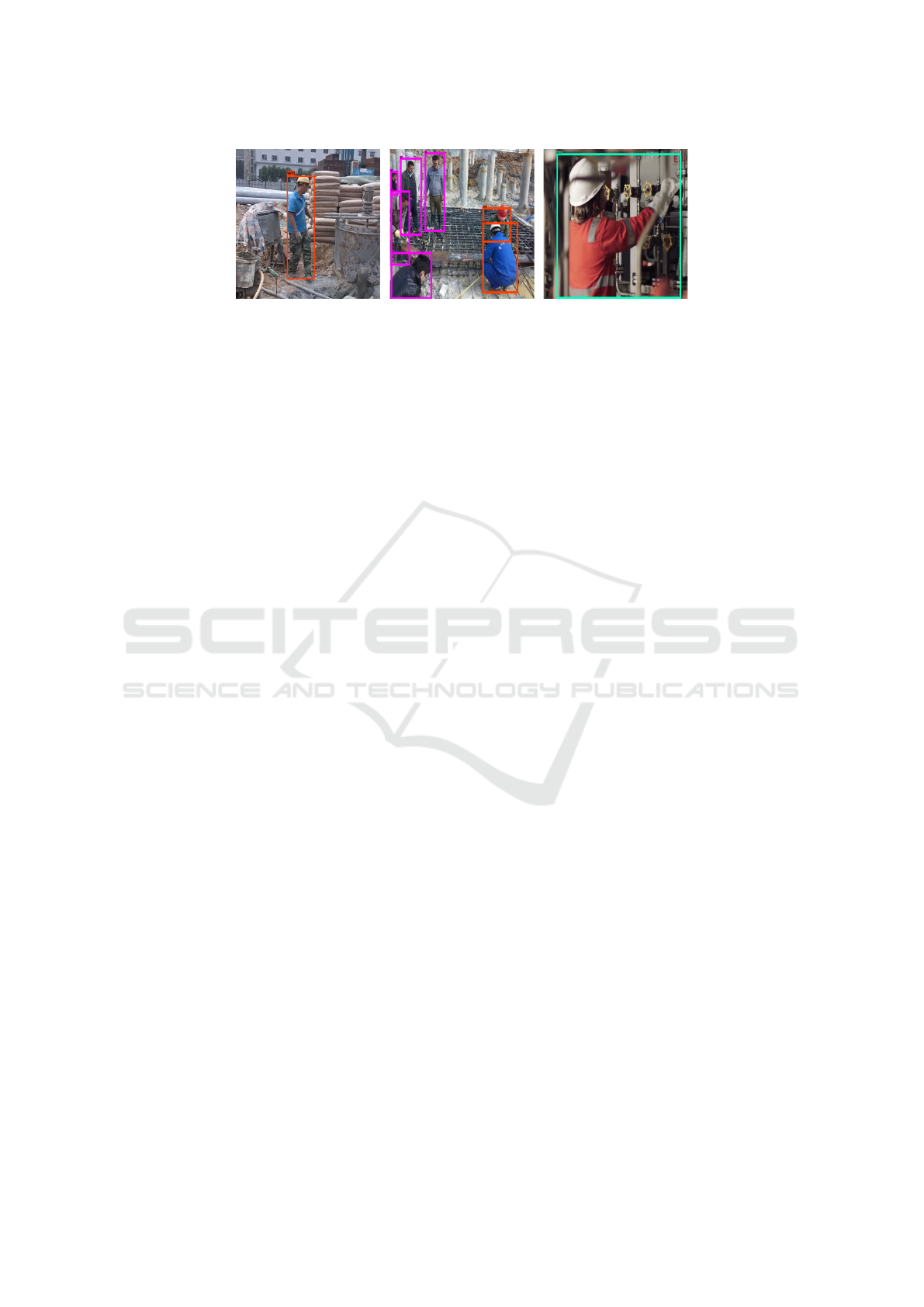
Figure 10: Example of detections obtained from YOLO-v4-AP1 model. The first two images are from the Crowd-sourced
dataset. The third image is from the Web-scrapped dataset that is in an industrial setting.
which employs an ensemble of classifiers VGG16,
ResNet50, and ResNet101, producing a final mAP of
72.87%. These results may indicate that superior re-
sults may be obtained from the individual improve-
ment of the classifiers or methods proposed in this
work. The ensemble method achieved an increase
of up to 2.45% compared to the best single classi-
fier (ResNet50) mAP (70.42%) of Approach II. Re-
garding the processing time, Approach I proved to be
more effective because of its one-stage implementa-
tion, which avoids bottlenecks between the process-
ing phases. Although slower, our results demonstrate
that Approach II still feasible to use it in real-time,
even with the use of an ensemble of classifiers.
From the implementation carried out for approach
I, it is possible to build a monitoring system that has
a robust detection and verification component. Since
the approach proved to be more efficient, not only in
terms of mAP (80.19%) but also in processing time,
reaching up to 11x faster (80 FPS) when compared
to approach II. Considering that, we believe that the
one-stage approach has a high potential for the con-
struction of an effective monitoring system that can
contribute to the safety of workers, minimizing the
number of accidents and live losses.
Regarding ID association component mentioned
in Figure 1, we believe that tracking algorithms such
as DeepSORT (Wojke et al., 2017) may present goods
results when employed along with the component ex-
plored in this work. This happens due to those al-
gorithms working well with robust detection models
to track real-time custom objects and assign unique
identities for each object.
REFERENCES
Bo, Y., Huan, Q., Huan, X., Rong, Z., Hongbin, L., Kebin,
M., Weizhong, Z., and Lei, Z. (2019). Helmet de-
tection under the power construction scene based on
image analysis. In 2019 IEEE 7th International Conf.
on Computer Science and Network Technology (ICC-
SNT), pages 67–71. IEEE.
Fang, Q., Li, H., Luo, X., Ding, L., Luo, H., Rose, T. M.,
and An, W. (2018). Detecting non-hardhat-use by
a deep learning method from far-field surveillance
videos. Automation in Construction, 85:1–9.
He, K., Zhang, X., Ren, S., and Sun, J. (2016). Deep resid-
ual learning for image recognition. In Proceedings of
the IEEE conference on computer vision and pattern
recognition, pages 770–778.
Krizhevsky, A., Sutskever, I., and Hinton, G. E. (2012). Im-
agenet classification with deep convolutional neural
networks. In Advances in neural information process-
ing systems, pages 1097–1105.
Li, J., Liu, H., Wang, T., Jiang, M., Wang, S., Li, K., and
Zhao, X. (2017). Safety helmet wearing detection
based on image processing and machine learning. In
2017 9th International Conf. on Advanced Computa-
tional Intelligence (ICACI), pages 201–205. IEEE.
Nath, N. D., Behzadan, A. H., and Paal, S. G. (2020). Deep
learning for site safety: Real-time detection of per-
sonal protective equipment. Automation in Construc-
tion, 112:103085.
Redmon, J., Divvala, S., Girshick, R., and Farhadi, A.
(2016). You only look once: Unified, real-time object
detection. In Proceedings of the IEEE conf. has on
computer vision and pattern recognition, pages 779–
788.
Ren, S., He, K., Girshick, R., and Sun, J. (2015). Faster
r-cnn: Towards real-time object detection with region
proposal networks. In Advances in neural information
processing systems, pages 91–99.
Simonyan, K. and Zisserman, A. (2014). Very deep con-
volutional networks for large-scale image recognition.
arXiv preprint arXiv:1409.1556.
Szegedy, C., Liu, W., Jia, Y., Sermanet, P., Reed, S.,
Anguelov, D., Erhan, D., Vanhoucke, V., and Rabi-
novich, A. (2015). Going deeper with convolutions.
In Proceedings of the IEEE conference on computer
vision and pattern recognition, pages 1–9.
Wojke, N., Bewley, A., and Paulus, D. (2017). Simple on-
line and realtime tracking with a deep association met-
ric. In 2017 IEEE international conference on image
processing (ICIP), pages 3645–3649. IEEE.
Wu, J., Cai, N., Chen, W., Wang, H., and Wang, G. (2019).
Automatic detection of hardhats worn by construction
personnel: A deep learning approach and benchmark
dataset. Automation in Construction, 106:102894.
Zheng, X., Yao, J., and Xu, X. (2019). Violation monitoring
system for power construction site. In IOP Conf. Se-
ries: Earth and Environmental Science, volume 234,
page 012062. IOP Publishing.
ICEIS 2021 - 23rd International Conference on Enterprise Information Systems
700